Pansharpening With Spatial Hessian Non-Convex Sparse and Spectral Gradient Low Rank Priors.
IEEE Trans. Image Process.(2023)
摘要
To get the high resolution multi-spectral (HRMS) images by the fusion of low resolution multi-spectral (LRMS) and panchromatic (PAN) images, an effectively pansharpening model with spatial Hessian non-convex sparse and spectral gradient low rank priors (PSHNSSGLR) is proposed in this paper. In particularly, from the statistical aspect of view, the spatial Hessian hyper-Laplacian non-convex sparse prior is developed to model the spatial Hessian consistency between HRMS and PAN. More importantly, it is recently the first work for pansharpening modeling with the spatial Hessian hyper-Laplacian non-convex sparse prior. Meanwhile, the spectral gradient low rank prior on HRMS is further developed for spectral feature preservation. Then, the alternating direction method of multipliers (ADMM) approach is applied for optimizing the proposed PSHNSSGLR model. Afterwards, many fusion experiments demonstrate the capability and superiority of PSHNSSGLR.
更多查看译文
关键词
Pansharpening, Laplace equations, Degradation, Spatial resolution, Satellites, Image fusion, Analytical models, spatial Hessian, hyper-Laplacian non-convex sparse prior, spectral gradient low rank
AI 理解论文
溯源树
样例
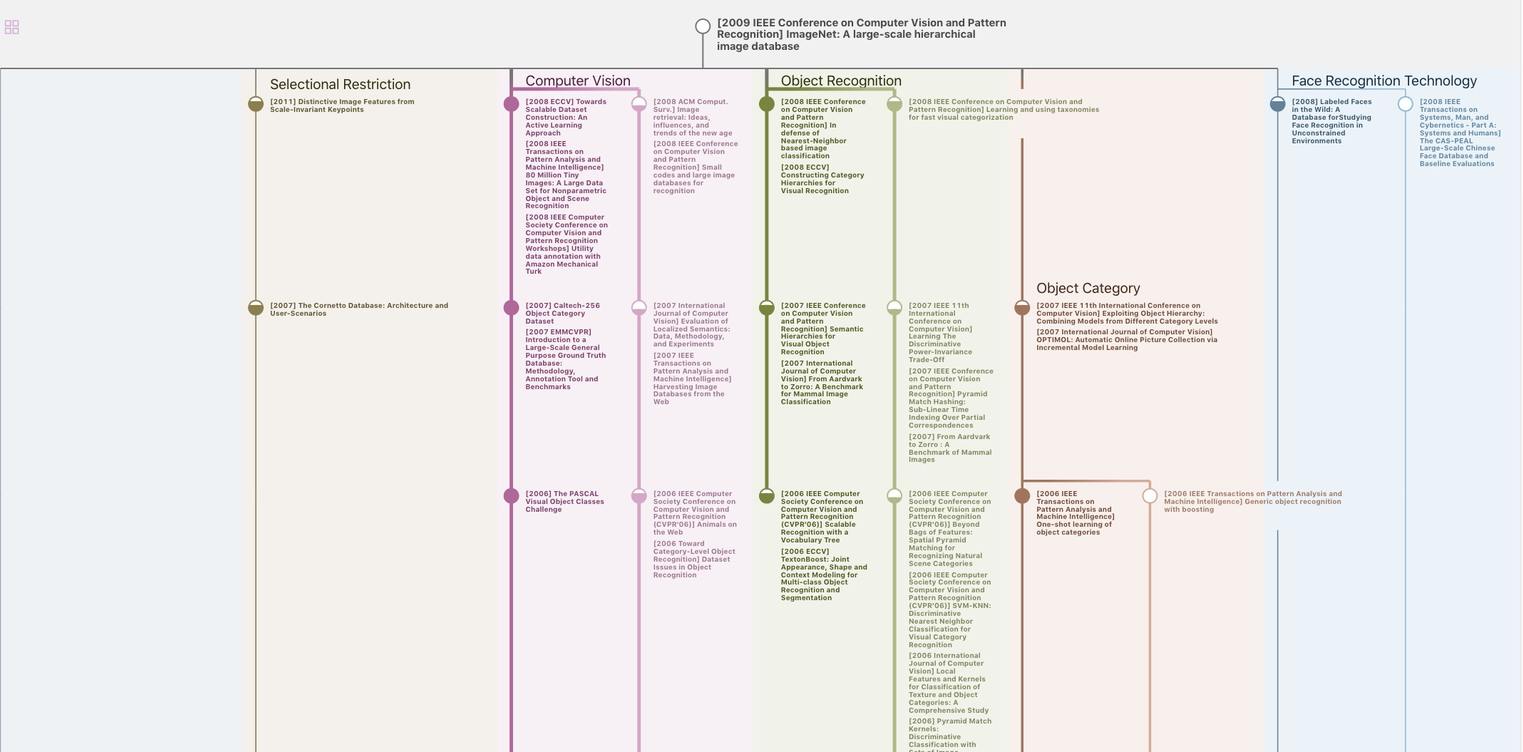
生成溯源树,研究论文发展脉络
Chat Paper
正在生成论文摘要