Optimisation of Spectral Wavelets for Persistence-based Graph Classification.
CoRR(2021)
摘要
A graph's spectral wavelet signature determines a filtration, and consequently an associated set of extended persistence diagrams. We propose a framework that optimises the choice of wavelet for a dataset of graphs, such that their associated persistence diagrams capture features of the graphs that are best suited to a given data science problem. Since the spectral wavelet signature of a graph is derived from its Laplacian, our framework encodes geometric properties of graphs in their associated persistence diagrams and can be applied to graphs without a priori node attributes. We apply our framework to graph classification problems and obtain performances competitive with other persistence-based architectures. To provide the underlying theoretical foundations, we extend the differentiability result for ordinary persistent homology to extended persistent homology.
更多查看译文
关键词
spectral wavelets,graph
AI 理解论文
溯源树
样例
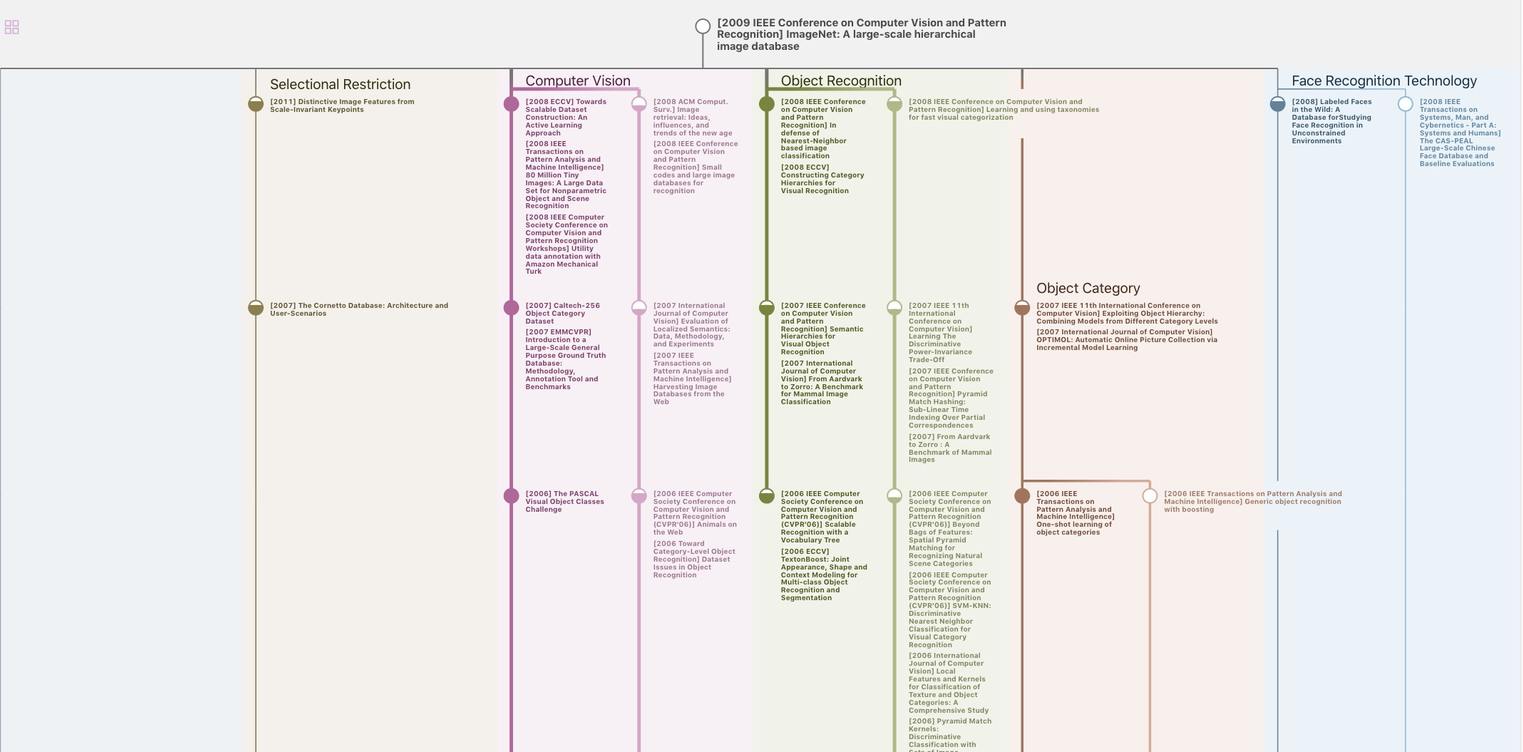
生成溯源树,研究论文发展脉络
Chat Paper
正在生成论文摘要