Multi-teacher Knowledge Distillation for Knowledge Graph Completion.
CoRR(2020)
摘要
Link prediction based on knowledge graph embedding (KGE) aims to predict new triples to complete knowledge graphs (KGs) automatically. However, recent KGE models tend to improve performance by excessively increasing vector dimensions, which would cause enormous training costs and save storage in practical applications. To address this problem, we first theoretically analyze the capacity of low-dimensional space for KG embeddings based on the principle of minimum entropy. Then, we propose a novel knowledge distillation framework for knowledge graph embedding, utilizing multiple low-dimensional KGE models as teachers. Under a novel iterative distillation strategy, the MulDE model produces soft labels according to training epochs and student performance adaptively. The experimental results show that MulDE can effectively improve the performance and training speed of low-dimensional KGE models. The distilled 32-dimensional models are very competitive compared to some of state-or-the-art (SotA) high-dimensional methods on several commonly-used datasets.
更多查看译文
关键词
knowledge graph completion,multi-teacher
AI 理解论文
溯源树
样例
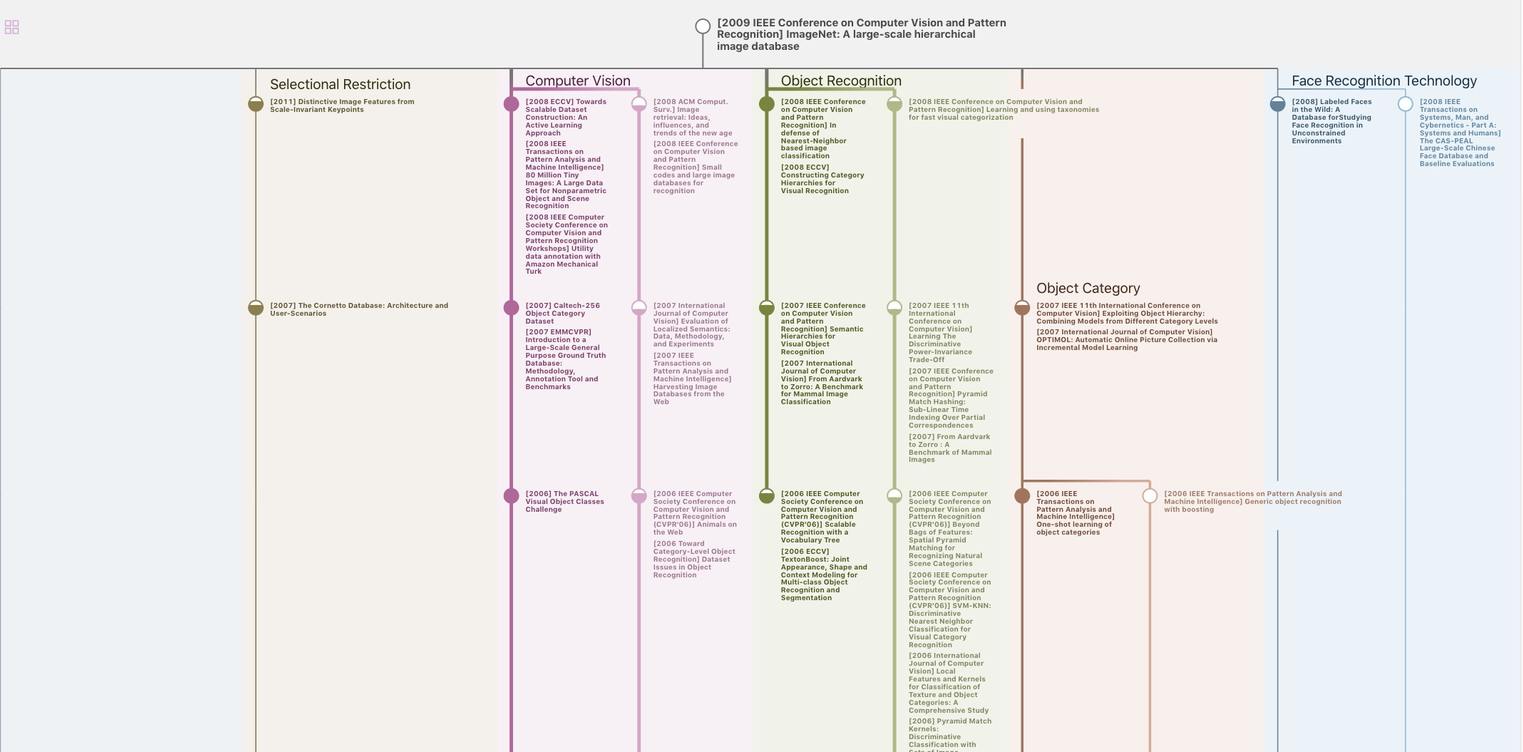
生成溯源树,研究论文发展脉络
Chat Paper
正在生成论文摘要