Online Policy Gradient for Model Free Learning of Linear Quadratic Regulators with √T Regret.
CoRR(2021)
摘要
We consider the task of learning to control a linear dynamical system under fixed quadratic costs, known as the Linear Quadratic Regulator (LQR) problem. While model-free approaches are often favorable in practice, thus far only model-based methods, which rely on costly system identification, have been shown to achieve regret that scales with the optimal dependence on the time horizon T. We present the first model-free algorithm that achieves similar regret guarantees. Our method relies on an efficient policy gradient scheme, and a novel and tighter analysis of the cost of exploration in policy space in this setting.
更多查看译文
关键词
model free learning,linear quadratic regulators,regret
AI 理解论文
溯源树
样例
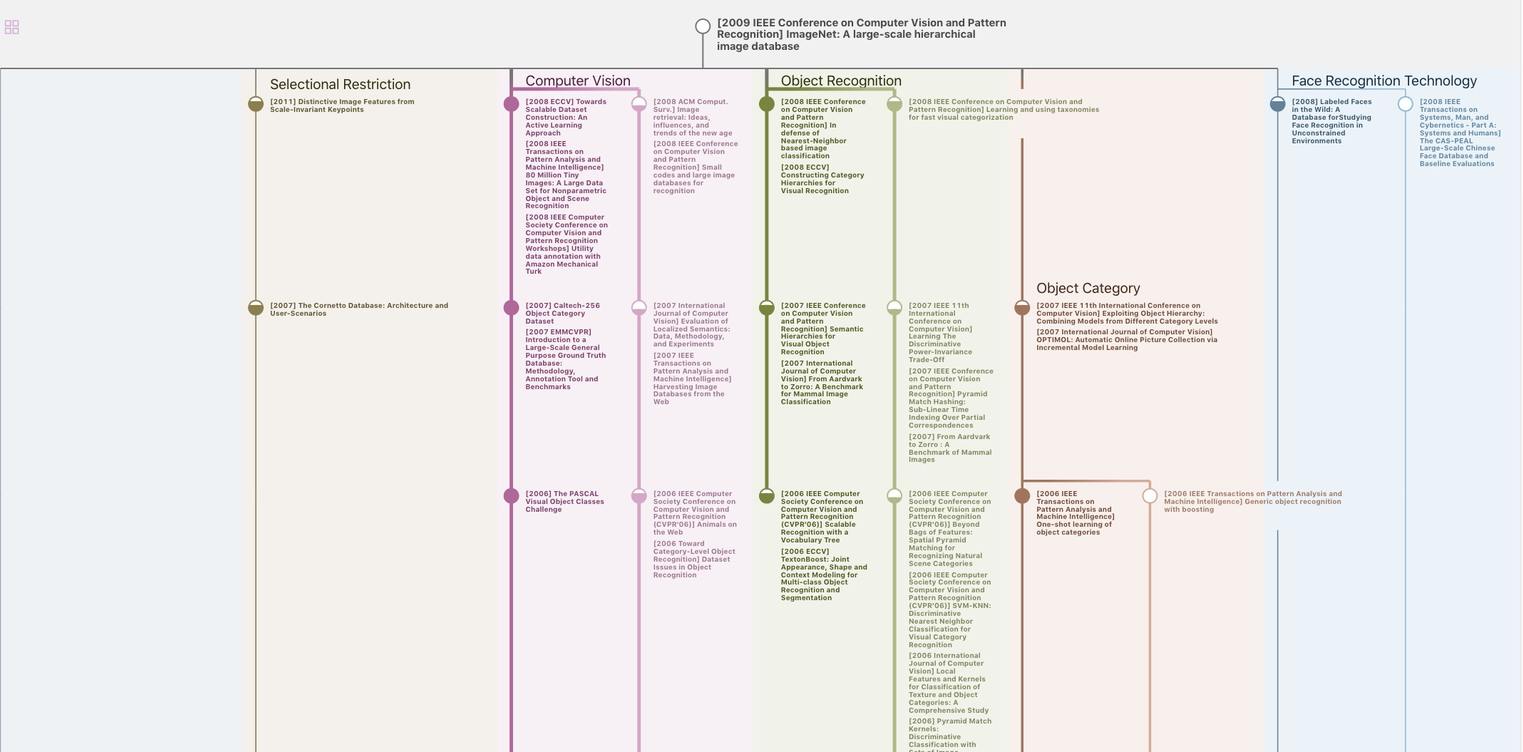
生成溯源树,研究论文发展脉络
Chat Paper
正在生成论文摘要