Learning How to Optimize Black-Box Functions With Extreme Limits on the Number of Function Evaluations.
CoRR(2021)
摘要
We consider black-box optimization in which only an extremely limited number of function evaluations, on the order of around 100, are affordable and the function evaluations must be performed in even fewer batches of a limited number of parallel trials. This is a typical scenario when optimizing variable settings that are very costly to evaluate, for example in the context of simulation-based optimization or machine learning hyperparameterization. We propose an original method that uses established approaches to propose a set of points for each batch and then down-selects from these candidate points to the number of trials that can be run in parallel. The key novelty of our approach lies in the introduction of a hyperparameterized method for down-selecting the number of candidates to the allowed batch-size, which is optimized offline using automated algorithm configuration. We tune this method for black box optimization and then evaluate on classical black box optimization benchmarks. Our results show that it is possible to learn how to combine evaluation points suggested by highly diverse black box optimization methods conditioned on the progress of the optimization. Compared with the state of the art in black box minimization and various other methods specifically geared towards few-shot minimization, we achieve an average reduction of 50\% of normalized cost, which is a highly significant improvement in performance.
更多查看译文
关键词
functions,functions,evaluations,learning,black-box
AI 理解论文
溯源树
样例
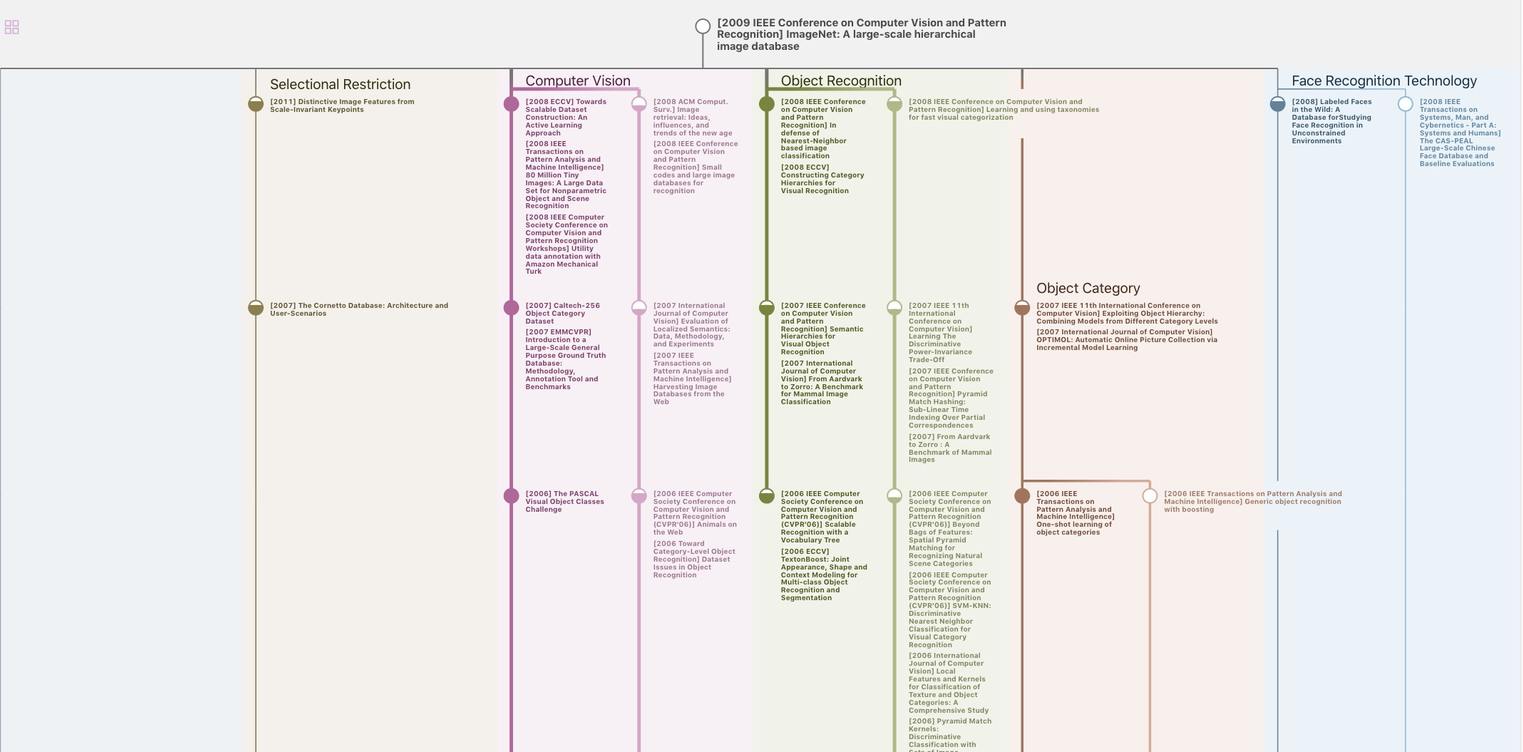
生成溯源树,研究论文发展脉络
Chat Paper
正在生成论文摘要