MIANet: Multi-level temporal information aggregation in mixed-periodicity time series forecasting tasks.
Eng. Appl. Artif. Intell.(2023)
摘要
Regular human activities generate a large number of time series with mixed periodicity that can reflect human behavior patterns and the societal working mechanism. When forecasting these time series, nonlinear neural networks often encounter some limitations, such as utilizing mixed-periodic patterns, balancing multi-level information, incorporating future vision, forecasting delays and scale insensitivity, which affect the forecasting accuracy. To address these problems, we propose the Multi-level Information Aggregation Network (MIANet), a novel neural network with four key characteristics: (i) a novel folded recurrent structure that dynamically updates the local and mini-local information at a global range in a compact manner; (ii) a new recurrent unit called Folded Convolution Aggregation Temporal Memory (FCATM) that extracts and aggregates neighbor-trends in local and mini-local data; (iii) a fusing decoder structure that promotes the sharing of forward-backward future information and adaptively adjusts relationships among adjacent points; and (iv) a new Skip-Autoregressive (SAR) linear strategy that addresses scale sensitivity issues. The SAR can be embedded as a plug-and-play component into other deep learning (DL) models. Compared with other baseline methods, MIANet obtains statistically significant improvements on six real-world datasets, as demonstrated by conducting two-sample t-tests, indicating that the MIANet can be applied to various predictive scenarios, such as road occupancy, electricity consumption, pedestrian flow and urban noise.
更多查看译文
关键词
Deep learning, Data mining, Time series forecasting, Sequence modeling, Encoder-decoder network, Time series with mixed periodicity
AI 理解论文
溯源树
样例
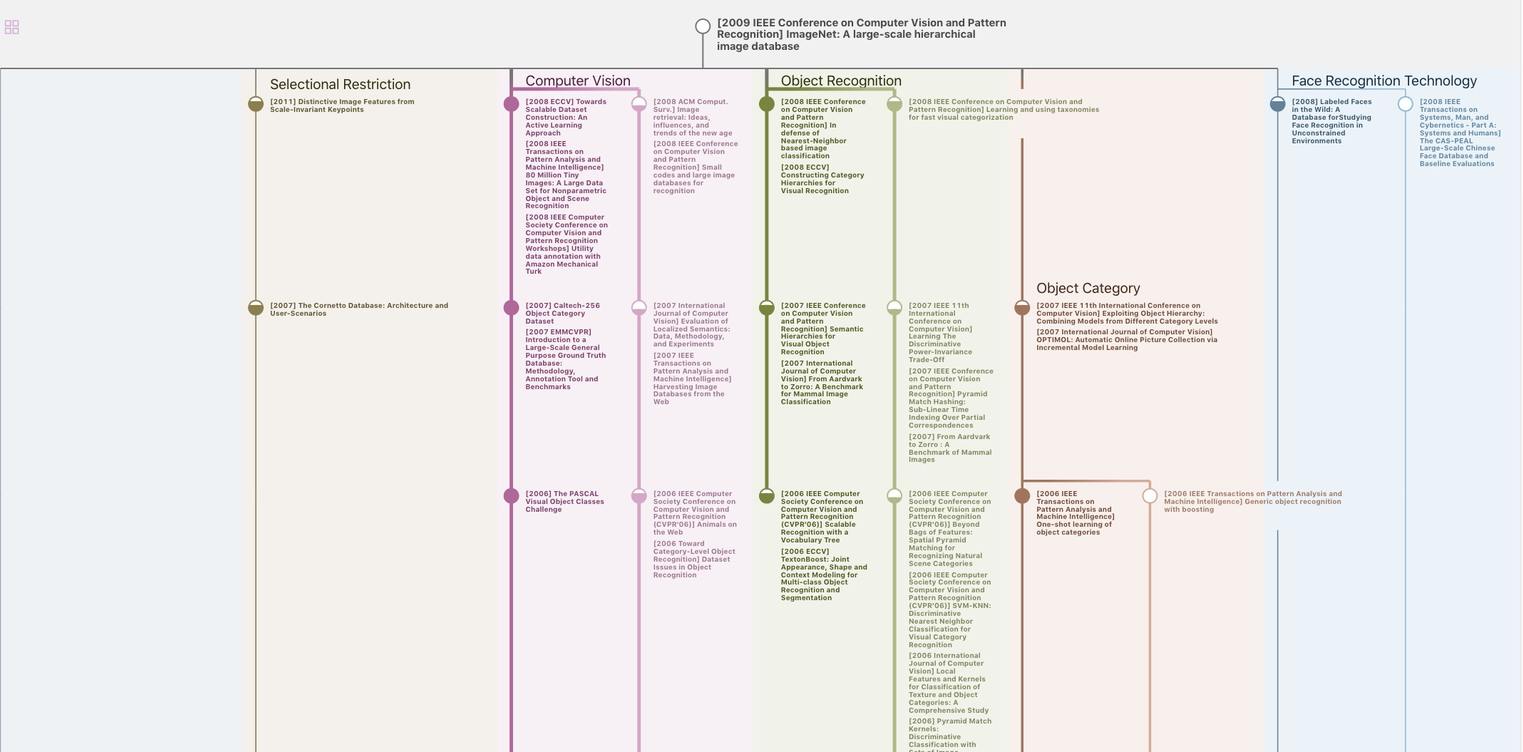
生成溯源树,研究论文发展脉络
Chat Paper
正在生成论文摘要