Field features: The impact in learning to rank approaches.
Appl. Soft Comput.(2023)
摘要
Learning to Rank approaches employ Machine Learning techniques for Information Retrieval. Traditionally, the features needed to train a ranking model are naively combined after being extracted from the various fields of the texts. Nevertheless, if not considered carefully, the learning process can make use of strongly correlated features. Moreover, the learned ranking models are not, to date, systematically analyzed in terms of how the field-based features affect their performances. In this work, the impact of using field-based features in Learning to Rank approaches is investigated. Specifically, the Field Learning to Rank technique is proposed to study if the field-based features perform better than the naively combined features. The experiments are conducted employing eight learning to rank approaches on two sizable benchmark datasets: MQ2007 and MQ2008. The models are assessed using three widely adopted Learning to Rank evaluation measures, namely Precision, Mean Average Precision, and Normalized Discounted Cumulative Gain. The results show that the use of field-based features achieve better performance than the naively combined features. Moreover, models aggregated from different fields further improve the ranking results. It is also observed that among the five examined fields, url and title are significantly more effective than wholedoc (full document), body, and anchor to build ranking models. Further, analyses indicate the existence of strong correlations between field features, such as the features from body and wholedoc, title and anchor, or title and url. The proposed Field Learning to Rank technique is shown to have the advantage of avoiding the combination of correlated features. These findings imply that the use of field-based features for training ranking models is valuable for enhancing the effectiveness of Learning to Rank approaches.
更多查看译文
关键词
Learning to Rank,Field Features,Aggregation,Information Retrieval
AI 理解论文
溯源树
样例
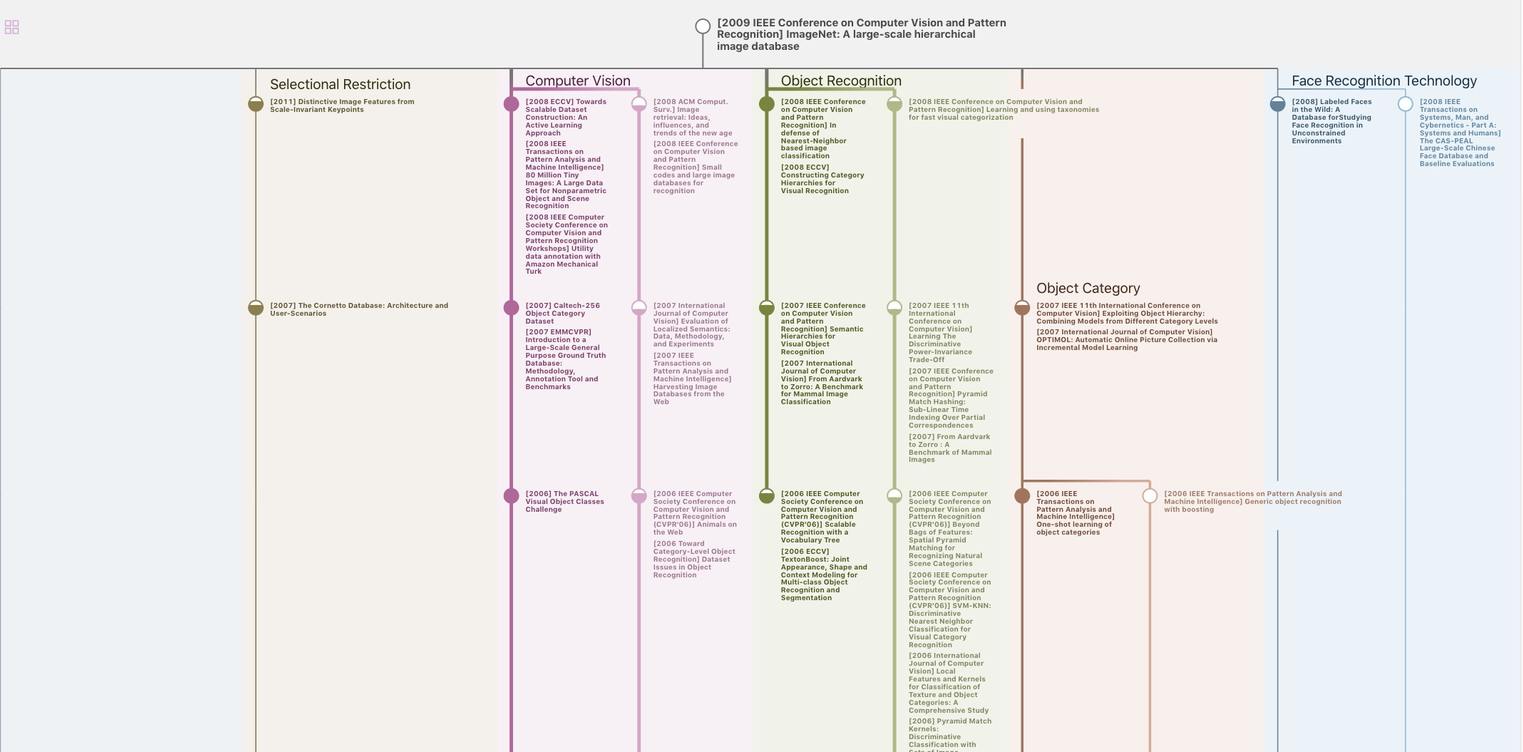
生成溯源树,研究论文发展脉络
Chat Paper
正在生成论文摘要