Development and research of triangle-filter convolution neural network for fuel reloading optimization of block-type HTGRs.
Appl. Soft Comput.(2023)
摘要
The problem of fuel reloading optimization is very demanding, which requires to search for the optimal suitable core configuration within a very huge solution space. To solve this problem, updating of optimization solutions and quantitative evaluation of core configurations are two main steps. With the development of computing science, the meta-heuristic algorithms (MHAs) turn out to be effective for updating optimization solutions; while the artificial neural networks (ANNs) proves to be practical for the other. However, there is few research for the application of convolution neural networks (CNNs). For any given image-like inputs, CNNs could quickly calculate the outputs mainly by convolution operators, using rectangle filters to scan the full inputs. However, the geometry of some reactors is not rectangle so that using rectangle filters fails to traverse. Aiming at solving the problems of fuel reloading optimization for block-type HTGRs, the triangle-filter convolution neural networks (TFCNNs) are developed. At the same time, uniform method and ring-ranked method are also developed for determining the weights of TFCNNs. Combined with genetic algorithm and particle swarm optimization, two wide-applied MHAs, TFCNNs are applied to solve the problems of fuel reloading optimization for block-type HTGRs. The results show that the TFCNNs could greatly predict the optimization parameters of testing data set with accuracy higher than 90%. In addition, comparing to DRAGON V4 program, the calculation time of TFCNNs is even less than 1.30% of it, while comparing to BP-ANNs, the ratio of feasible solutions by TFCNNs is evidently higher.(c) 2023 Elsevier B.V. All rights reserved.
更多查看译文
关键词
fuel,triangle-filter,block-type
AI 理解论文
溯源树
样例
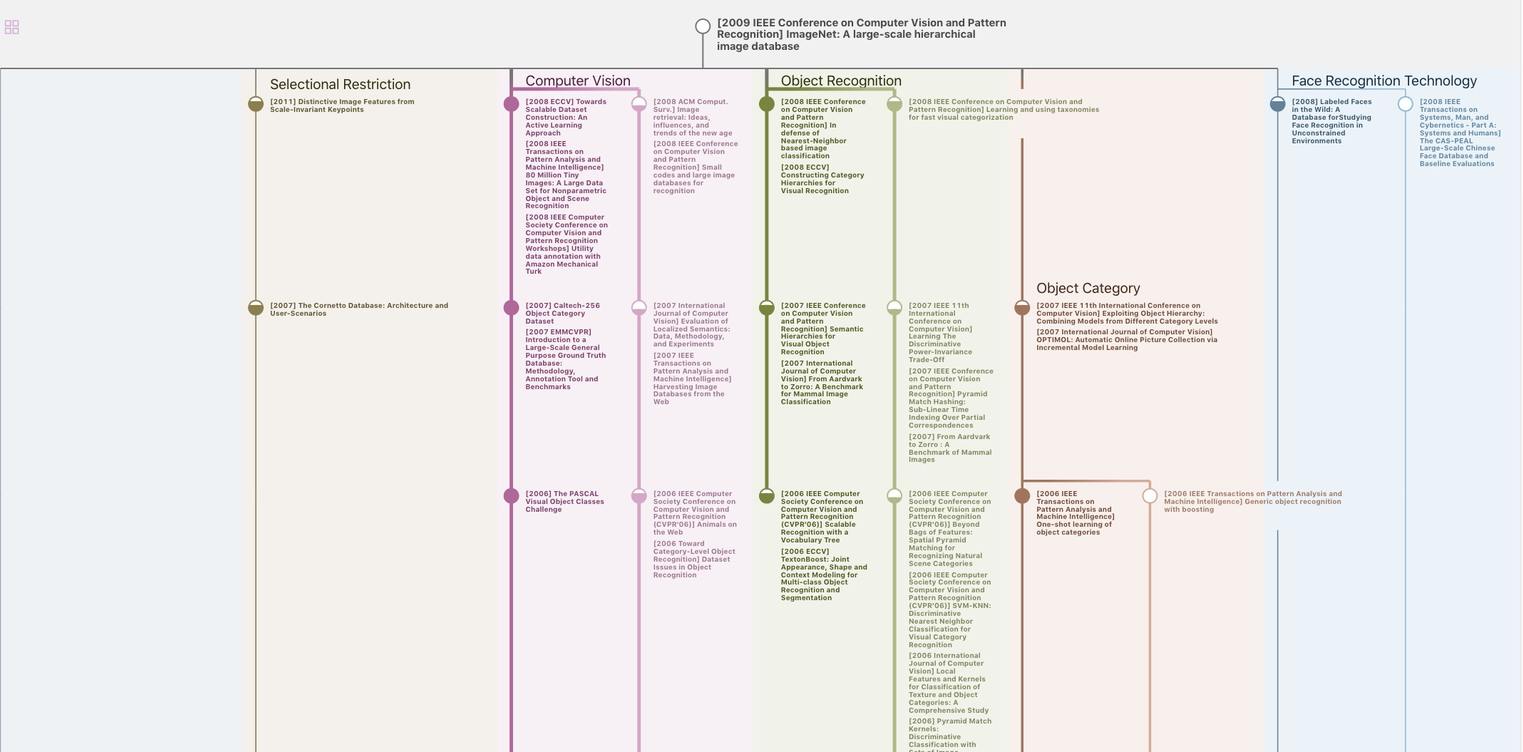
生成溯源树,研究论文发展脉络
Chat Paper
正在生成论文摘要