An Integrated Framework for COVID-19 Classification Based on Ensembles of Deep Features and Entropy Coded GLEO Feature Selection.
Int. J. Uncertain. Fuzziness Knowl. Based Syst.(2023)
摘要
COVID-19 is a challenging worldwide pandemic disease nowadays that spreads from person to person in a very fast manner. It is necessary to develop an automated technique for COVID-19 identification. This work investigates a new framework that predicts COVID-19 based on X-ray images. The suggested methodology contains core phases as preprocessing, feature extraction, selection and categorization. The Guided and 2D Gaussian filters are utilized for image improvement as a preprocessing phase. The outcome is then passed to 2D-superpixel method for region of interest (ROI). The pre-trained models such as Darknet-53 and Densenet-201 are then applied for features extraction from the segmented images. The entropy coded GLEO features selection is based on the extracted and selected features, and ensemble serially to produce a single feature vector. The single vector is finally supplied as an input to the variations of the SVM classifier for the categorization of the normal/abnormal (COVID-19) X-rays images. The presented approach is evaluated with different measures known as accuracy, recall, F1 Score, and precision. The integrated framework for the proposed system achieves the acceptable accuracies on the SVM Classifiers, which authenticate the proposed approach's effectiveness.
更多查看译文
关键词
COVID-19, Darknet-53, Densenet-201, entropy, GLEO, SVM, superpixel, X-ray
AI 理解论文
溯源树
样例
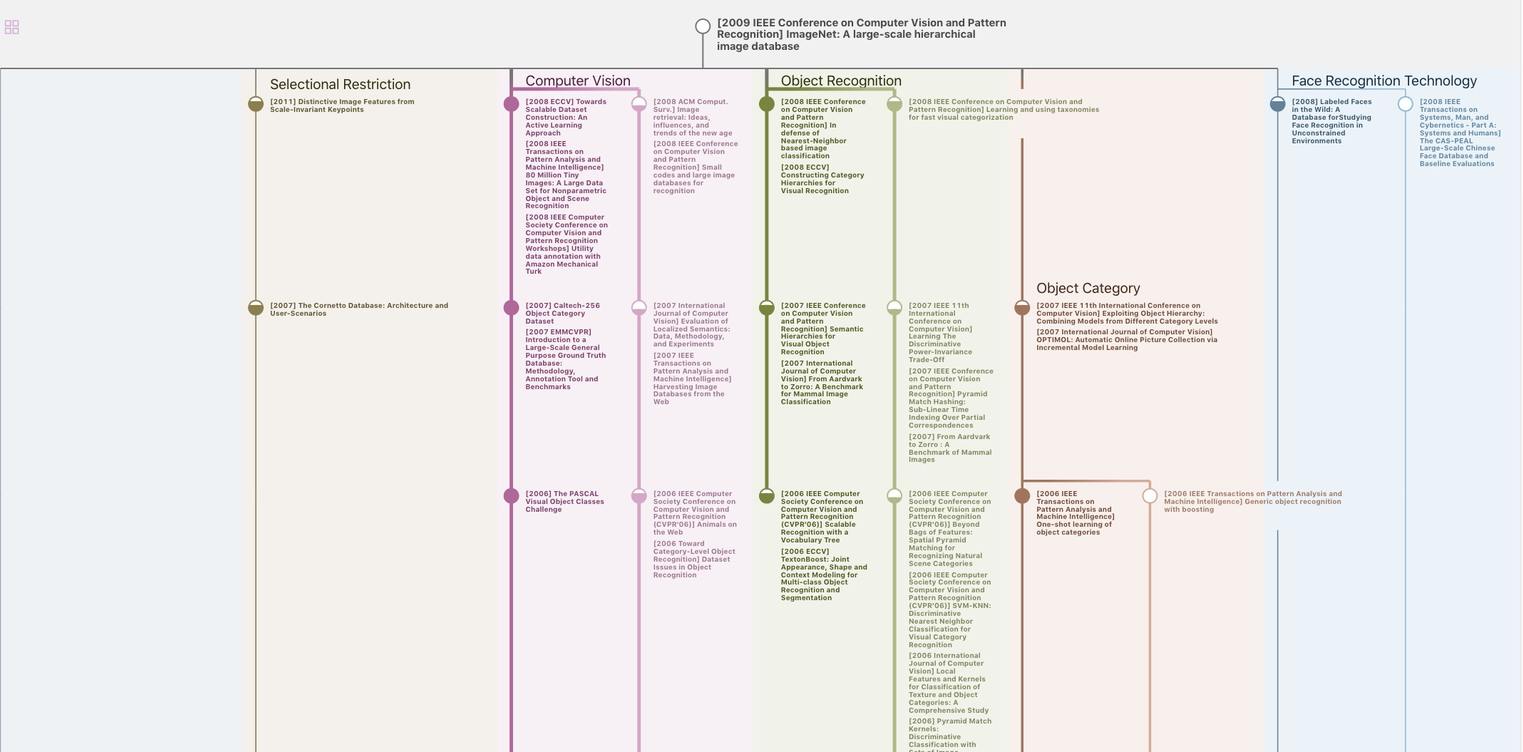
生成溯源树,研究论文发展脉络
Chat Paper
正在生成论文摘要