A Multiscale Nonlocal Feature Extraction Network for Breast Lesion Segmentation in Ultrasound Images
IEEE Trans. Instrum. Meas.(2023)
摘要
Breast lesion segmentation in ultrasound images is of great importance since it can help us to characterize and localize lesion regions. However, low-quality imaging, blurred boundary, and variable lesion shapes bring challenges to accurate segmentation. In recent years, many U-Net variants have been proposed and successfully applied to breast lesion segmentation. However, these methods suffer from two limitations: 1) ignoring the ability to capture rich global context information and 2) introducing extra complex operations. To alleviate these challenges, we propose a multiscale nonlocal feature extraction network (MNFE-Net) for accurately segmenting breast lesions. The core idea includes three points: 1) parallel encoder (PE) models long-range dependencies; 2) multiscale feature module (MFM) refines local features without introducing extra complex operations; and 3) global feature guidance module (GFGM) extracts global semantic information. MNFE-Net mainly has the following advantages: 1) the method has excellent performance for segmentation of malignant breast lesions; 2) the PE increases network parameters without significantly decreasing inference speed; and 3) the method is easy to understand and execute. Extensive experiment results with six state-of-the-art (SOTA) methods on three public breast ultrasound datasets demonstrate the superior segmentation performance of our proposed MNFE-Net.
更多查看译文
关键词
Feature extraction,Image segmentation,Lesions,Transformers,Breast,Ultrasonic imaging,Semantics,Breast ultrasound images segmentation,convolutional neural networks,transformer
AI 理解论文
溯源树
样例
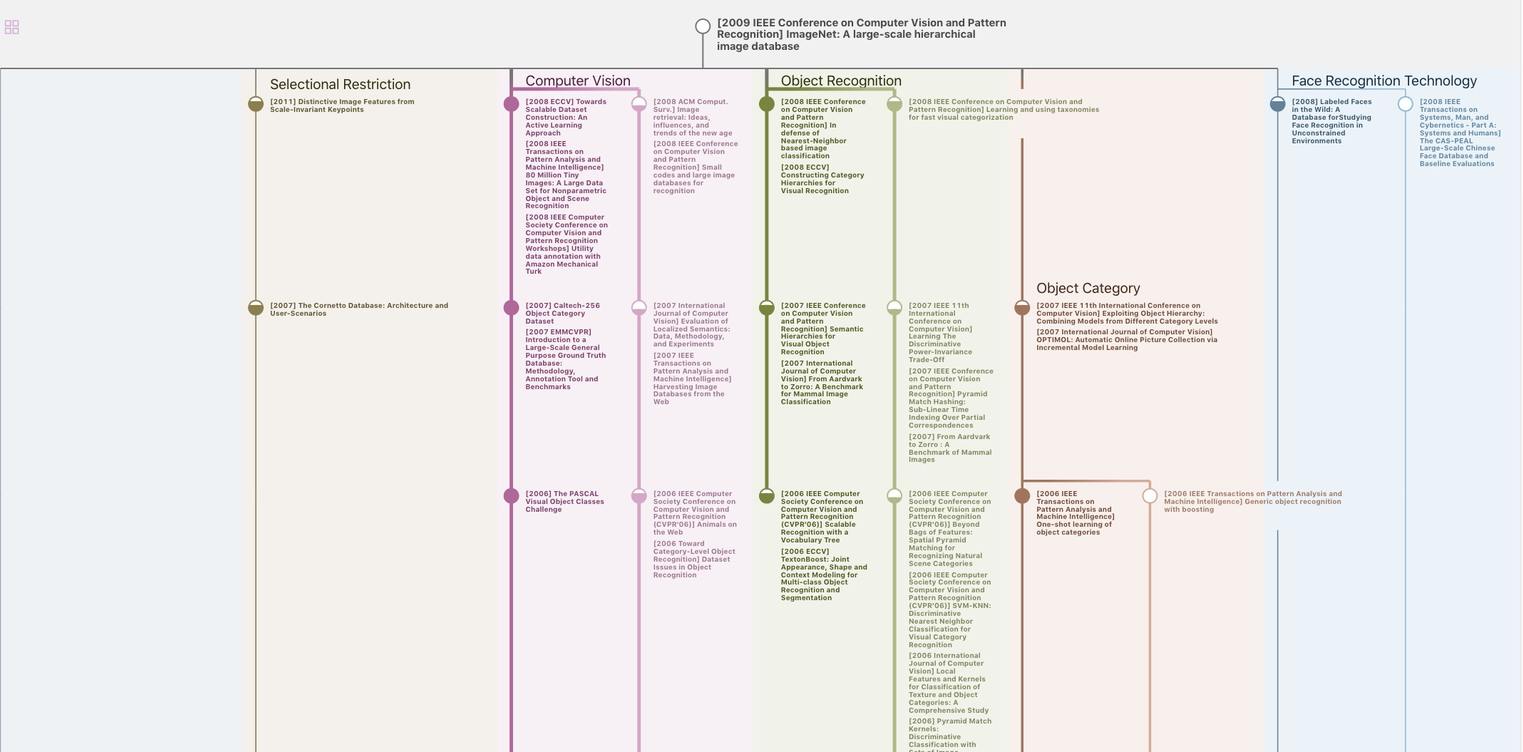
生成溯源树,研究论文发展脉络
Chat Paper
正在生成论文摘要