Expanding Synthetic Real-World Degradations for Blind Video Super Resolution
CoRR(2023)
摘要
Video super-resolution (VSR) techniques, especially deep-learning-based algorithms, have drastically improved over the last few years and shown impressive performance on synthetic data. However, their performance on real-world video data suffers because of the complexity of real-world degradations and misaligned video frames. Since obtaining a synthetic dataset consisting of low-resolution (LR) and high-resolution (HR) frames are easier than obtaining real-world LR and HR images, in this paper, we propose synthesizing real-world degradations on synthetic training datasets. The proposed synthetic real-world degradations (SRWD) include a combination of the blur, noise, downsampling, pixel binning, and image and video compression artifacts. We then propose using a random shuffling-based strategy to simulate these degradations on the training datasets and train a single end-to-end deep neural network (DNN) on the proposed larger variation of realistic synthesized training data. Our quantitative and qualitative comparative analysis shows that the proposed training strategy using diverse realistic degradations improves the performance by 7.1 % in terms of NRQM compared to RealBasicVSR and by 3.34 % compared to BSRGAN on the VideoLQ dataset. We also introduce a new dataset that contains high-resolution real-world videos that can serve as a common ground for bench-marking.
更多查看译文
关键词
blind video super resolution,deep-learning-based algorithms,diverse realistic degradations,low-resolution,misaligned video frames,random shuffling-based strategy,real-world LR,real-world video data suffers,real-world videos,realistic synthesized training data,single end-to-end deep neural network,synthetic data,synthetic dataset,synthetic real-world degradations,synthetic training datasets,training strategy,video compression artifacts,video super-resolution techniques
AI 理解论文
溯源树
样例
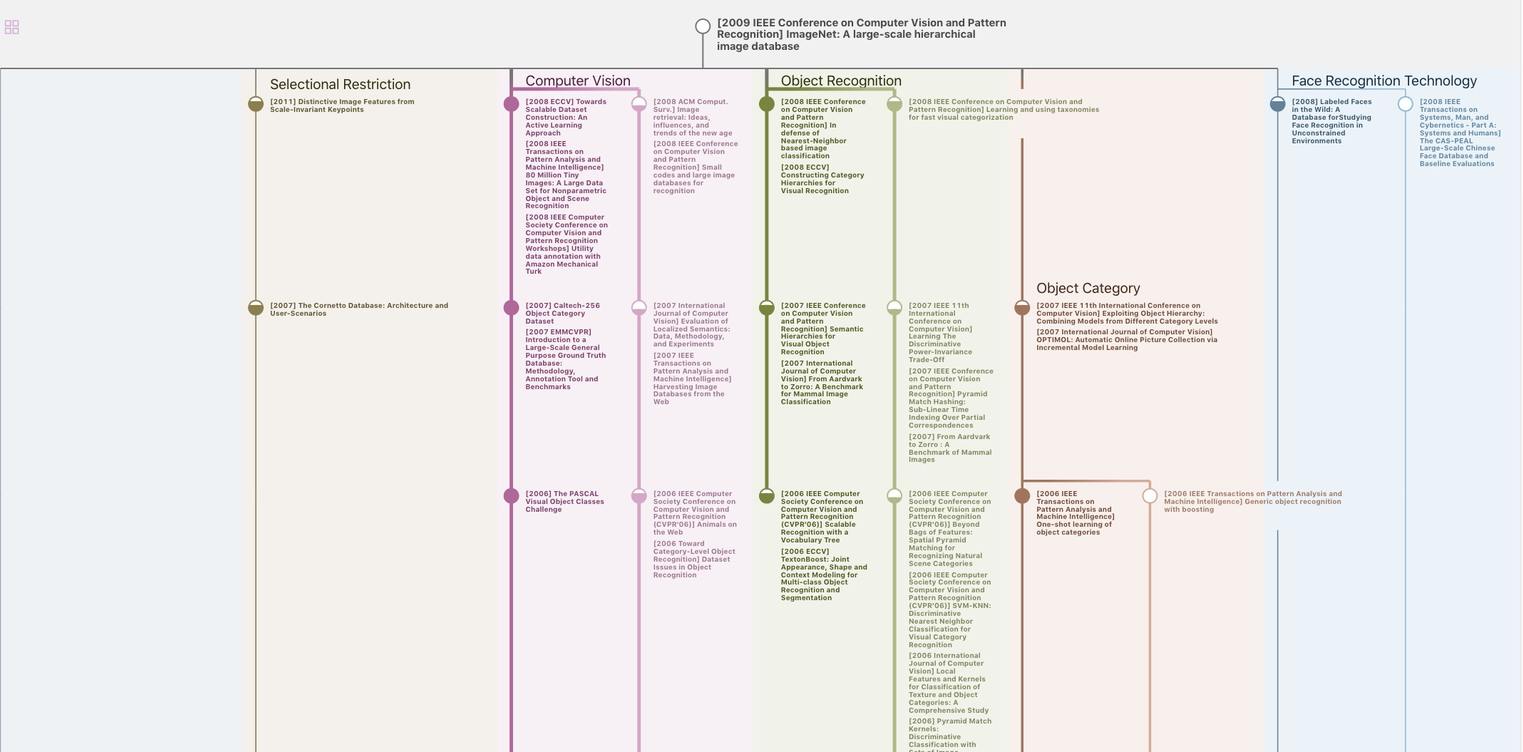
生成溯源树,研究论文发展脉络
Chat Paper
正在生成论文摘要