Edge-aware Consistent Stereo Video Depth Estimation
CoRR(2023)
摘要
Video depth estimation is crucial in various applications, such as scene reconstruction and augmented reality. In contrast to the naive method of estimating depths from images, a more sophisticated approach uses temporal information, thereby eliminating flickering and geometrical inconsistencies. We propose a consistent method for dense video depth estimation; however, unlike the existing monocular methods, ours relates to stereo videos. This technique overcomes the limitations arising from the monocular input. As a benefit of using stereo inputs, a left-right consistency loss is introduced to improve the performance. Besides, we use SLAM-based camera pose estimation in the process. To address the problem of depth blurriness during test-time training (TTT), we present an edge-preserving loss function that improves the visibility of fine details while preserving geometrical consistency. We show that our edge-aware stereo video model can accurately estimate the dense depth maps.
更多查看译文
关键词
video,edge-aware
AI 理解论文
溯源树
样例
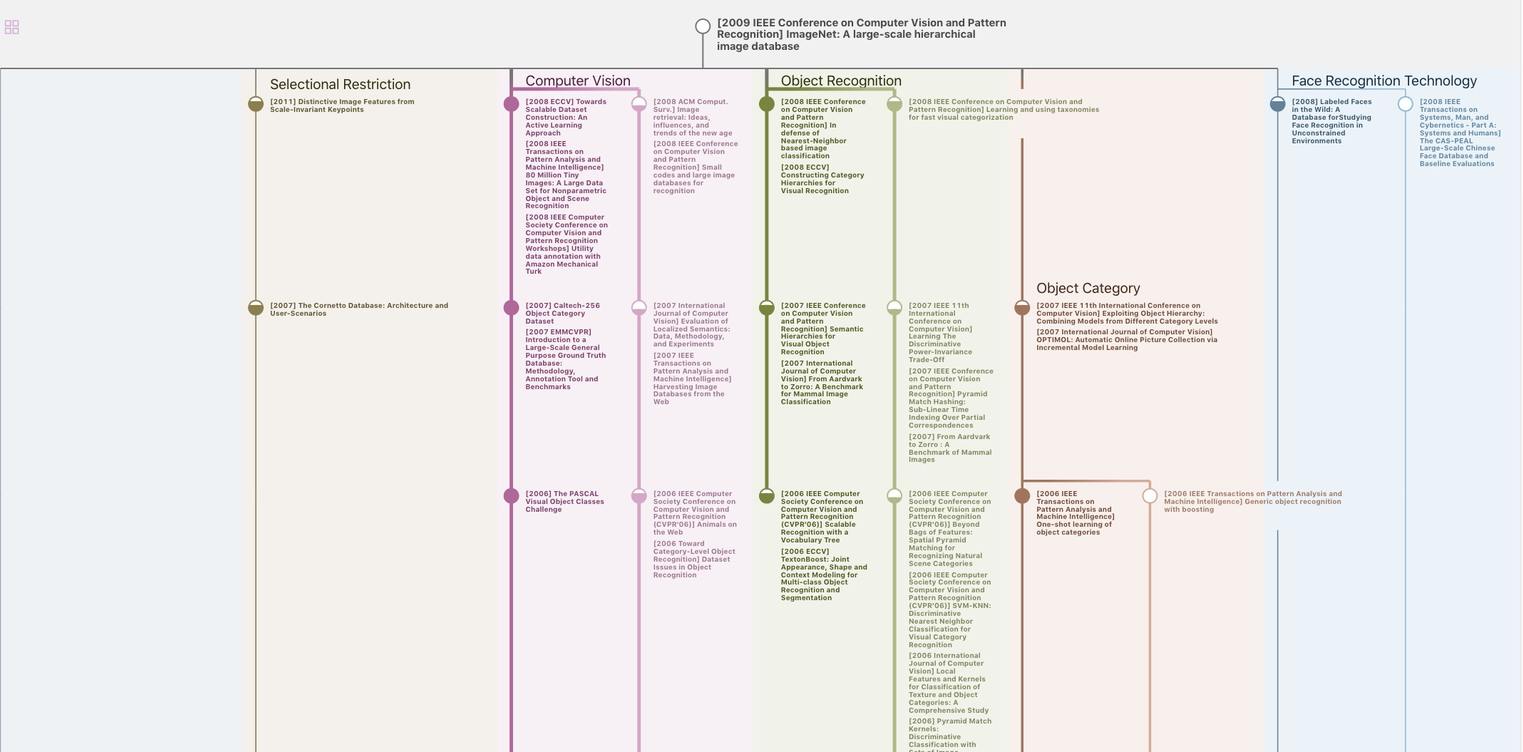
生成溯源树,研究论文发展脉络
Chat Paper
正在生成论文摘要