Faithful Question Answering with Monte-Carlo Planning
conf_acl(2023)
摘要
Although large language models demonstrate remarkable question-answering performances, revealing the intermediate reasoning steps that the models faithfully follow remains challenging. In this paper, we propose FAME (FAithful question answering with MontE-carlo planning) to answer questions based on faithful reasoning steps. The reasoning steps are organized as a structured entailment tree, which shows how premises are used to produce intermediate conclusions that can prove the correctness of the answer. We formulate the task as a discrete decision-making problem and solve it through the interaction of a reasoning environment and a controller. The environment is modular and contains several basic task-oriented modules, while the controller proposes actions to assemble the modules. Since the search space could be large, we introduce a Monte-Carlo planning algorithm to do a look-ahead search and select actions that will eventually lead to high-quality steps. FAME achieves state-of-the-art performance on the standard benchmark. It can produce valid and faithful reasoning steps compared with large language models with a much smaller model size.
更多查看译文
关键词
planning,monte-carlo
AI 理解论文
溯源树
样例
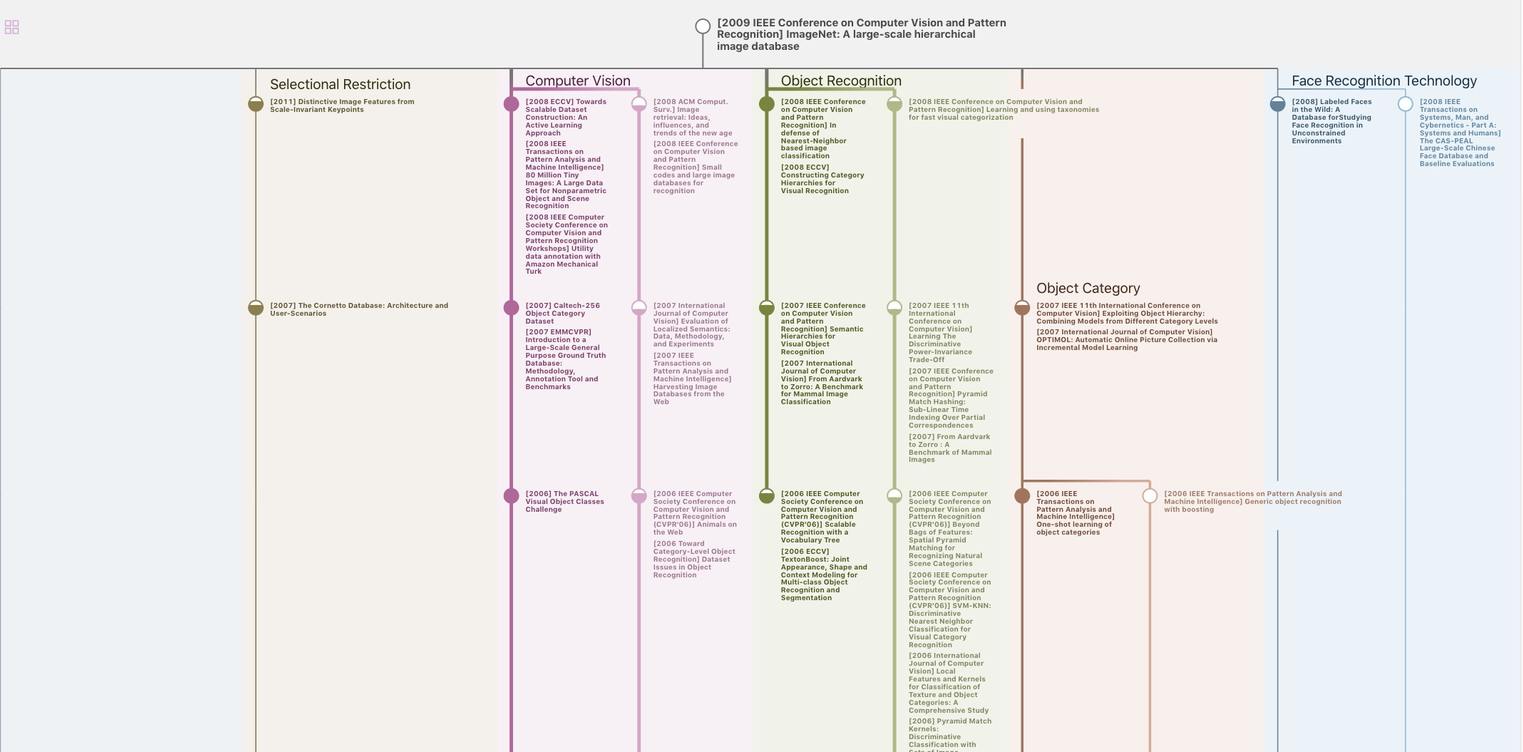
生成溯源树,研究论文发展脉络
Chat Paper
正在生成论文摘要