A Preconditioned Riemannian Gradient Descent Algorithm for Low-Rank Matrix Recovery
arXiv (Cornell University)(2023)
摘要
The low-rank matrix recovery problem often arises in various fields, including signal processing, machine learning, and imaging science. The Riemannian gradient descent (RGD) algorithm has proven to be an efficient algorithm for solving this problem. In this paper, we present a preconditioned Riemannian gradient descent (PRGD) for low-rank matrix recovery. The preconditioner, noted for its simplicity and computational efficiency, is constructed by weighting the (i,j)-th entry of the gradient matrix according to the norms of the i-th row and the j-th column. We establish the theoretical recovery guarantee for PRGD under the restricted isometry property assumption. Experimental results indicate that PRGD can accelerate RGD by up to tenfold in solving low-rank matrix recovery problems such as matrix completion.
更多查看译文
关键词
matrix,low-rank
AI 理解论文
溯源树
样例
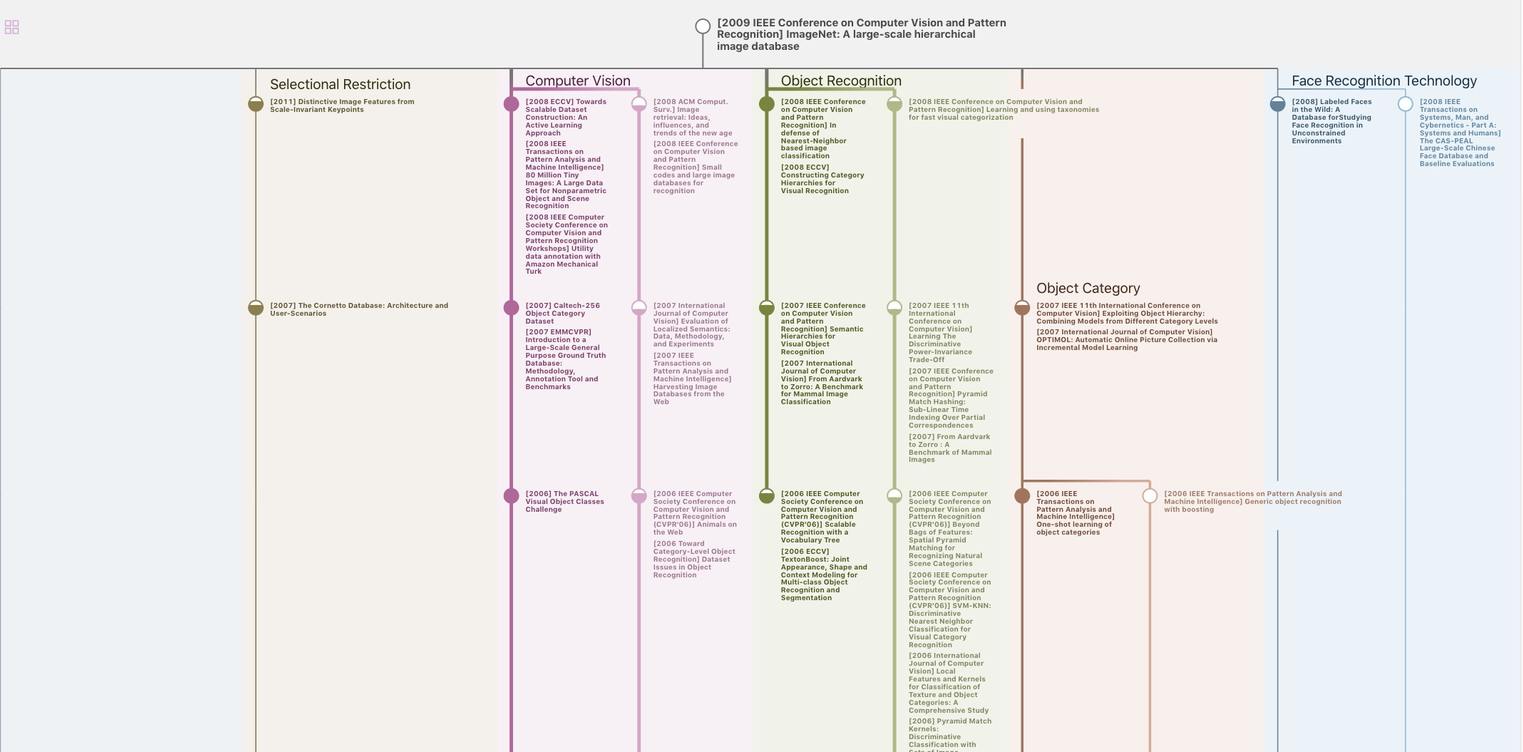
生成溯源树,研究论文发展脉络
Chat Paper
正在生成论文摘要