Cross-stream contrastive learning for self-supervised skeleton-based action recognition
Image and Vision Computing(2023)
摘要
Self-supervised skeleton-based action recognition enjoys a rapid growth along with the development of contras-tive learning. The existing methods rely on imposing invariance to augmentations of 3D skeleton within a single data stream, which merely leverages the easy positive pairs and limits the ability to explore the complicated movement patterns. In this paper, we advocate that the defect of single-stream contrast and the lack of necessary feature transformation are responsible for easy positives, and therefore propose a Cross-Stream Contrastive Learning framework for skeleton-based action Representation learning (CSCLR). Specifically, the proposed CSCLR not only utilizes intra-stream contrast pairs, but introduces inter-stream contrast pairs as hard samples to formulate a better representation learning. Besides, to further exploit the potential of positive pairs and in-crease the robustness of self-supervised representation learning, we propose a Positive Feature Transformation (PFT) strategy which adopts feature-level manipulation to increase the variance of positive pairs. To validate the effectiveness of our method, we conduct extensive experiments on three benchmark datasets NTU-RGB + D 60, NTU-RGB + D 120 and PKU-MMD. Experimental results show that our proposed CSCLR exceeds the state-of-the-art methods on a diverse range of evaluation protocols. (c) 2023 Published by Elsevier B.V.
更多查看译文
关键词
Self-supervised learning,Contrastive learning,Skeleton-based action recognition
AI 理解论文
溯源树
样例
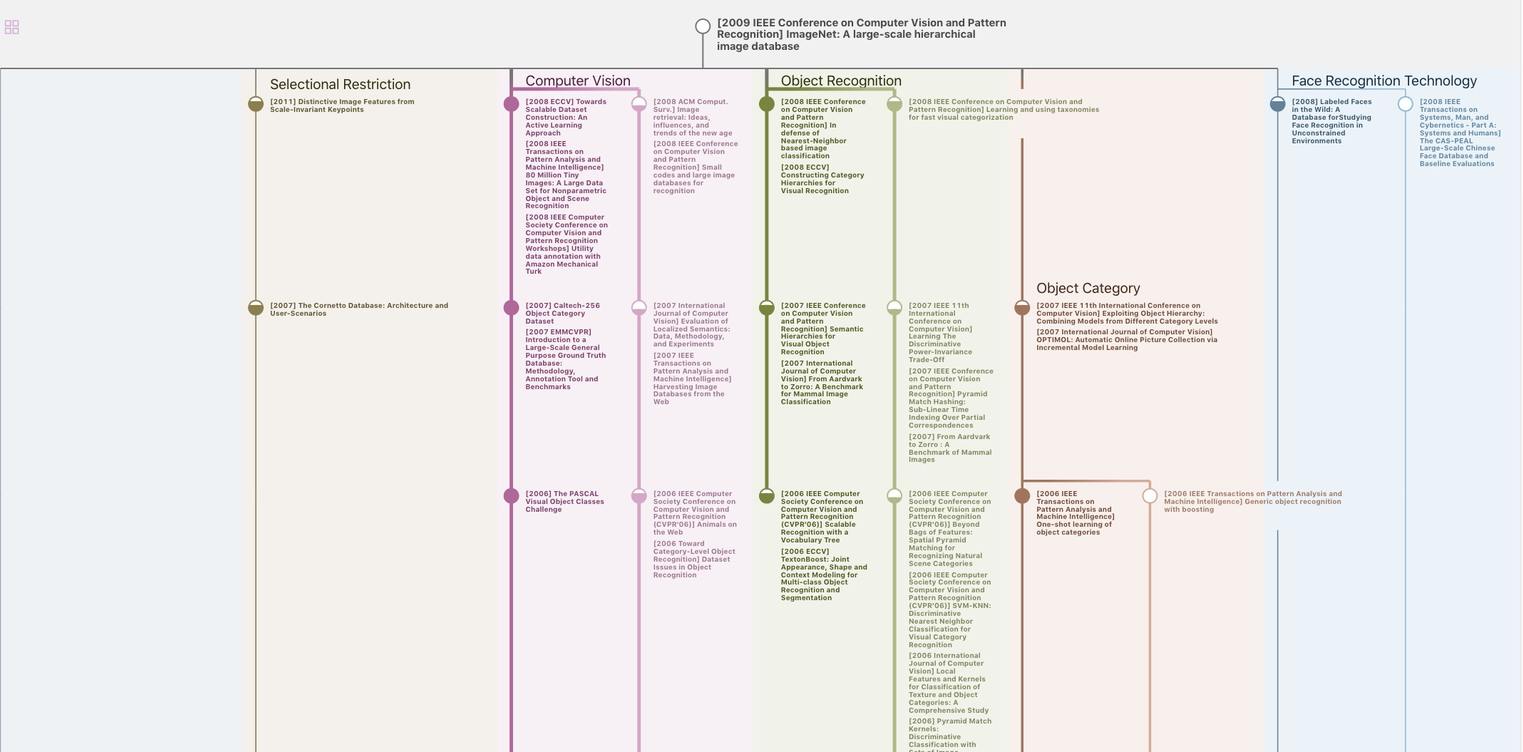
生成溯源树,研究论文发展脉络
Chat Paper
正在生成论文摘要