Synergies Between Federated Learning and O-RAN: Towards an Elastic Virtualized Architecture for Multiple Distributed Machine Learning Services
CoRR(2023)
摘要
Federated learning (FL) is the most popular distributed machine learning technique. However, implementation of FL over modern wireless networks faces key challenges caused by (i) dynamics of the network conditions, (ii) coexistence of multiple FL services/tasks in the system, and (iii) concurrent execution of FL services with other network services, which are not jointly considered in prior works. Motivated by these challenges, we introduce a generic FL paradigm over next-generation (NextG) networks, called dynamic multi-service FL (DMS-FL). We identify three unexplored design considerations in DMS-FL: (i) FL service operator accumulation, (ii) wireless resource fragmentation, and (iii) signal strength fluctuations. We take the first steps towards addressing these design considerations through proposing a novel distributed ML architecture called elastic virtualized FL (EV-FL). EV-FL unleashes the full potential of Open RAN (O-RAN) systems and introduces an elastic resource provisioning methodology to execute FL services. It further constitutes a multi-time-scale FL management system that introduces three dimensions into existing FL architectures: (i) virtualization, (ii) scalability, and (iii) elasticity. Through investigating EV-FL, we reveal a series of open research directions for future work. We finally simulate EV-FL to demonstrate its potential to save wireless resources and increase fairness among FL services.
更多查看译文
关键词
federated learning,machine learning,o-ran
AI 理解论文
溯源树
样例
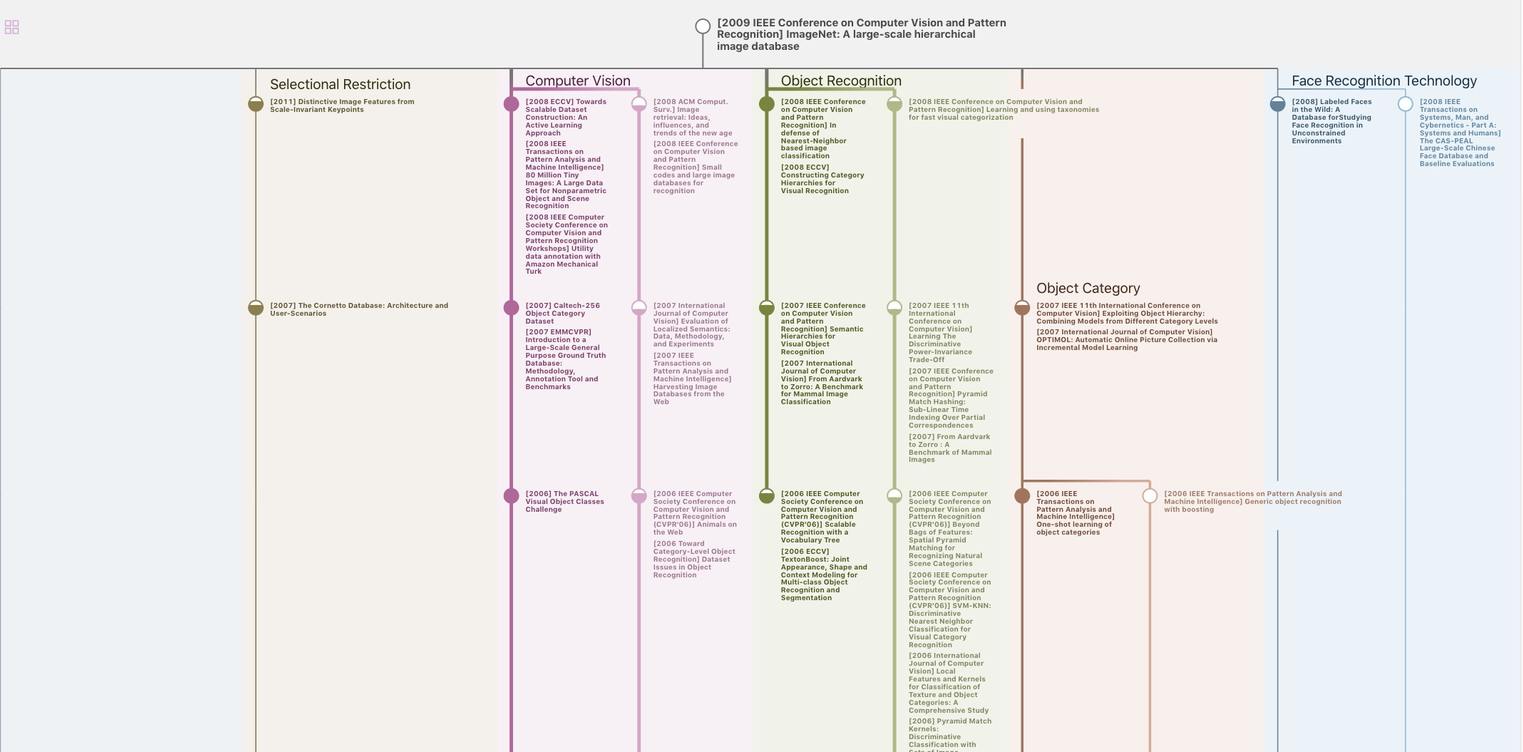
生成溯源树,研究论文发展脉络
Chat Paper
正在生成论文摘要