FreeLM: Fine-Tuning-Free Language Model
CoRR(2023)
摘要
Pre-trained language models (PLMs) have achieved remarkable success in NLP tasks. Despite the great success, mainstream solutions largely follow the pre-training then finetuning paradigm, which brings in both high deployment costs and low training efficiency. Nevertheless, fine-tuning on a specific task is essential because PLMs are only pre-trained with language signal from large raw data. In this paper, we propose a novel fine-tuning-free strategy for language models, to consider both language signal and teacher signal. Teacher signal is an abstraction of a battery of downstream tasks, provided in a unified proposition format. Trained with both language and strong task-aware teacher signals in an interactive manner, our FreeLM model demonstrates strong generalization and robustness. FreeLM outperforms large models e.g., GPT-3 and InstructGPT, on a range of language understanding tasks in experiments. FreeLM is much smaller with 0.3B parameters, compared to 175B in these models.
更多查看译文
关键词
language,model,fine-tuning-free
AI 理解论文
溯源树
样例
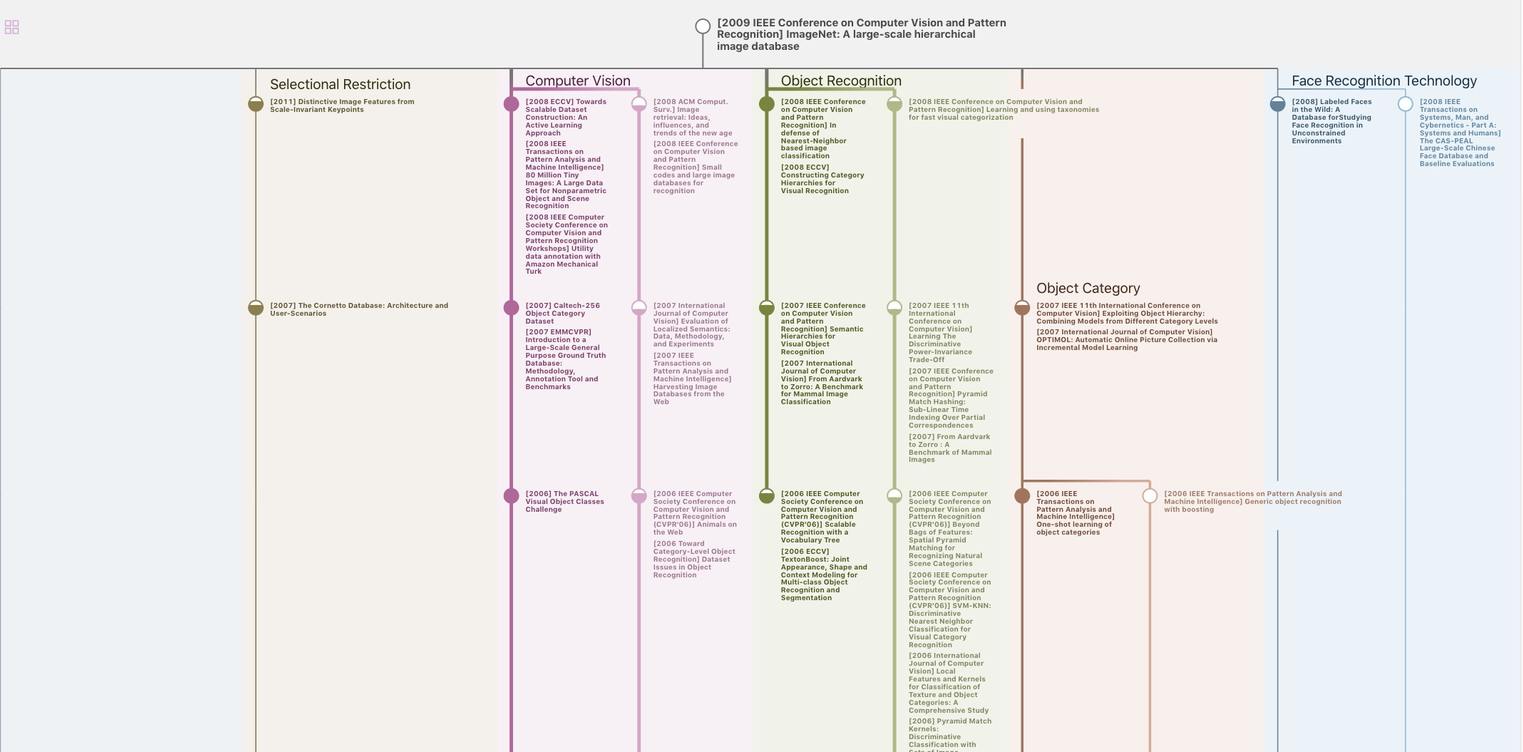
生成溯源树,研究论文发展脉络
Chat Paper
正在生成论文摘要