How to Unleash the Power of Large Language Models for Few-shot Relation Extraction?
CoRR(2023)
摘要
Scaling language models have revolutionized widespread NLP tasks, yet little comprehensively explored few-shot relation extraction with large language models. In this paper, we investigate principal methodologies, in-context learning and data generation, for few-shot relation extraction via GPT-3.5 through exhaustive experiments. To enhance few-shot performance, we further propose task-related instructions and schema-constrained data generation. We observe that in-context learning can achieve performance on par with previous prompt learning approaches, and data generation with the large language model can boost previous solutions to obtain new state-of-the-art few-shot results on four widely-studied relation extraction datasets. We hope our work can inspire future research for the capabilities of large language models in few-shot relation extraction. Code is available in https://github.com/zjunlp/DeepKE/tree/main/example/llm.
更多查看译文
关键词
large language models,relation
AI 理解论文
溯源树
样例
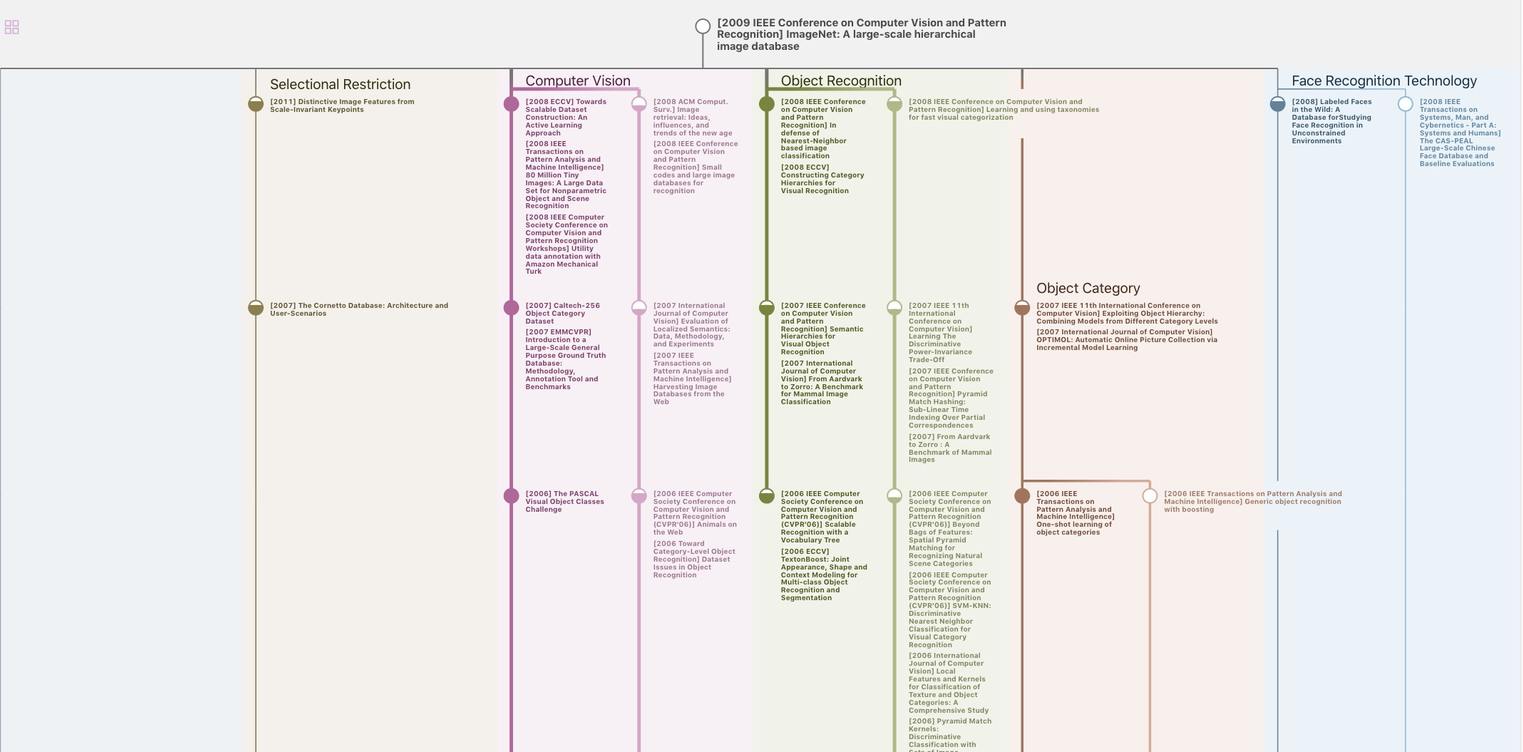
生成溯源树,研究论文发展脉络
Chat Paper
正在生成论文摘要