Memory of recurrent networks: Do we compute it right?
CoRR(2023)
摘要
Numerical evaluations of the memory capacity (MC) of recurrent neural networks reported in the literature often contradict well-established theoretical bounds. In this paper, we study the case of linear echo state networks, for which the total memory capacity has been proven to be equal to the rank of the corresponding Kalman controllability matrix. We shed light on various reasons for the inaccurate numerical estimations of the memory, and we show that these issues, often overlooked in the recent literature, are of an exclusively numerical nature. More explicitly, we prove that when the Krylov structure of the linear MC is ignored, a gap between the theoretical MC and its empirical counterpart is introduced. As a solution, we develop robust numerical approaches by exploiting a result of MC neutrality with respect to the input mask matrix. Simulations show that the memory curves that are recovered using the proposed methods fully agree with the theory.
更多查看译文
关键词
recurrent networks,memory
AI 理解论文
溯源树
样例
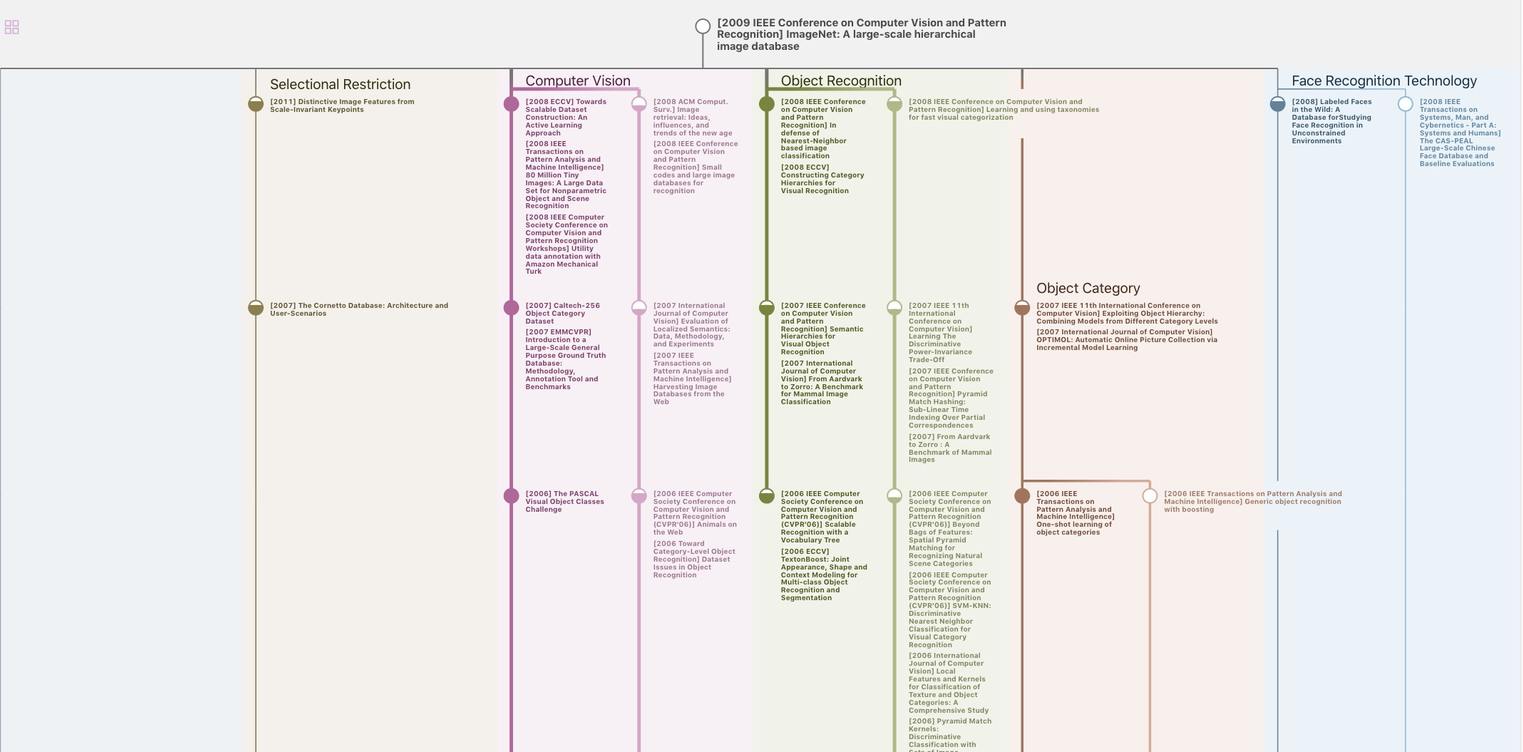
生成溯源树,研究论文发展脉络
Chat Paper
正在生成论文摘要