Machine-Learned Invertible Coarse Graining for Multiscale Molecular Modeling
CoRR(2023)
摘要
Multiscale molecular modeling is widely applied in scientific research of molecular properties over large time and length scales. Two specific challenges are commonly present in multiscale modeling, provided that information between the coarse and fine representations of molecules needs to be properly exchanged: One is to construct coarse grained (CG) models by passing information from the fine to coarse levels; the other is to restore finer molecular details given CG configurations. Although these two problems are commonly addressed independently, in this work, we present a theory connecting them, and develop a methodology called Cycle Coarse Graining (CCG) to solve both problems in a unified manner. In CCG, reconstruction can be achieved via a tractable optimization process, leading to a general method to retrieve fine details from CG simulations, which in turn, delivers a new solution to the CG problem, yielding an efficient way to calculate free energies in a rare-event-free manner. CCG thus provides a systematic way for multiscale molecular modeling, where the finer details of CG simulations can be efficiently retrieved, and the CG models can be improved consistently.
更多查看译文
关键词
multiscale molecular
AI 理解论文
溯源树
样例
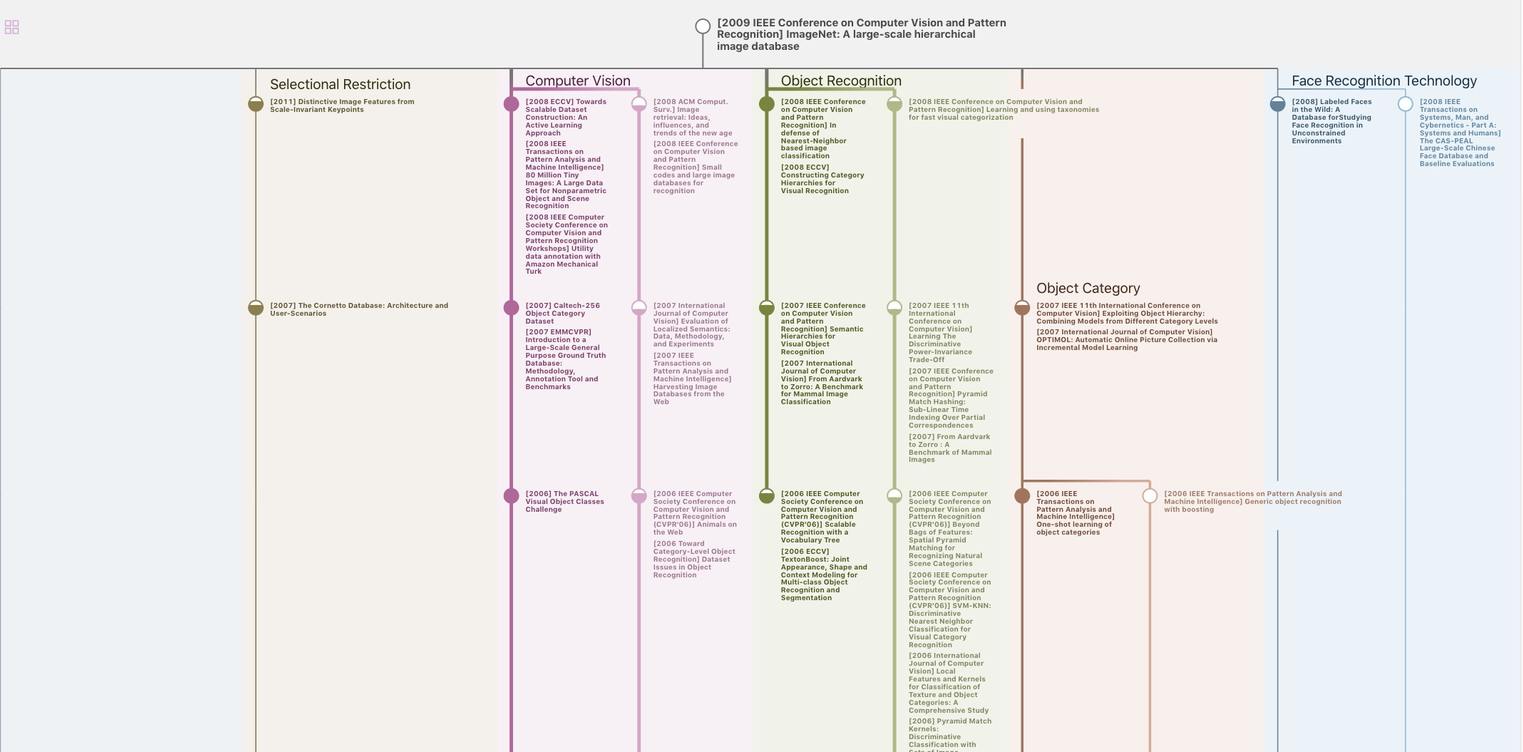
生成溯源树,研究论文发展脉络
Chat Paper
正在生成论文摘要