An adaptive sampling augmented Lagrangian method for stochastic optimization with deterministic constraints
COMPUTERS & MATHEMATICS WITH APPLICATIONS(2023)
摘要
The primary goal of this paper is to provide an efficient solution algorithm based on the augmented Lagrangian framework for optimization problems with a stochastic objective function and deterministic constraints. Our main contribution is combining the augmented Lagrangian framework with adaptive sampling, resulting in an efficient optimization methodology validated with practical examples. To achieve the presented efficiency, we consider inexact solutions for the augmented Lagrangian subproblems, and through an adaptive sampling mechanism, we control the variance in the gradient estimates. Furthermore, we analyze the theoretical performance of the proposed scheme by showing equivalence to a gradient descent algorithm on a Moreau envelope function, and we prove sublinear convergence for convex objectives and linear convergence for strongly convex objectives with affine equality constraints. The worst-case sample complexity of the resulting algorithm, for an arbitrary choice of penalty parameter in the augmented Lagrangian function, is O(epsilon(-3-delta)), where epsilon > 0 is the expected error of the solution and delta > 0 is a user-defined parameter. If the penalty parameter is chosen to be O(epsilon(-1)), we demonstrate that the result can be improved to O(epsilon(-2)), which is competitive with the other methods employed in the literature. Moreover, if the objective function is strongly convex with affine equality constraints, we obtain O(epsilon(-1)log(1/epsilon)) complexity. Finally, we empirically verify the performance of our adaptive sampling augmented Lagrangian framework in machine learning optimization and engineering design problems, including topology optimization of a heat sink with environmental uncertainty.
更多查看译文
关键词
Augmented Lagrangian,Stochastic optimization,Adaptive sampling,Constrained optimization,Engineering design,Machine learning
AI 理解论文
溯源树
样例
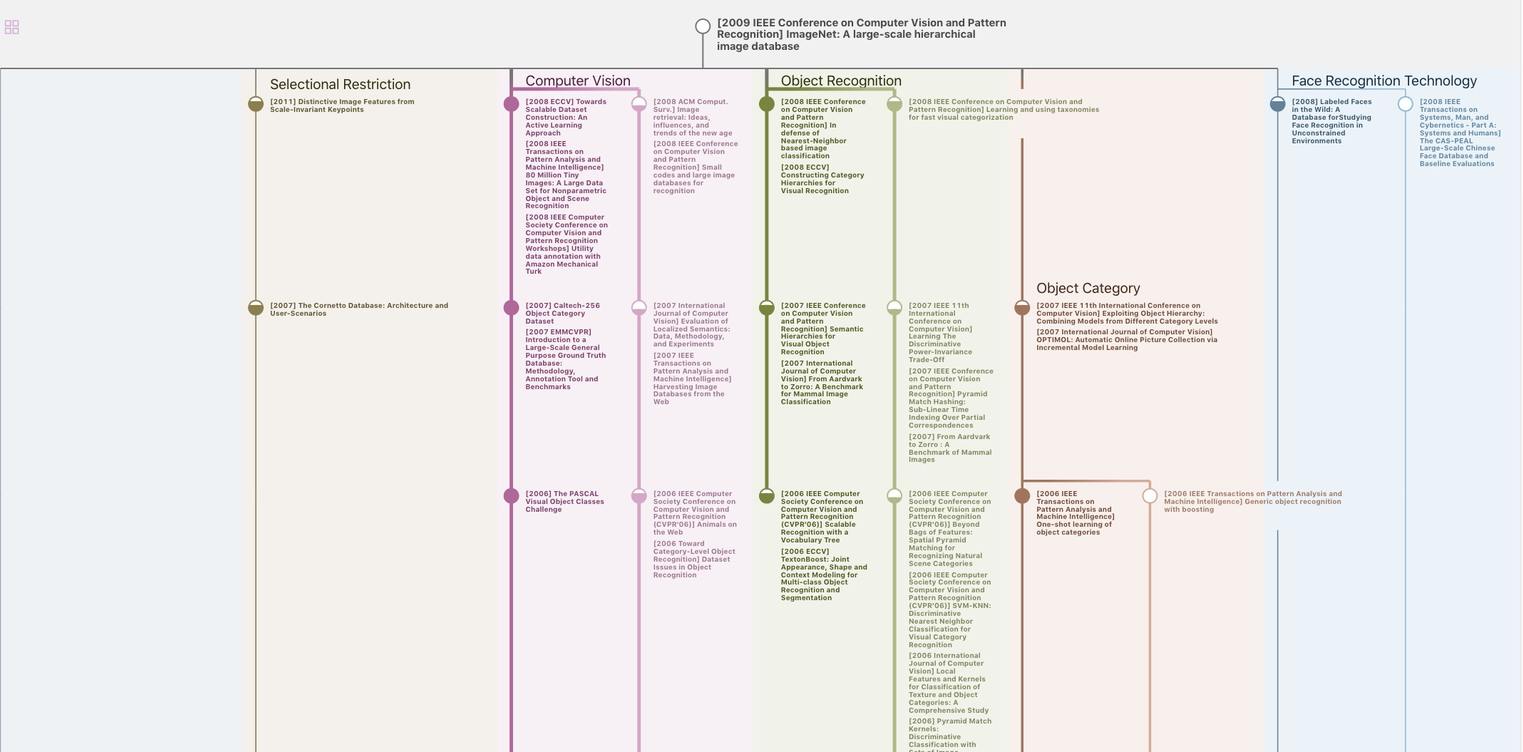
生成溯源树,研究论文发展脉络
Chat Paper
正在生成论文摘要