Discover and Cure: Concept-aware Mitigation of Spurious Correlation
ICML 2023(2023)
摘要
Deep neural networks often rely on spurious correlations to make predictions, which hinders generalization beyond training environments. For instance, models that associate cats with bed backgrounds can fail to predict the existence of cats in other environments without beds. Mitigating spurious correlations is crucial in building trustworthy models. However, the existing works lack transparency to offer insights into the mitigation process. In this work, we propose an interpretable framework, Discover and Cure (DISC), to tackle the issue. With human-interpretable concepts, DISC iteratively 1) discovers unstable concepts across different environments as spurious attributes, then 2) intervenes on the training data using the discovered concepts to reduce spurious correlation. Across systematic experiments, DISC provides superior generalization ability and interpretability than the existing approaches. Specifically, it outperforms the state-of-the-art methods on an object recognition task and a skin-lesion classification task by 7.5% and 9.6%, respectively. Additionally, we offer theoretical analysis and guarantees to understand the benefits of models trained by DISC. Code and data are available at https://github.com/Wuyxin/DISC.
更多查看译文
关键词
correlation,mitigation,concept-aware
AI 理解论文
溯源树
样例
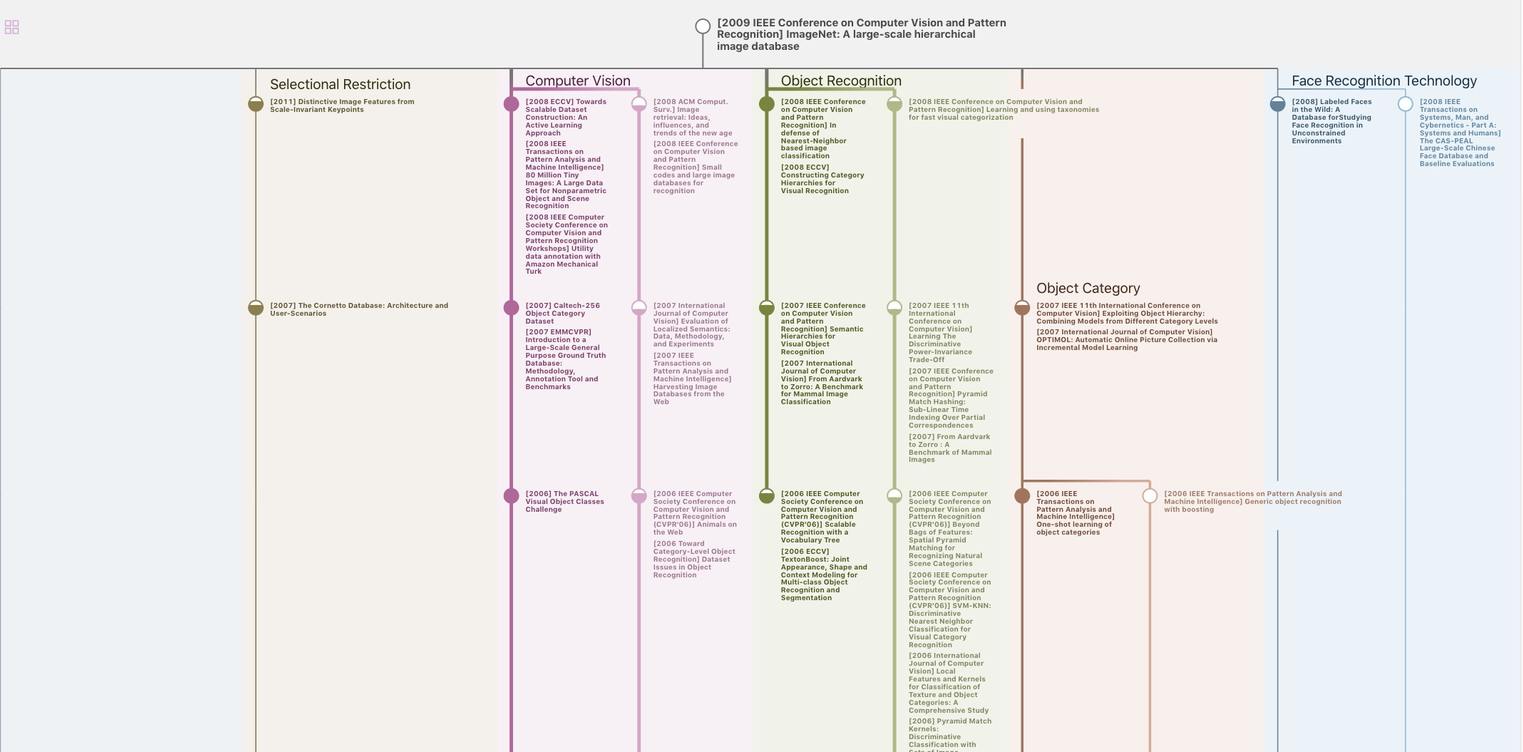
生成溯源树,研究论文发展脉络
Chat Paper
正在生成论文摘要