DualHSIC: HSIC-Bottleneck and Alignment for Continual Learning
CoRR(2023)
摘要
Rehearsal-based approaches are a mainstay of continual learning (CL). They mitigate the catastrophic forgetting problem by maintaining a small fixed-size buffer with a subset of data from past tasks. While most rehearsal-based approaches study how to effectively exploit the knowledge from the buffered past data, little attention is paid to the inter-task relationships with the critical task-specific and task-invariant knowledge. By appropriately leveraging inter-task relationships, we propose a novel CL method named DualHSIC to boost the performance of existing rehearsal-based methods in a simple yet effective way. DualHSIC consists of two complementary components that stem from the so-called Hilbert Schmidt independence criterion (HSIC): HSIC-Bottleneck for Rehearsal (HBR) lessens the inter-task interference and HSIC Alignment (HA) promotes task-invariant knowledge sharing. Extensive experiments show that DualHSIC can be seamlessly plugged into existing rehearsal-based methods for consistent performance improvements, and also outperforms recent state-of-the-art regularization-enhanced rehearsal methods. Source code will be released.
更多查看译文
关键词
learning,alignment,hsic-bottleneck
AI 理解论文
溯源树
样例
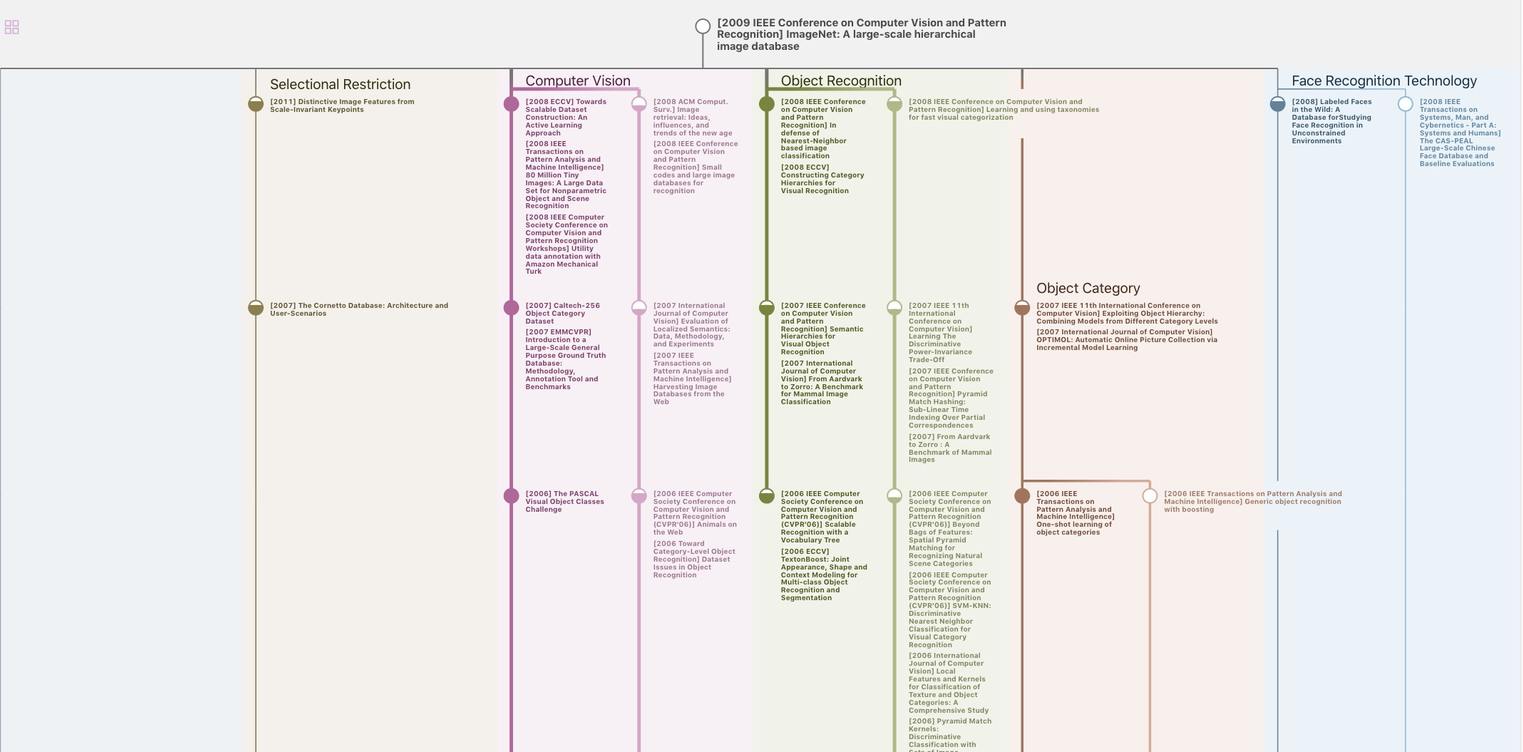
生成溯源树,研究论文发展脉络
Chat Paper
正在生成论文摘要