S2abEL: A Dataset for Entity Linking from Scientific Tables.
CoRR(2023)
摘要
Entity linking (EL) is the task of linking a textual mention to its corresponding entry in a knowledge base, and is critical for many knowledge-intensive NLP applications. When applied to tables in scientific papers, EL is a step toward large-scale scientific knowledge bases that could enable advanced scientific question answering and analytics. We present the first dataset for EL in scientific tables. EL for scientific tables is especially challenging because scientific knowledge bases can be very incomplete, and disambiguating table mentions typically requires understanding the papers's tet in addition to the table. Our dataset, S2abEL, focuses on EL in machine learning results tables and includes hand-labeled cell types, attributed sources, and entity links from the PaperswithCode taxonomy for 8,429 cells from 732 tables. We introduce a neural baseline method designed for EL on scientific tables containing many out-of-knowledge-base mentions, and show that it significantly outperforms a state-of-the-art generic table EL method. The best baselines fall below human performance, and our analysis highlights avenues for improvement.
更多查看译文
关键词
entity linking,scientific tables,dataset
AI 理解论文
溯源树
样例
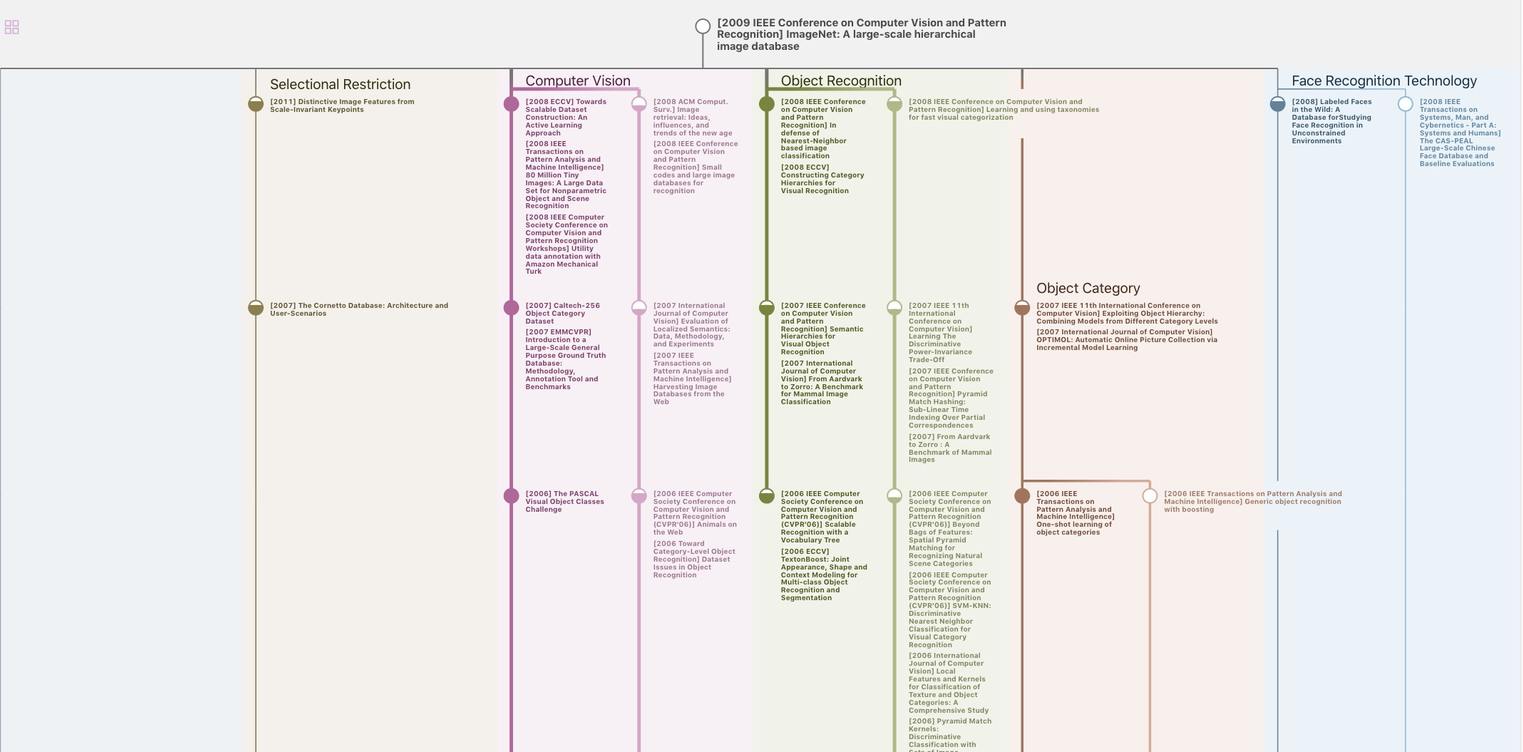
生成溯源树,研究论文发展脉络
Chat Paper
正在生成论文摘要