The eROSITA Final Equatorial-Depth Survey (eFEDS): A Machine Learning Approach to Infer Galaxy Cluster Masses from eROSITA X-ray Images
arXiv (Cornell University)(2023)
摘要
We develop a neural network based pipeline to estimate masses of galaxy clusters with a known redshift directly from photon information in X-rays. Our neural networks are trained using supervised learning on simulations of eROSITA observations, focusing in this paper on the Final Equatorial Depth Survey (eFEDS). We use convolutional neural networks which are modified to include additional information of the cluster, in particular its redshift. In contrast to existing work, we utilize simulations including background and point sources to develop a tool which is usable directly on observational eROSITA data for an extended mass range from group size halos to massive clusters with masses in between $10^{13}M_\odot更多
查看译文
关键词
infer galaxy cluster masses,erosita,equatorial-depth,x-ray
AI 理解论文
溯源树
样例
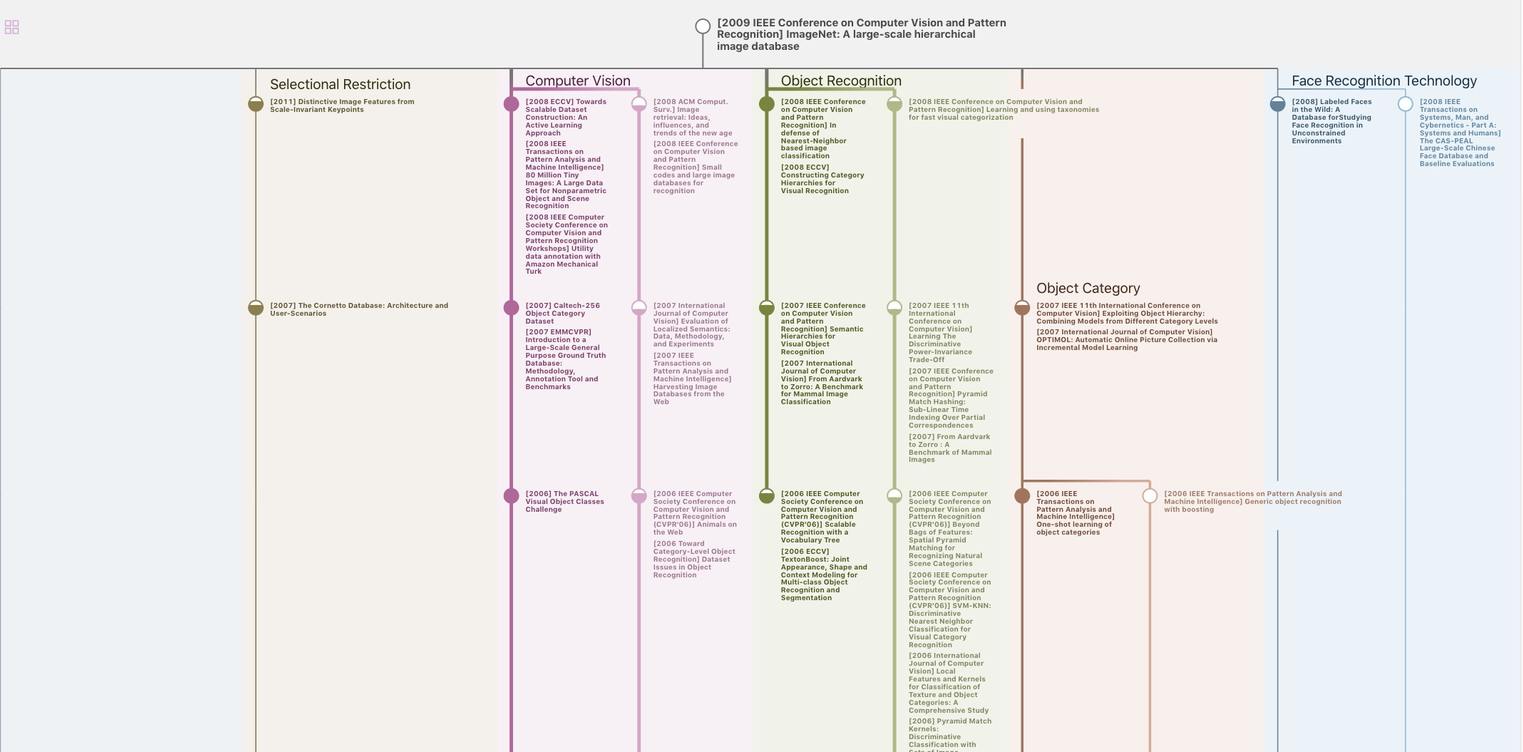
生成溯源树,研究论文发展脉络
Chat Paper
正在生成论文摘要