Made of Steel? Learning Plausible Materials for Components in the Vehicle Repair Domain
17TH CONFERENCE OF THE EUROPEAN CHAPTER OF THE ASSOCIATION FOR COMPUTATIONAL LINGUISTICS, EACL 2023(2023)
摘要
We propose a novel approach to learn domain-specific plausible materials for components in the vehicle repair domain by probing Pre-trained Language Models (PLMs) in a cloze task style setting to overcome the lack of annotated datasets. We devise a new method to aggregate salient predictions from a set of cloze query templates and show that domain-adaptation using either a small, high-quality or a customized Wikipedia corpus boosts performance. When exploring resource-lean alternatives, we find a distilled PLM clearly outperforming a classic pattern-based algorithm. Further, given that 98% of our domain-specific components are multiword expressions, we successfully exploit the compositionality assumption as a way to address data sparsity.
更多查看译文
AI 理解论文
溯源树
样例
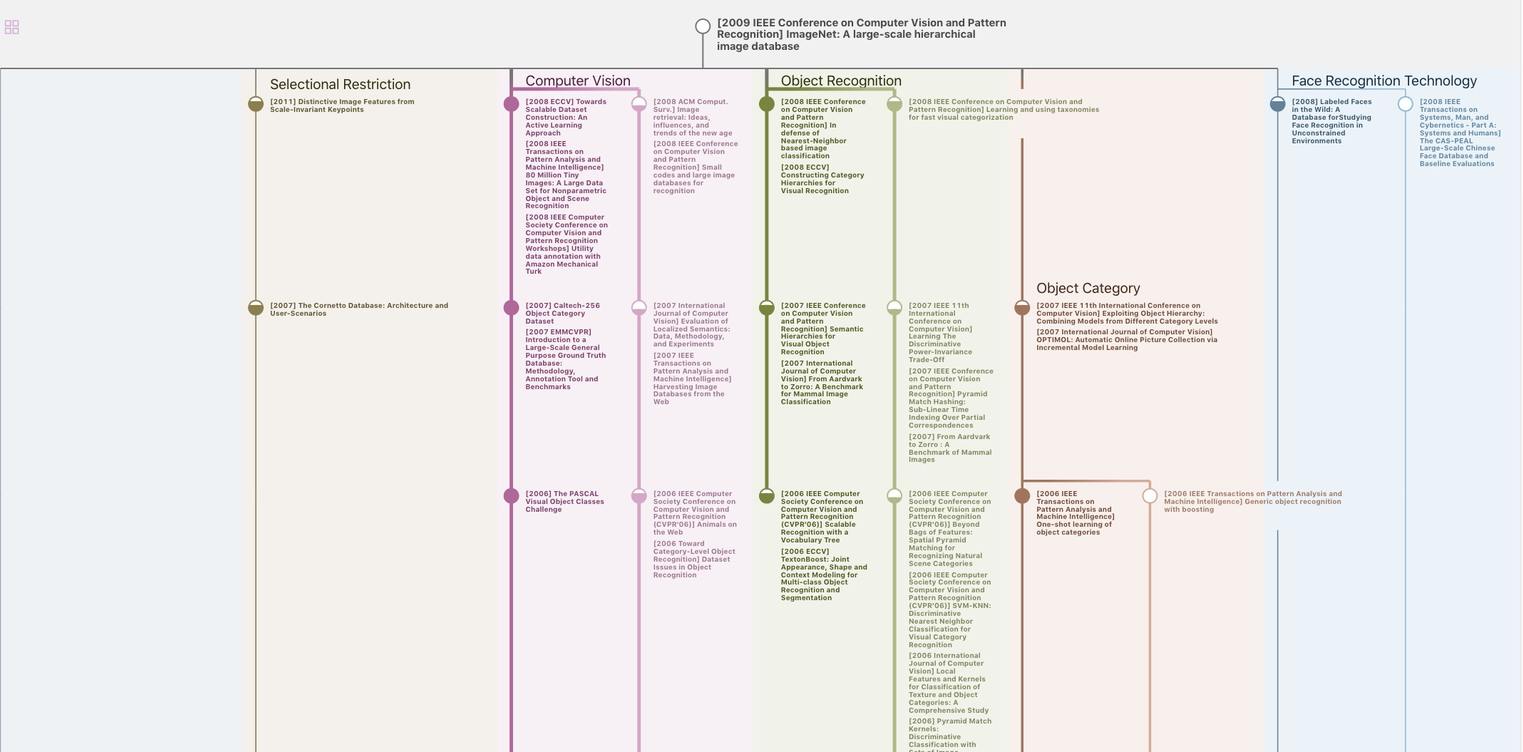
生成溯源树,研究论文发展脉络
Chat Paper
正在生成论文摘要