Meta-path reasoning of knowledge graph for commonsense question answering.
Frontiers Comput. Sci.(2024)
摘要
Commonsense question answering (CQA) requires understanding and reasoning over QA context and related commonsense knowledge, such as a structured Knowledge Graph (KG). Existing studies combine language models and graph neural networks to model inference. However, traditional knowledge graph are mostly concept-based, ignoring direct path evidence necessary for accurate reasoning. In this paper, we propose MRGNN (Meta-path Reasoning Graph Neural Network), a novel model that comprehensively captures sequential semantic information from concepts and paths. In MRGNN, meta-paths are introduced as direct inference evidence and an original graph neural network is adopted to aggregate features from both concepts and paths simultaneously. We conduct sufficient experiments on the CommonsenceQA and OpenBookQA datasets, showing the effectiveness of MRGNN. Also, we conduct further ablation experiments and explain the reasoning behavior through the case study.
更多查看译文
关键词
question answering,knowledge graph,graph neural network,meta-path reasoning
AI 理解论文
溯源树
样例
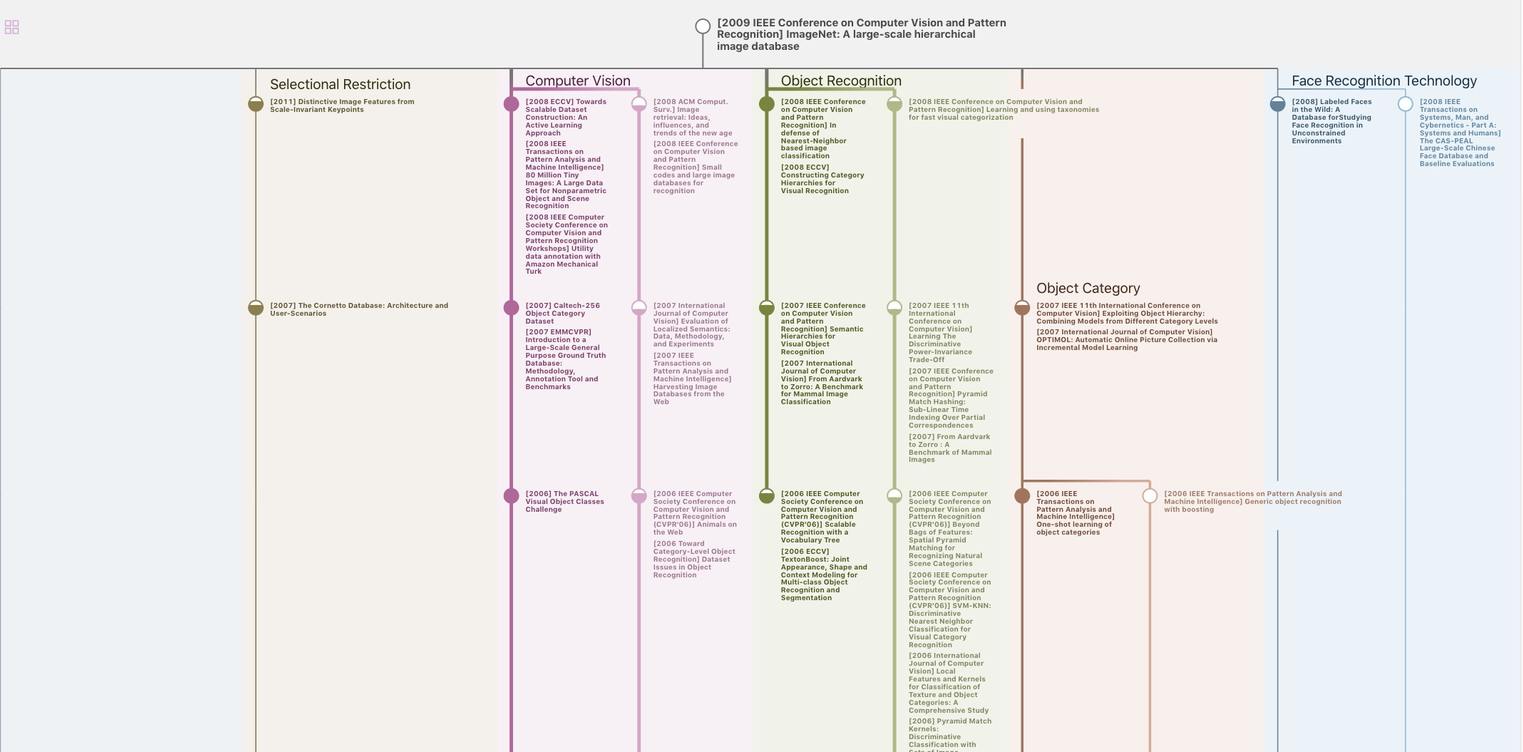
生成溯源树,研究论文发展脉络
Chat Paper
正在生成论文摘要