Dual-feature Fusion Attention Network for Small Object Segmentation
Computers in Biology and Medicine(2023)
摘要
Accurate segmentation of medical images is an important step during radiotherapy planning and clinical diagnosis. However, manually marking organ or lesion boundaries is tedious, time-consuming, and prone to error due to subjective variability of radiologist. Automatic segmentation remains a challenging task owing to the variation (in shape and size) across subjects. Moreover, existing convolutional neural networks based methods perform poorly in small medical objects segmentation due to class imbalance and boundary ambiguity. In this paper, we propose a dual feature fusion attention network (DFF-Net) to improve the segmentation accuracy of small objects. It mainly includes two core modules: the dual-branch feature fusion module (DFFM) and the reverse attention context module (RACM). We first extract multi-resolution features by multi-scale feature extractor, then construct DFFM to aggregate the global and local contextual information to achieve information complementarity among features, which provides sufficient guidance for accurate small objects segmentation. Moreover, to alleviate the degradation of segmentation accuracy caused by blurred medical image boundaries, we propose RACM to enhance the edge texture of features. Experimental results on datasets NPC, ACDC, and Polyp demonstrate that our proposed method has fewer parameters, faster inference, and lower model complexity, and achieves better accuracy than more state-of-the-art methods.
更多查看译文
关键词
Small object segmentation, Dual-branch feature fusion, Reverse attention, Multi-resolution features
AI 理解论文
溯源树
样例
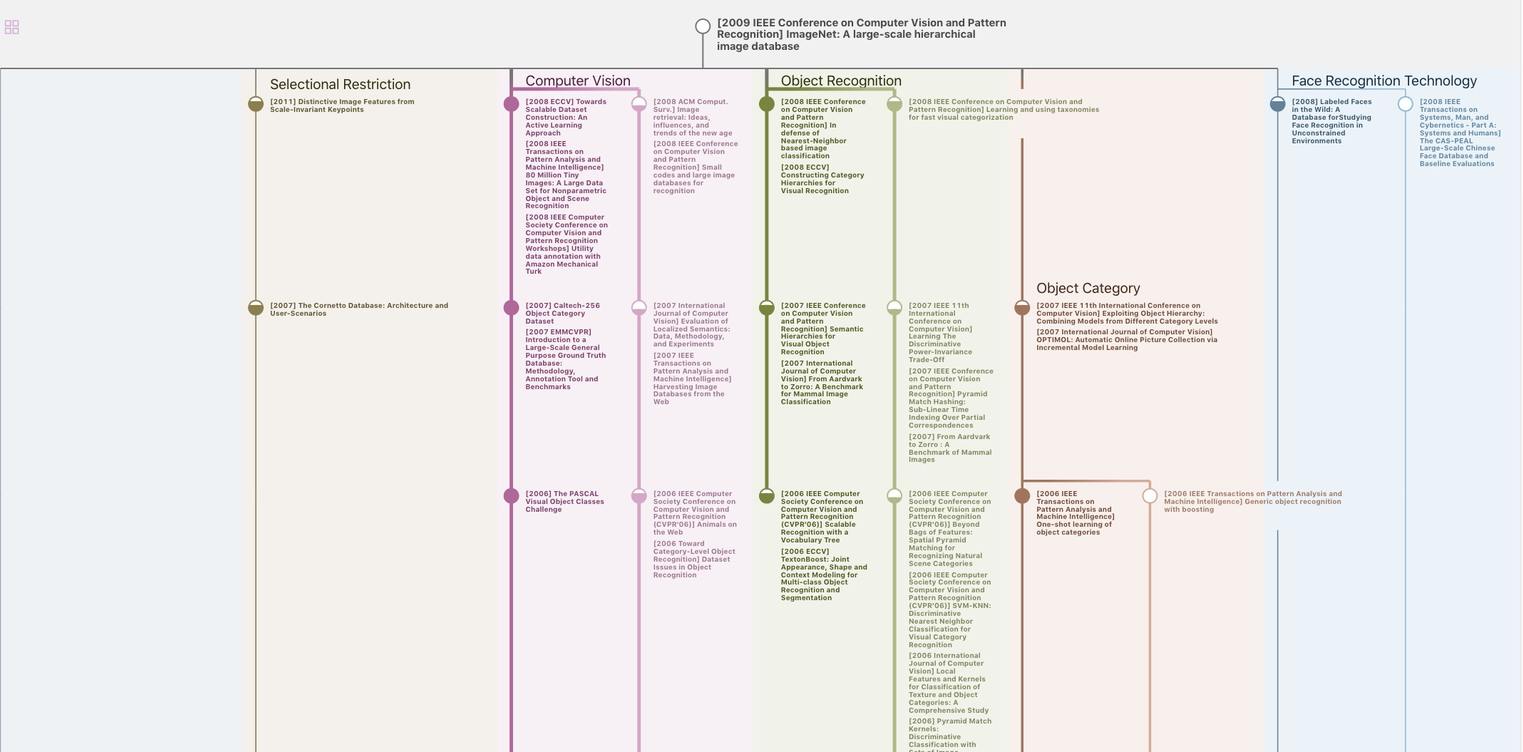
生成溯源树,研究论文发展脉络
Chat Paper
正在生成论文摘要