Multi-Granularity Context Network for Efficient Video Semantic Segmentation
IEEE transactions on image processing : a publication of the IEEE Signal Processing Society(2023)
摘要
Current video semantic segmentation tasks involve two main challenges: how to take full advantage of multi-frame context information, and how to improve computational efficiency. To tackle the two challenges simultaneously, we present a novel Multi-Granularity Context Network (MGCNet) by aggregating context information at multiple granularities in a more effective and efficient way. Our method first converts image features into semantic prototypes, and then conducts a non-local operation to aggregate the per-frame and short-term contexts jointly. An additional long-term context module is introduced to capture the video-level semantic information during training. By aggregating both local and global semantic information, a strong feature representation is obtained. The proposed pixel-to-prototype non-local operation requires less computational cost than traditional non-local ones, and is video-friendly since it reuses the semantic prototypes of previous frames. Moreover, we propose an uncertainty-aware and structural knowledge distillation strategy to boost the performance of our method. Experiments on Cityscapes and CamVid datasets with multiple backbones demonstrate that the proposed MGCNet outperforms other state-of-the-art methods with high speed and low latency.
更多查看译文
关键词
Semantics,Semantic segmentation,Prototypes,Aggregates,Feature extraction,Training,Task analysis,Video semantic segmentation,light-weight networks,non-local operation
AI 理解论文
溯源树
样例
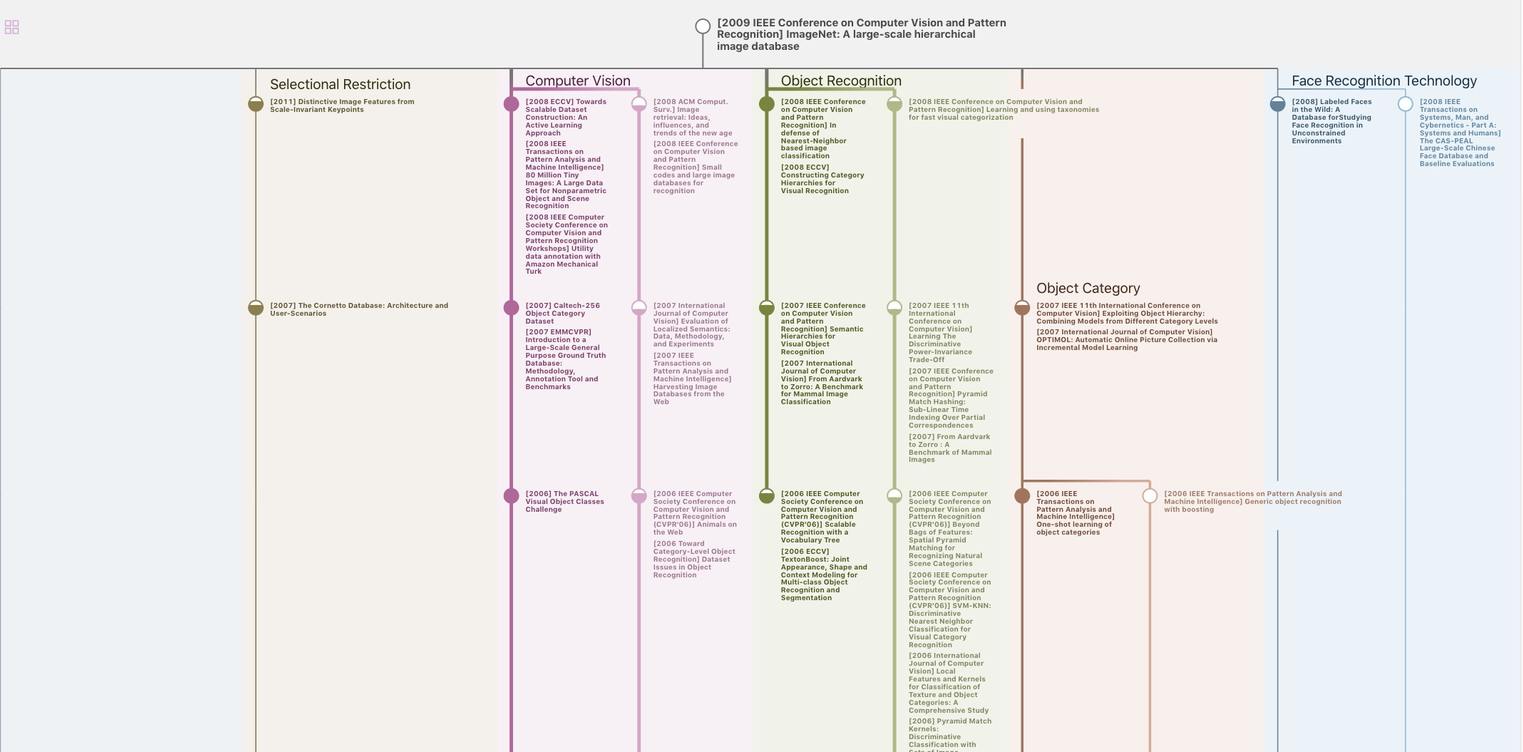
生成溯源树,研究论文发展脉络
Chat Paper
正在生成论文摘要