Privacy preserving federated learning for full heterogeneity.
ISA transactions(2023)
摘要
Federated learning is a novel distribute machine learning paradigm to support cooperative model training among multiple participant clients, where each client keeps its private data locally to protect its data privacy. However, in practical application domains, Federated learning still meets several heterogeneous challenges such data heterogeneity, model heterogeneity, and computation heterogeneity, significantly decreasing its global model performance. To the best of our knowledge, existing solutions only focus on one or two challenges in their heterogeneous settings. In this paper, to address the above challenges simultaneously, we present a novel solution called Full Heterogeneous Federated Learning (FHFL). Firstly, we propose a synthetic data generation approach to mitigate the Non-IID data heterogeneity problem. Secondly, we use knowledge distillation to learn from heterogeneous models of participant clients for model aggregation in the central server. Finally, we produce an opportunistic computation schedule strategy to exploit the idle computation resources for fast-computing clients. Experiment results on different datasets show that our FHFL method can achieve an excellent model training performance. We believe it will serve as a pioneer work for distributed model training among heterogeneous clients in Federated learning.
更多查看译文
AI 理解论文
溯源树
样例
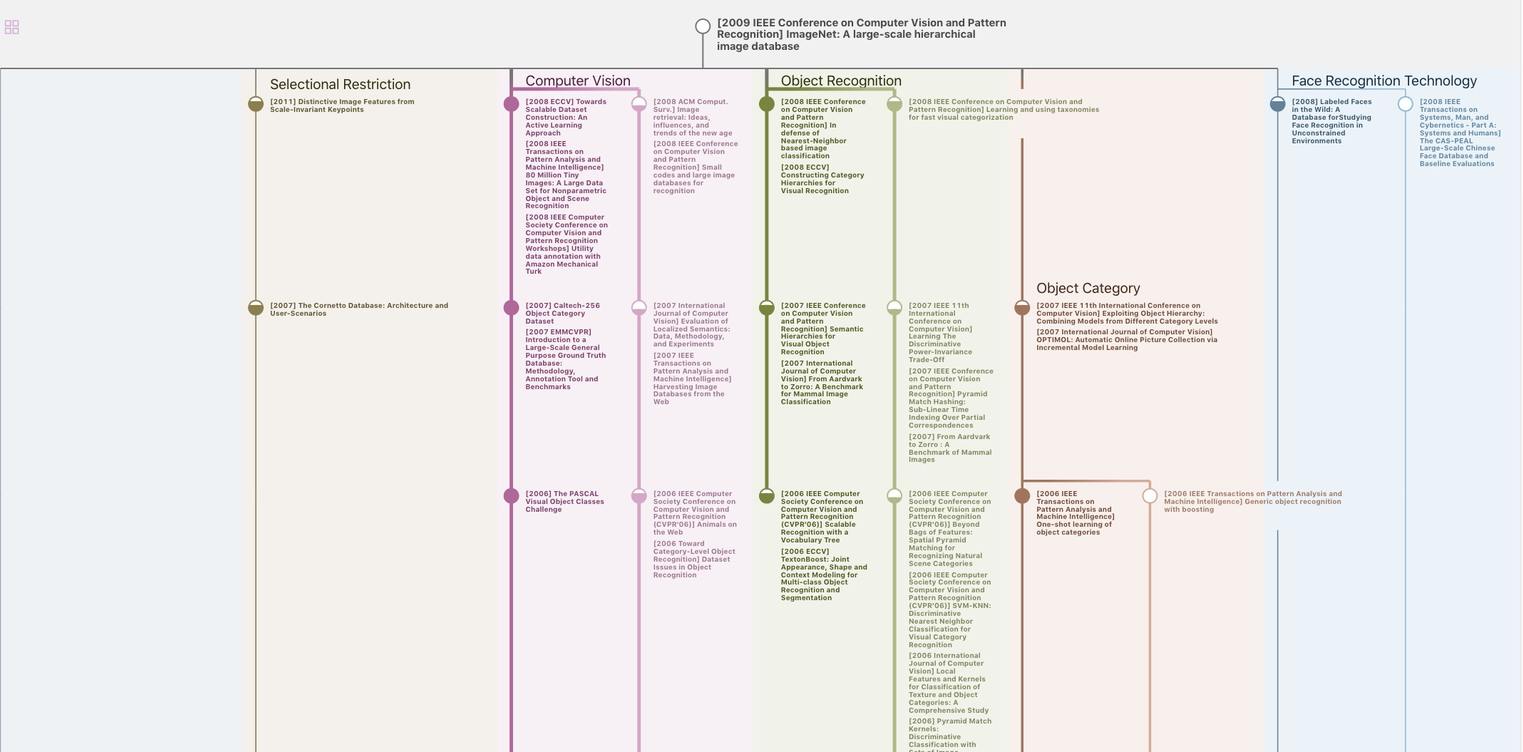
生成溯源树,研究论文发展脉络
Chat Paper
正在生成论文摘要