Data Representation via Attribute Selection- Propagation Neural Network
IEEE Transactions on Signal and Information Processing over Networks(2023)
摘要
Graph Convolutional Networks (GCNs) have been commonly studied for attribute graph data representation. It is known that the core of Graph Convolution (GC) operation is to define a specific graph propagation operation for graph node's attributes. Existing GCs mainly perform propagation over node's all attributes. However, this ‘full’ propagation may be unreasonable on some practical learning tasks and also be vulnerable to the noisy attributes. To address this issue, in this article, we propose a novel Attribute Selection-Propagation (ASP) mechanism for attribute graph data representation by incorporating attribute selection into GCs. The main aspect of the proposed ASP is that it can be formulated as a
regularization model
based on which we can derive a simple update rule to implement ASP in a
self-supervised
manner. ASP aims to adaptively propagate some optimal attributes of node to better serve message passing in GCs. Using ASP, we then present a novel graph neural network, named ASPNet for attribute graph representation and learning. Experiments on several graph learning tasks including node classification, clustering and link prediction demonstrate the effectiveness of the proposed ASPNet.
更多查看译文
关键词
Graph convolutional networks,full propagation,attribute selection-propagation,graph learning tasks
AI 理解论文
溯源树
样例
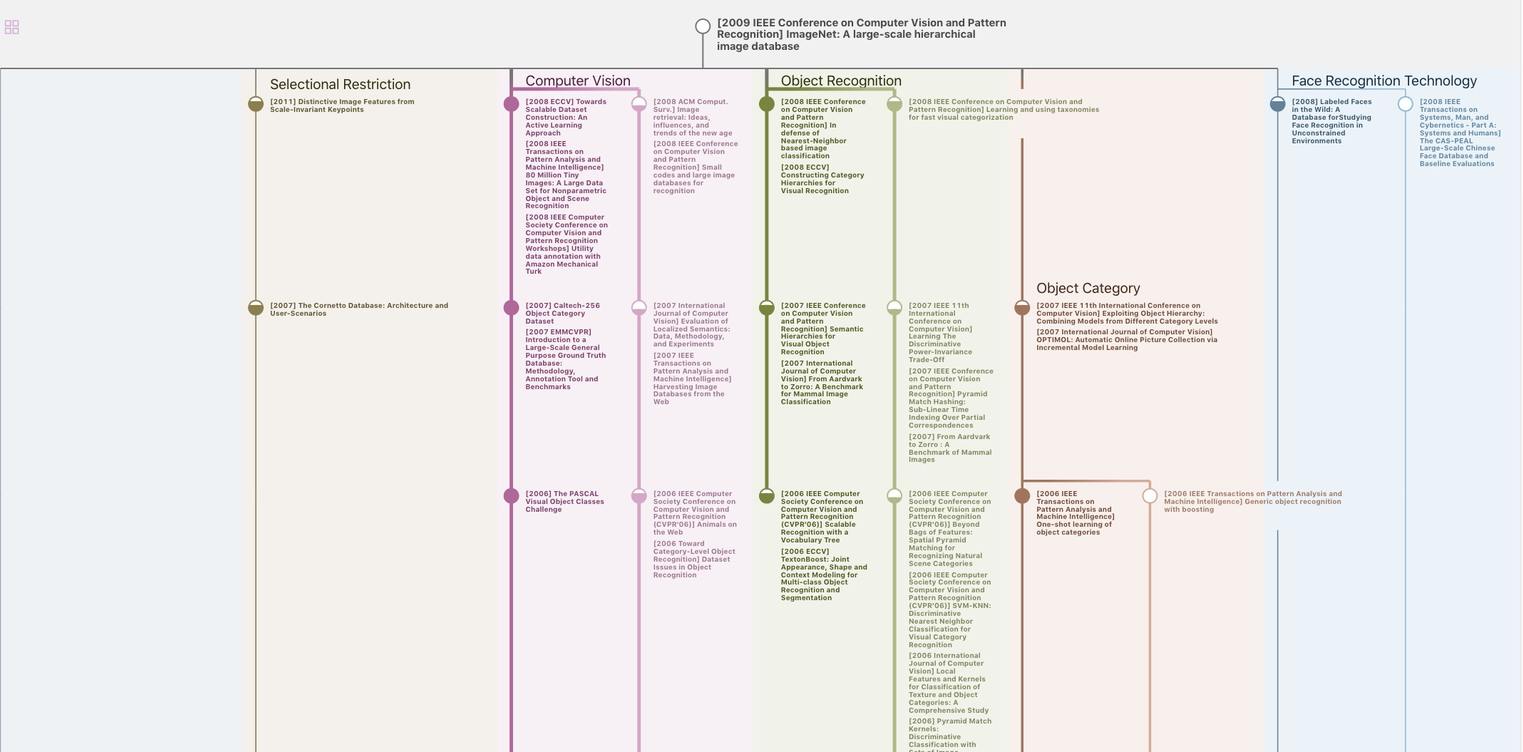
生成溯源树,研究论文发展脉络
Chat Paper
正在生成论文摘要