Dose prediction of organs at risk in patients with cervical cancer receiving brachytherapy using needle insertion based on a neural network method
BMC Cancer(2023)
摘要
Objective A neural network method was employed to establish a dose prediction model for organs at risk (OAR) in patients with cervical cancer receiving brachytherapy using needle insertion. Methods A total of 218 CT-based needle-insertion brachytherapy fraction plans for loco-regionally advanced cervical cancer treatment were analyzed in 59 patients. The sub-organ of OAR was automatically generated by self-written MATLAB, and the volume of the sub-organ was read. Correlations between D2cm 3 of each OAR and volume of each sub-organ—as well as high-risk clinical target volume for bladder, rectum, and sigmoid colon—were analyzed. We then established a neural network predictive model of D2cm 3 of OAR using the matrix laboratory neural net. Of these plans, 70% were selected as the training set, 15% as the validation set, and 15% as the test set. The regression R value and mean squared error were subsequently used to evaluate the predictive model. Results The D2cm 3 /D90 of each OAR was related to volume of each respective sub-organ. The R values for bladder, rectum, and sigmoid colon in the training set for the predictive model were 0.80513, 0.93421, and 0.95978, respectively. The ∆D2cm 3 /D90 for bladder, rectum, and sigmoid colon in all sets was 0.052 ± 0.044, 0.040 ± 0.032, and 0.041 ± 0.037, respectively. The MSE for bladder, rectum, and sigmoid colon in the training set for the predictive model was 4.779 × 10 −3 , 1.967 × 10 −3 and 1.574 × 10 −3 , respectively. Conclusion The neural network method based on a dose-prediction model of OAR in brachytherapy using needle insertion was simple and reliable. In addition, it only addressed volumes of sub-organs to predict the dose of OAR, which we believe is worthy of further promotion and application.
更多查看译文
关键词
Brachytherapy,Dose prediction,Needle insertion,Neural network
AI 理解论文
溯源树
样例
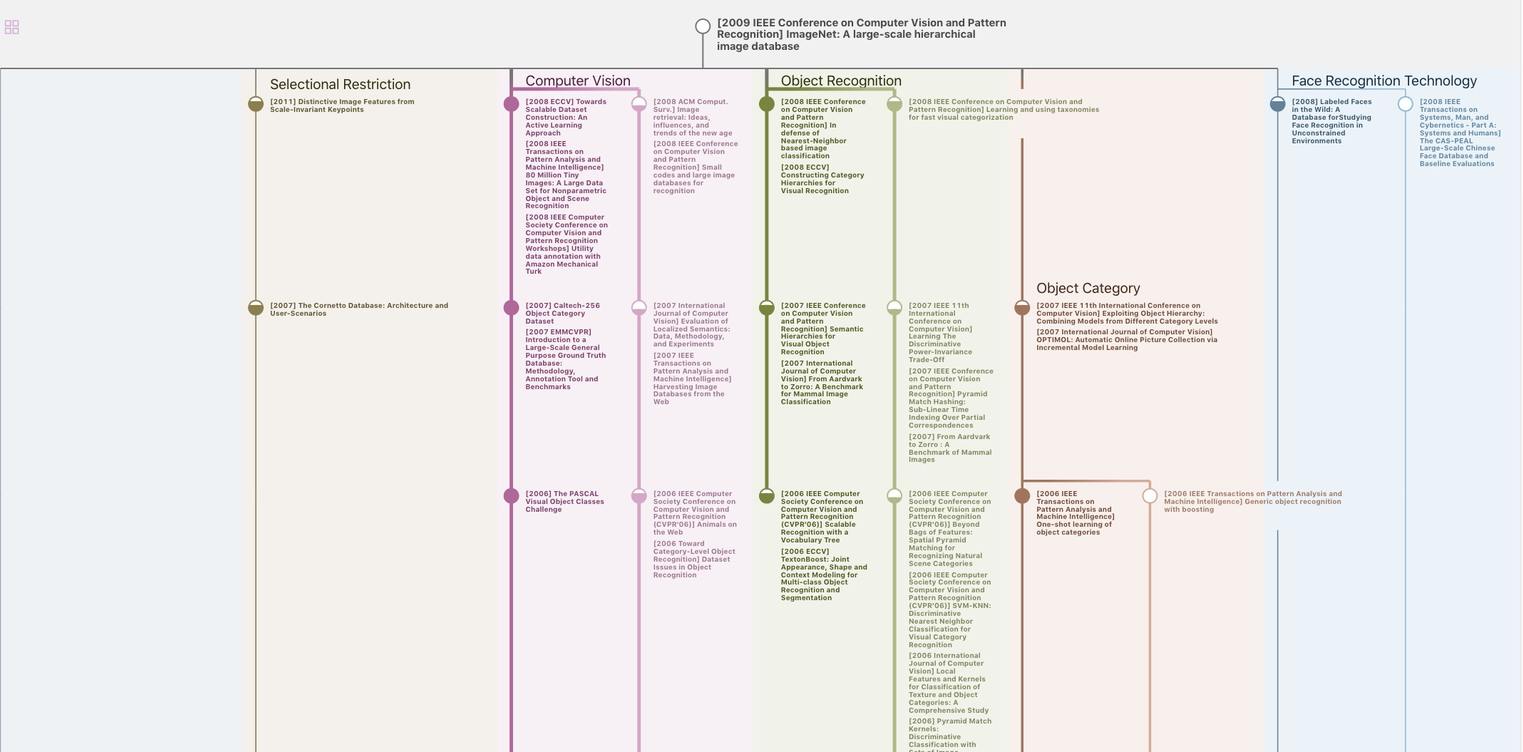
生成溯源树,研究论文发展脉络
Chat Paper
正在生成论文摘要