Domain-Invariant Information Aggregation for Domain Generalization Semantic Segmentation
Neurocomputing(2023)
摘要
Domain generalization semantic segmentation methods aim to generalize well on out-of-distribution scenes, which is crucial for real-world applications. Recent works focus on learning domain-invariant content information by using normalization, whitening, and domain randomization to remove style information. Although these methods improve the performance on out-of-distribution scenes to some extent, they ignore the learning of edge and semantic layout information. The edge information describes the shape and boundary of an object and the semantic layout information contains the common sense priors (e.g., the spatial position of objects). For one thing, we observe that the shape of the same object with different styles is domain-invariant in the edge map. For another, we observe that the common sense priors in the semantic layout information of different scenes are domain-invariant. Motivated by these observations, a novel approach is proposed for domain generalization semantic segmentation by using the edge and semantic layout information. Specifically, the proposed approach contains the edge reconstruction module (ERM), the semantic layout reconstruction module (SLRM), and the triple information aggregation module (TIAM). The ERM and SLRM aim to explicitly learn the edge and semantic layout information. The TIAM aggregates the edge and semantic layout information to refine the content information. Extensive experiments demonstrate that our approach achieves superior performance over current approaches on domain generalization segmentation tasks. The source code will be released at https://github.com/seabearlmx/DIIA.
更多查看译文
关键词
domain-invariant generalization,segmentation,aggregation
AI 理解论文
溯源树
样例
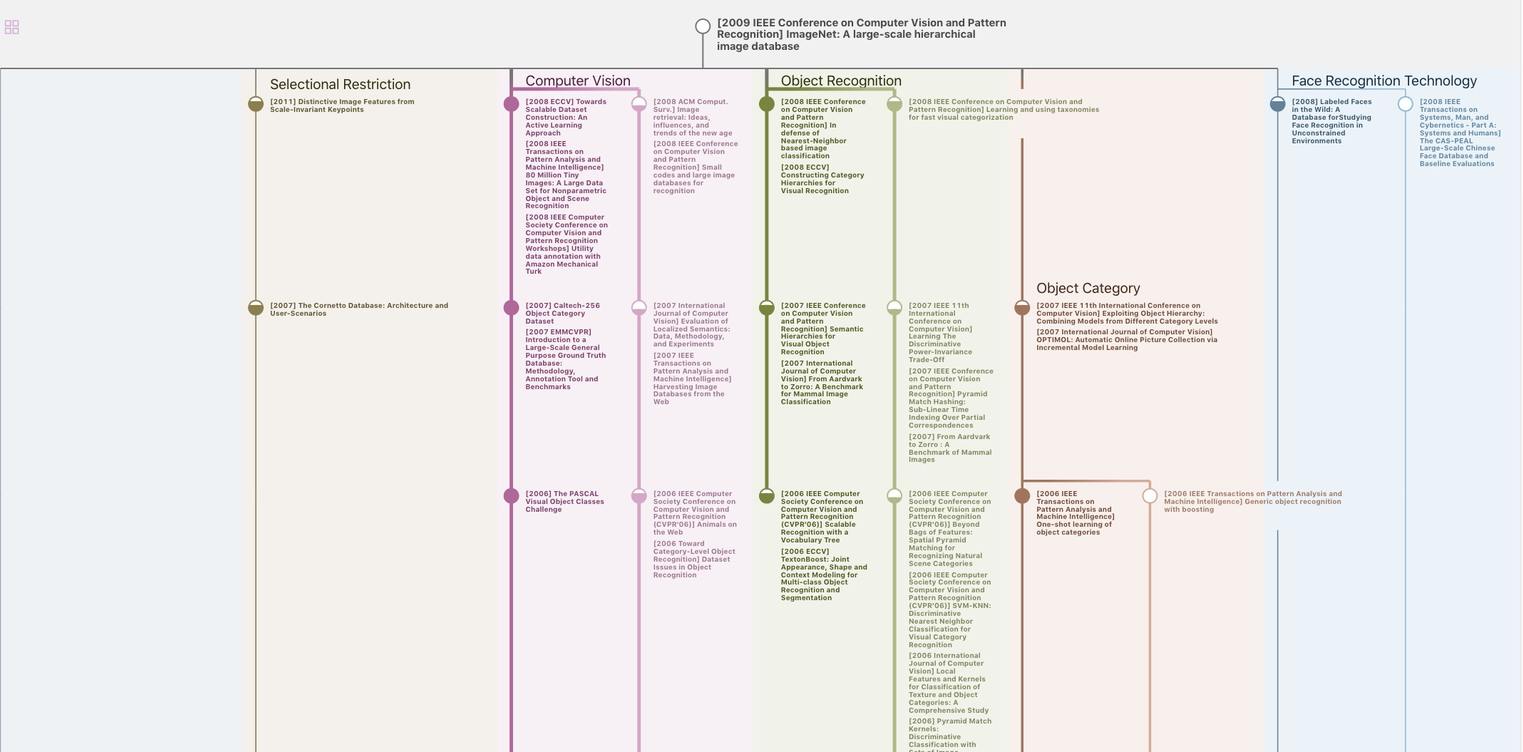
生成溯源树,研究论文发展脉络
Chat Paper
正在生成论文摘要