Pseudo-Hamiltonian neural networks for learning partial differential equations
JOURNAL OF COMPUTATIONAL PHYSICS(2024)
摘要
Pseudo-Hamiltonian neural networks (PHNN) were recently introduced for learning dynamical systems that can be modelled by ordinary differential equations. In this paper, we extend the method to partial differential equations. The resulting model is comprised of up to three neural networks, modelling terms representing conservation, dissipation and external forces, and discrete convolution operators that can either be learned or be given as input. We demonstrate numerically the superior performance of PHNN compared to a baseline model that models the full dynamics by a single neural network. Moreover, since the PHNN model consists of three parts with different physical interpretations, these can be studied separately to gain insight into the system, and the learned model is applicable also if external forces are removed or changed.
更多查看译文
关键词
Physics-informed machine learning,Hamiltonian neural networks,Partial differential equations,Inverse problem
AI 理解论文
溯源树
样例
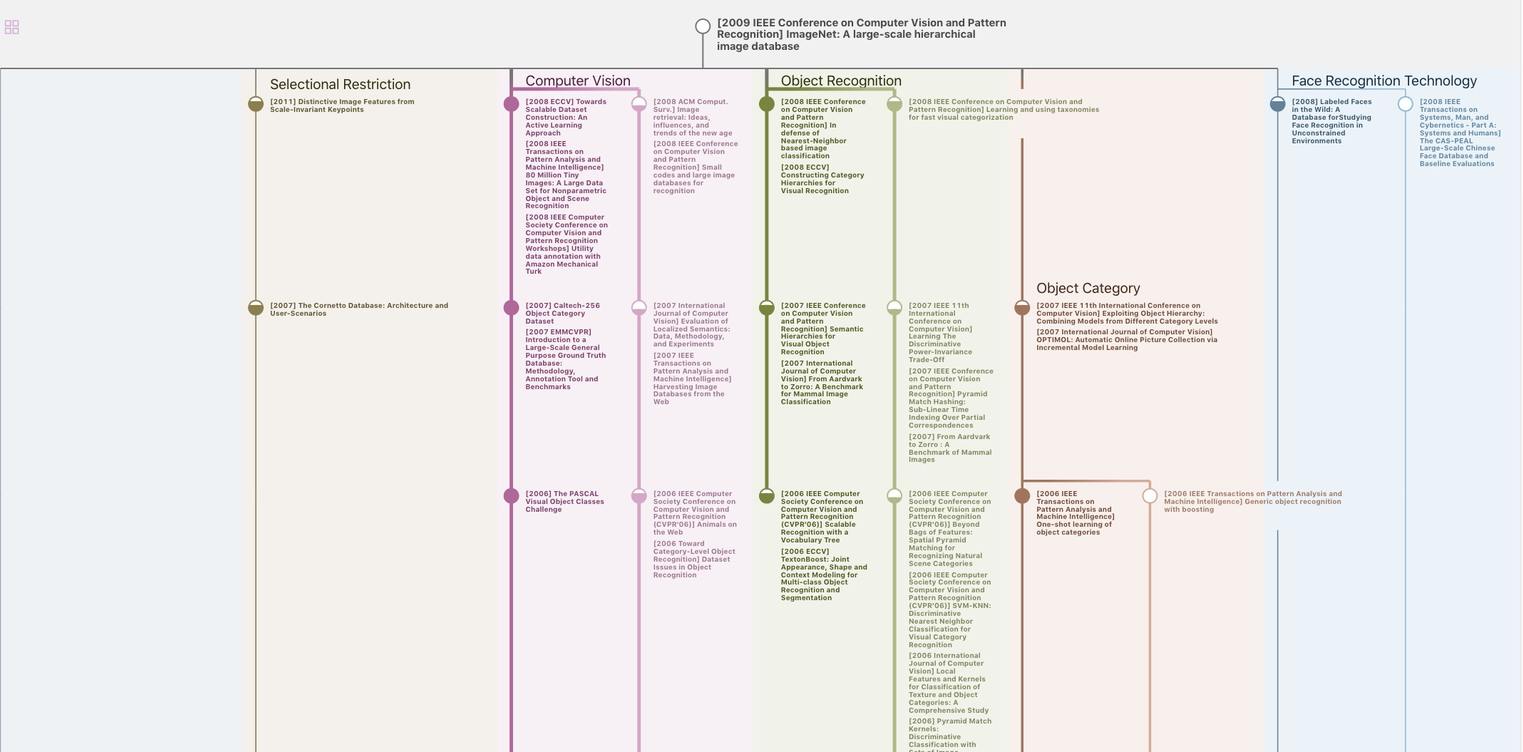
生成溯源树,研究论文发展脉络
Chat Paper
正在生成论文摘要