Propagating Kernel Ambiguity Sets in Nonlinear Data-driven Dynamics Models
CoRR(2023)
摘要
This paper provides answers to an open problem: given a nonlinear data-driven dynamical system model, e.g., kernel conditional mean embedding (CME) and Koopman operator, how can one propagate the ambiguity sets forward for multiple steps? This problem is the key to solving distributionally robust control and learning-based control of such learned system models under a data-distribution shift. Different from previous works that use either static ambiguity sets, e.g., fixed Wasserstein balls, or dynamic ambiguity sets under known piece-wise linear (or affine) dynamics, we propose an algorithm that exactly propagates ambiguity sets through nonlinear data-driven models using the Koopman operator and CME, via the kernel maximum mean discrepancy geometry. Through both theoretical and numerical analysis, we show that our kernel ambiguity sets are the natural geometric structure for the learned data-driven dynamical system models.
更多查看译文
关键词
kernel ambiguity sets,dynamics
AI 理解论文
溯源树
样例
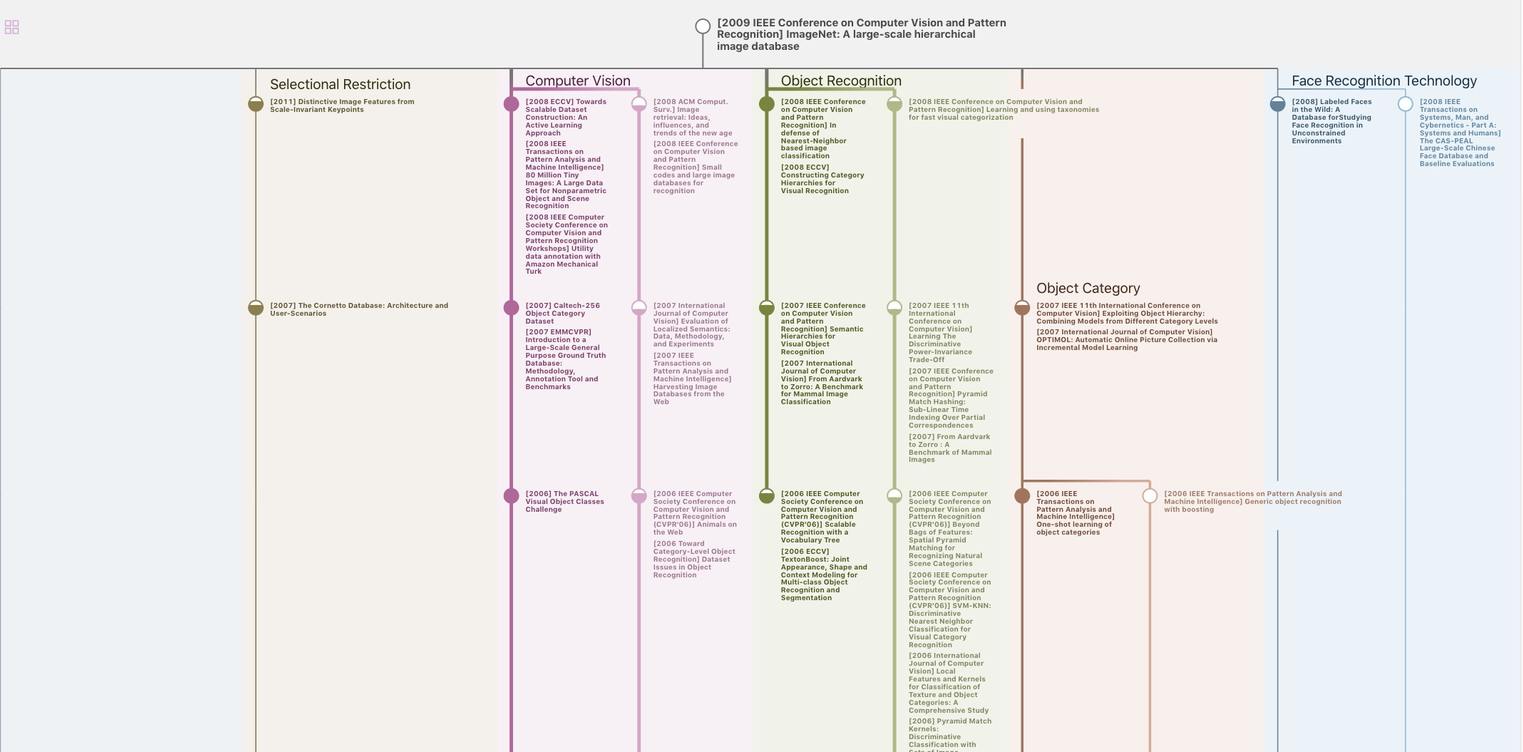
生成溯源树,研究论文发展脉络
Chat Paper
正在生成论文摘要