A Data-Driven Hybrid Automaton Framework to Modeling Complex Dynamical Systems
CoRR(2023)
摘要
In this paper, a computationally efficient data-driven hybrid automaton model is proposed to capture unknown complex dynamical system behaviors using multiple neural networks. The sampled data of the system is divided by valid partitions into groups corresponding to their topologies and based on which, transition guards are defined. Then, a collection of small-scale neural networks that are computationally efficient are trained as the local dynamical description for their corresponding topologies. After modeling the system with a neural-network-based hybrid automaton, the set-valued reachability analysis with low computation cost is provided based on interval analysis and a split and combined process. At last, a numerical example of the limit cycle is presented to illustrate that the developed models can significantly reduce the computational cost in reachable set computation without sacrificing any modeling precision.
更多查看译文
关键词
data-driven modeling,hybrid automata,neural networks
AI 理解论文
溯源树
样例
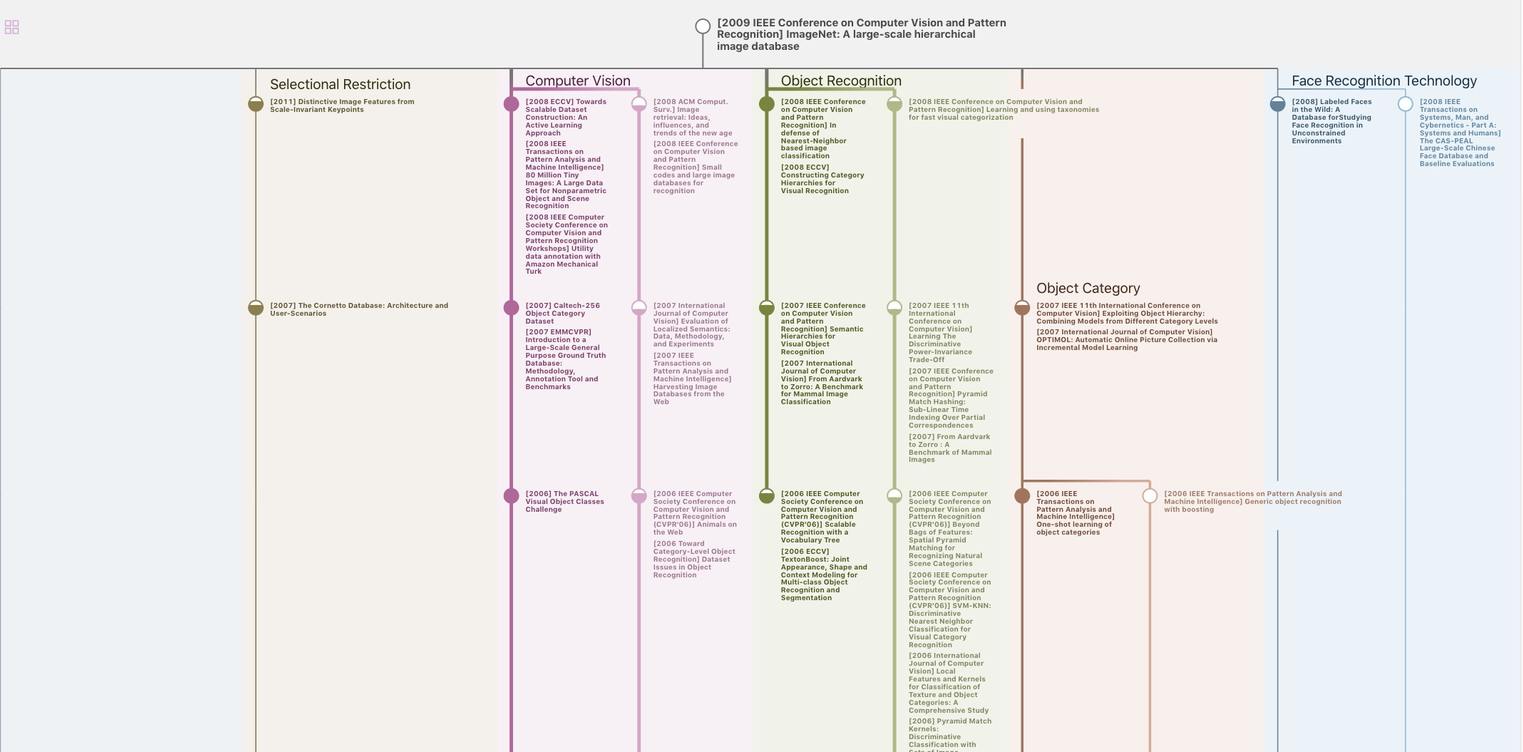
生成溯源树,研究论文发展脉络
Chat Paper
正在生成论文摘要