Algorithmic Vibe in Information Retrieval
WWW 2023(2023)
摘要
When information retrieval systems return a ranked list of results in response to a query, they may be choosing from a large set of candidate results that are equally useful and relevant. This means we might be able to identify a difference between rankers A and B, where ranker A systematically prefers a certain type of relevant results. Ranker A may have this systematic difference (different “vibe”) without having systematically better or worse results according to standard information retrieval metrics. We first show that a vibe difference can exist, comparing two publicly available rankers, where the one that is trained on health-related queries will systematically prefer health-related results, even for non-health queries. We define a vibe metric that lets us see the words that a ranker prefers. We investigate the vibe of search engine clicks vs. human labels. We perform an initial study into correcting for vibe differences to make ranker A more like ranker B via changes in negative sampling during training.
更多查看译文
AI 理解论文
溯源树
样例
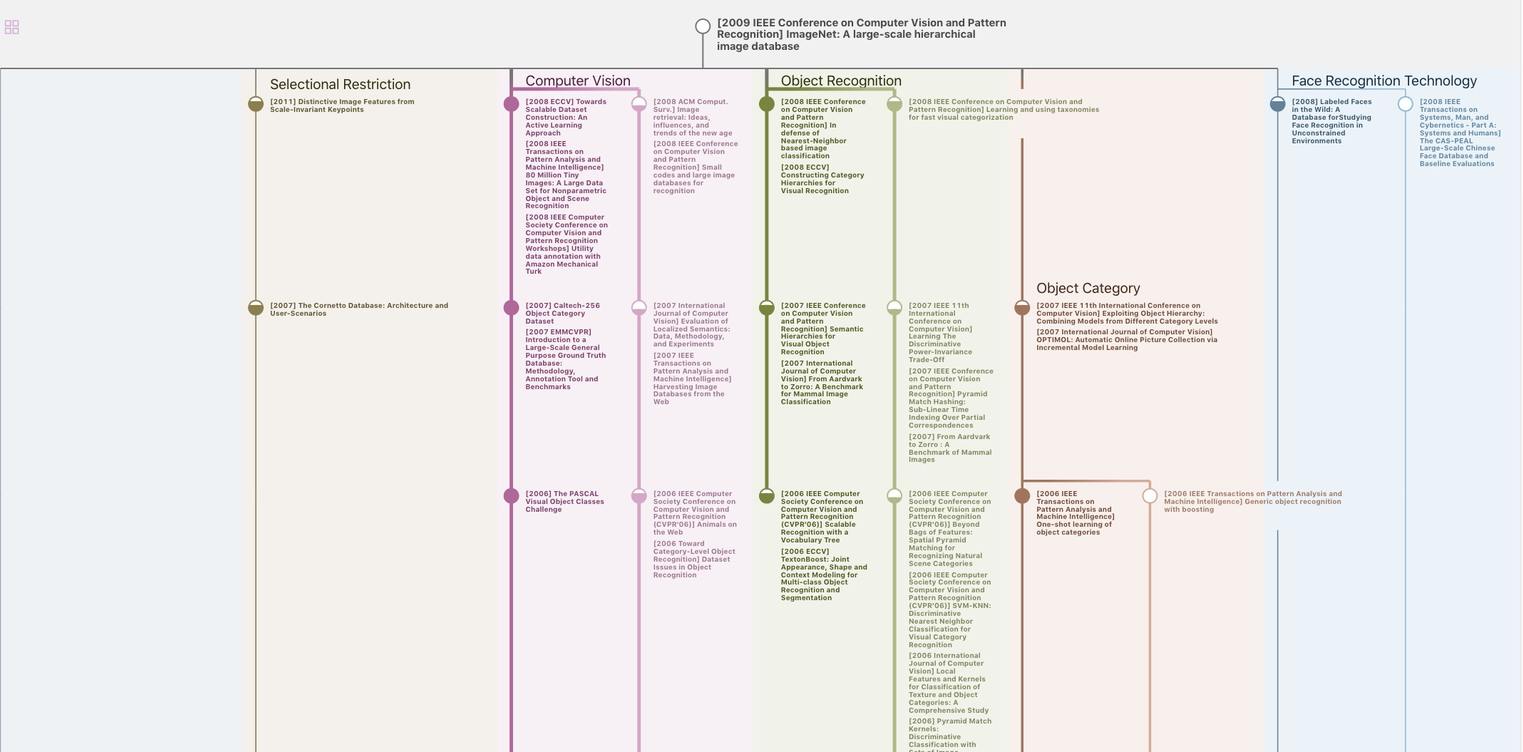
生成溯源树,研究论文发展脉络
Chat Paper
正在生成论文摘要