Match4Match: Enhancing Text-Video Retrieval by Maximum Flow with Minimum Cost
WWW 2023(2023)
摘要
With the explosive growth of video and text data on the web, text-video retrieval has become a vital task for online video platforms. Recently, text-video retrieval methods based on pre-trained models have attracted a lot of attention. However, existing methods cannot effectively capture the fine-grained information in videos, and typically suffer from the hubness problem where a collection of similar videos are retrieved by a large number of different queries. In this paper, we propose Match4Match, a new text-video retrieval method based on CLIP (Contrastive Language-Image Pretraining) and graph optimization theories. To balance calculation efficiency and model accuracy, Match4Match seamlessly supports three inference modes for different application scenarios. In fast vector retrieval mode, we embed texts and videos in the same space and employ a vector retrieval engine to obtain the top K videos. In fine-grained alignment mode, our method fully utilizes the pre-trained knowledge of the CLIP model to align words with corresponding video frames, and uses the fine-grained information to compute text-video similarity more accurately. In flow-style matching mode, to alleviate the detrimental impact of the hubness problem, we model the retrieval problem as a combinatorial optimization problem and solve it using maximum flow with minimum cost algorithm. To demonstrate the effectiveness of our method, we conduct experiments on five public text-video datasets. The overall performance of our proposed method outperforms state-of-the-art methods. Additionally, we evaluate the computational efficiency of Match4Match. Benefiting from the three flexible inference modes, Match4Match can respond to a large number of query requests with low latency or achieve high recall with acceptable time consumption.
更多查看译文
AI 理解论文
溯源树
样例
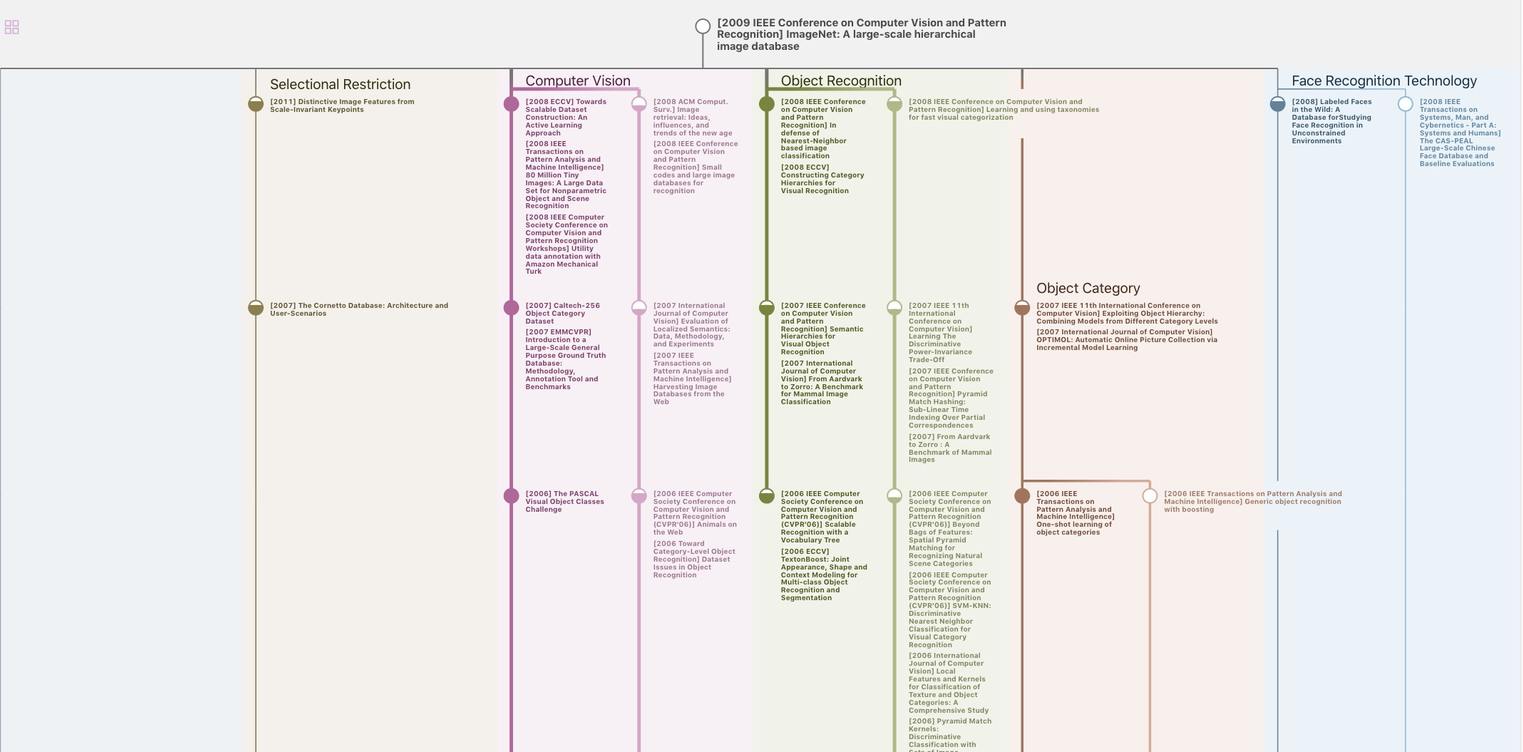
生成溯源树,研究论文发展脉络
Chat Paper
正在生成论文摘要