Transferring Audio Deepfake Detection Capability across Languages
WWW 2023(2023)
摘要
The proliferation of deepfake content has motivated a surge of detection studies. However, existing detection methods in the audio area exclusively work in English, and there is a lack of data resources in other languages. Cross-lingual deepfake detection, a critical but rarely explored area, urges more study. This paper conducts the first comprehensive study on the cross-lingual perspective of deepfake detection. We observe that English data enriched in deepfake algorithms can teach a detector the knowledge of various spoofing artifacts, contributing to performing detection across language domains. Based on the observation, we first construct a first-of-its-kind cross-lingual evaluation dataset including heterogeneous spoofed speech uttered in the two most widely spoken languages, then explored domain adaptation (DA) techniques to transfer the artifacts detection capability and propose effective and practical DA strategies fitting the cross-lingual scenario. Our adversarial-based DA paradigm teaches the model to learn real/fake knowledge while losing language dependency. Extensive experiments over 137-hour audio clips validate the adapted models can detect fake audio generated by unseen algorithms in the new domain.
更多查看译文
AI 理解论文
溯源树
样例
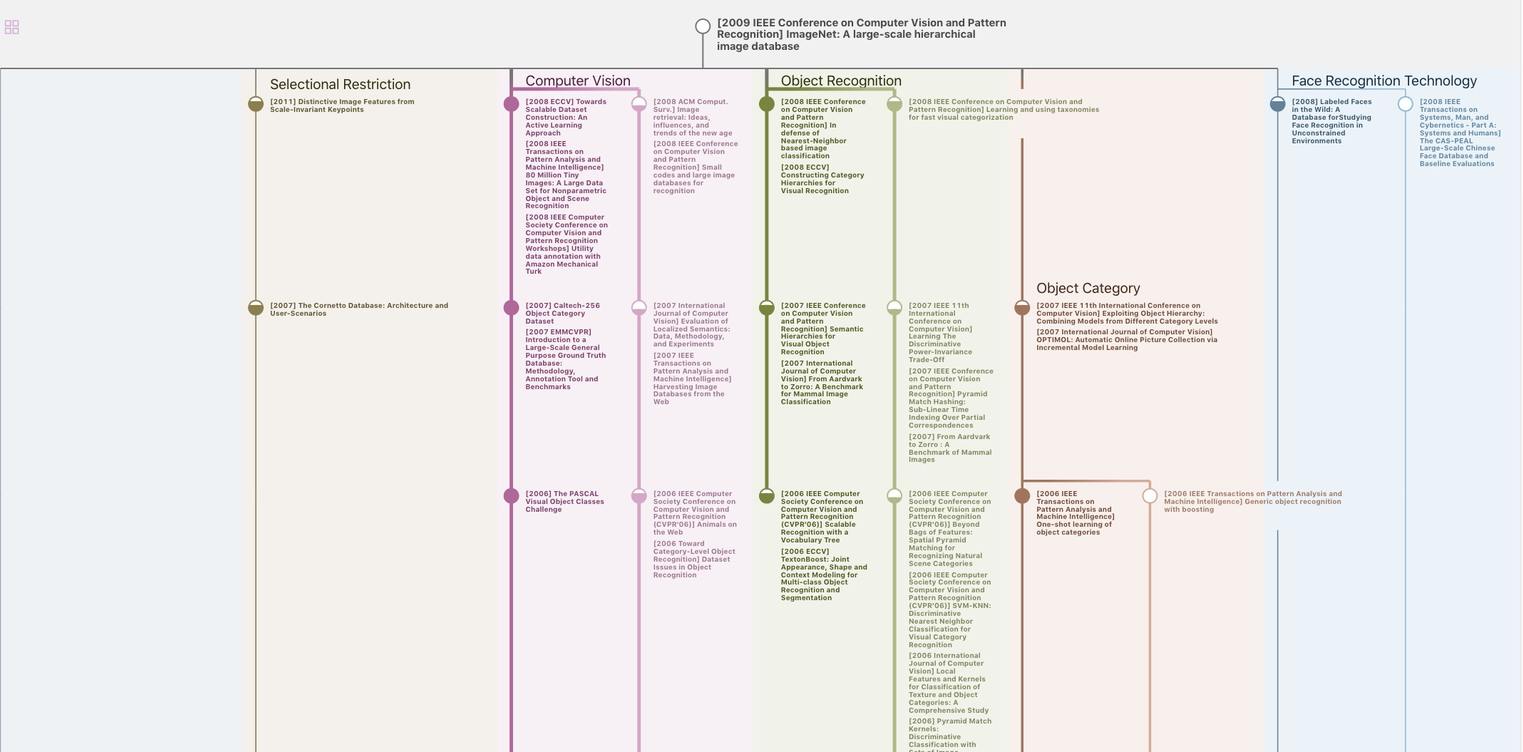
生成溯源树,研究论文发展脉络
Chat Paper
正在生成论文摘要