ColdNAS: Search to Modulate for User Cold-Start Recommendation: ColdNAS
WWW 2023(2023)
摘要
Making personalized recommendation for cold-start users, who only have a few interaction histories, is a challenging problem in recommendation systems. Recent works leverage hypernetworks to directly map user interaction histories to user-specific parameters, which are then used to modulate predictor by feature-wise linear modulation function. These works obtain the state-of-the-art performance. However, the physical meaning of scaling and shifting in recommendation data is unclear. Instead of using a fixed modulation function and deciding modulation position by expertise, we propose a modulation framework called ColdNAS for user cold-start problem, where we look for proper modulation structure, including function and position, via neural architecture search. We design a search space which covers broad models and theoretically prove that this search space can be transformed to a much smaller space, enabling an efficient and robust one-shot search algorithm. Extensive experimental results on benchmark datasets show that ColdNAS consistently performs the best. We observe that different modulation functions lead to the best performance on different datasets, which validates the necessity of designing a searching-based method. Codes are available at https://github.com/LARS-research/ColdNAS.
更多查看译文
关键词
search,cold-start
AI 理解论文
溯源树
样例
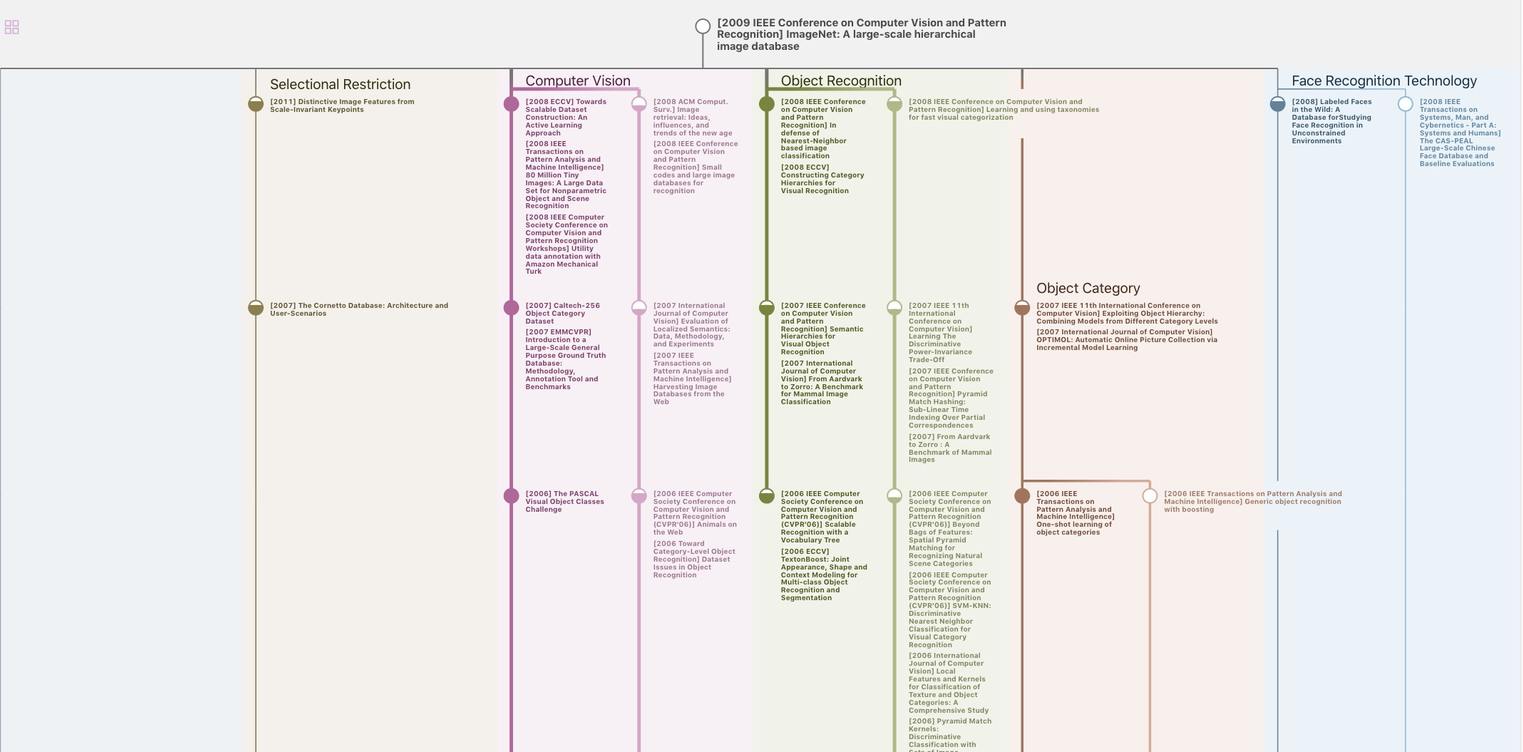
生成溯源树,研究论文发展脉络
Chat Paper
正在生成论文摘要