Unifying and Improving Graph Convolutional Neural Networks with Wavelet Denoising Filters
WWW 2023(2023)
摘要
Graph convolutional neural network (GCN) is a powerful deep learning framework for network data. However, variants of graph neural architectures can lead to drastically different performance on different tasks. Model comparison calls for a unifying framework with interpretability and principled experimental procedures. Based on the theories from graph signal processing (GSP), we show that GCN’s capability is fundamentally limited by the uncertainty principle, and wavelets provide a controllable trade-off between local and global information. We adapt wavelet denoising filters to the graph domain, unifying popular variants of GCN under a common interpretable mathematical framework. Furthermore, we propose WaveThresh and WaveShrink which are novel GCN models based on proven denoising filters from the signal processing literature. Empirically, we evaluate our models and other popular GCNs under a more principled procedure and analyze how trade-offs between local and global graph signals can lead to better performance in different datasets.
更多查看译文
AI 理解论文
溯源树
样例
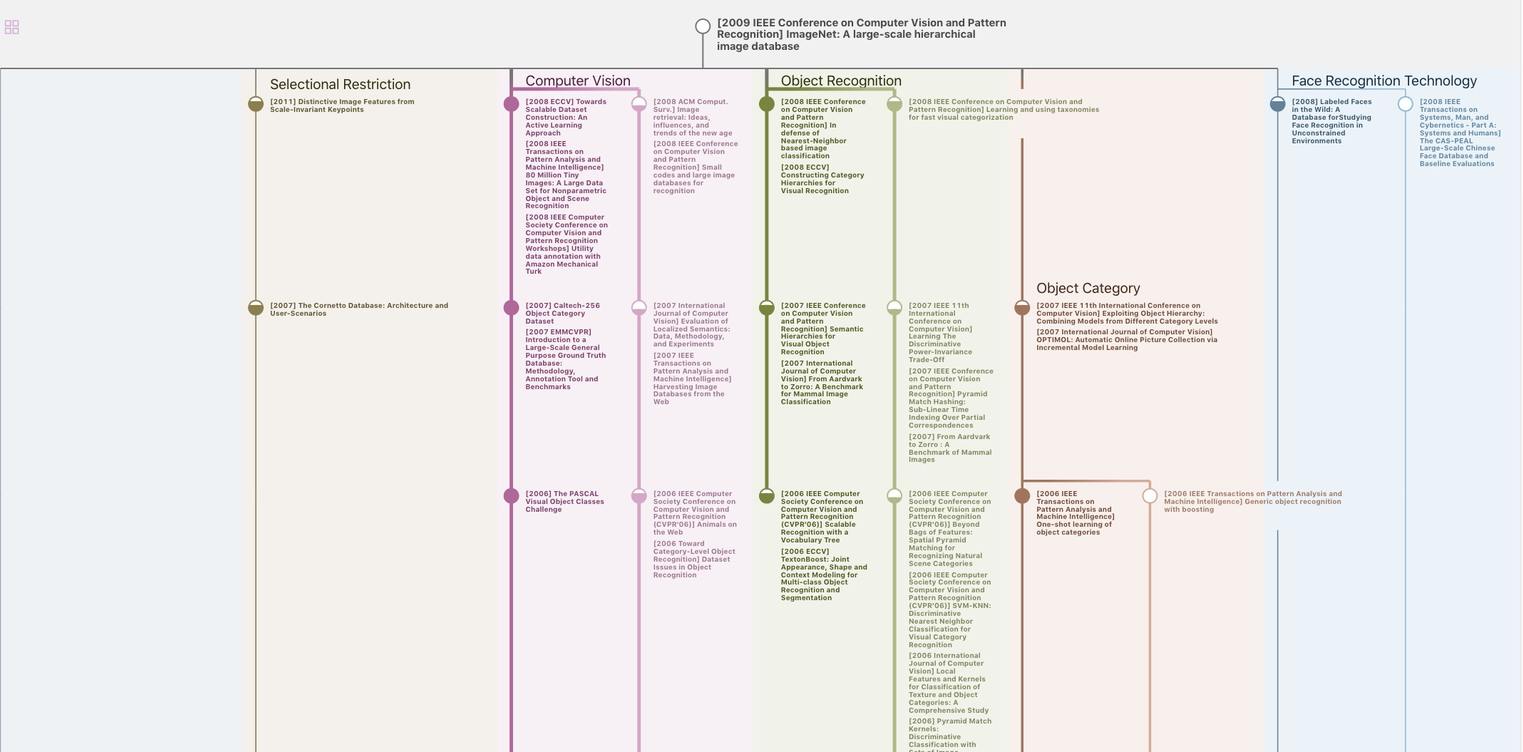
生成溯源树,研究论文发展脉络
Chat Paper
正在生成论文摘要