Enhancing Hierarchy-Aware Graph Networks with Deep Dual Clustering for Session-based Recommendation
WWW 2023(2023)
摘要
Session-based Recommendation aims at predicting the next interacted item based on short anonymous behavior sessions. However, existing solutions neglect to model two inherent properties of sequential representing distributions, i.e., hierarchy structures resulted from item popularity and collaborations existing in both intra- and inter-session. Tackling with these two factors at the same time is challenging. On the one hand, traditional Euclidean space utilized in previous studies fails to capture hierarchy structures due to a restricted representation ability. On the other hand, the intuitive apply of hyperbolic geometry could extract hierarchical patterns but more emphasis on degree distribution weakens intra- and inter-session collaborations. To address the challenges, we propose a Hierarchy-Aware Dual Clustering Graph Network (HADCG) model for session-based recommendation. Towards the first challenge, we design the hierarchy-aware graph modeling module which converts sessions into hyperbolic session graphs, adopting hyperbolic geometry in propagation and attention mechanism so as to integrate chronological and hierarchical information. As for the second challenge, we introduce the deep dual clustering module which develops a two-level clustering strategy, i.e., information regularizer for intra-session clustering and contrastive learner for inter-session clustering, to enhance hyperbolic representation learning from collaborative perspectives and further promote recommendation performance. Extensive experiments on three real-world datasets demonstrate the effectiveness of the proposed HADCG.
更多查看译文
AI 理解论文
溯源树
样例
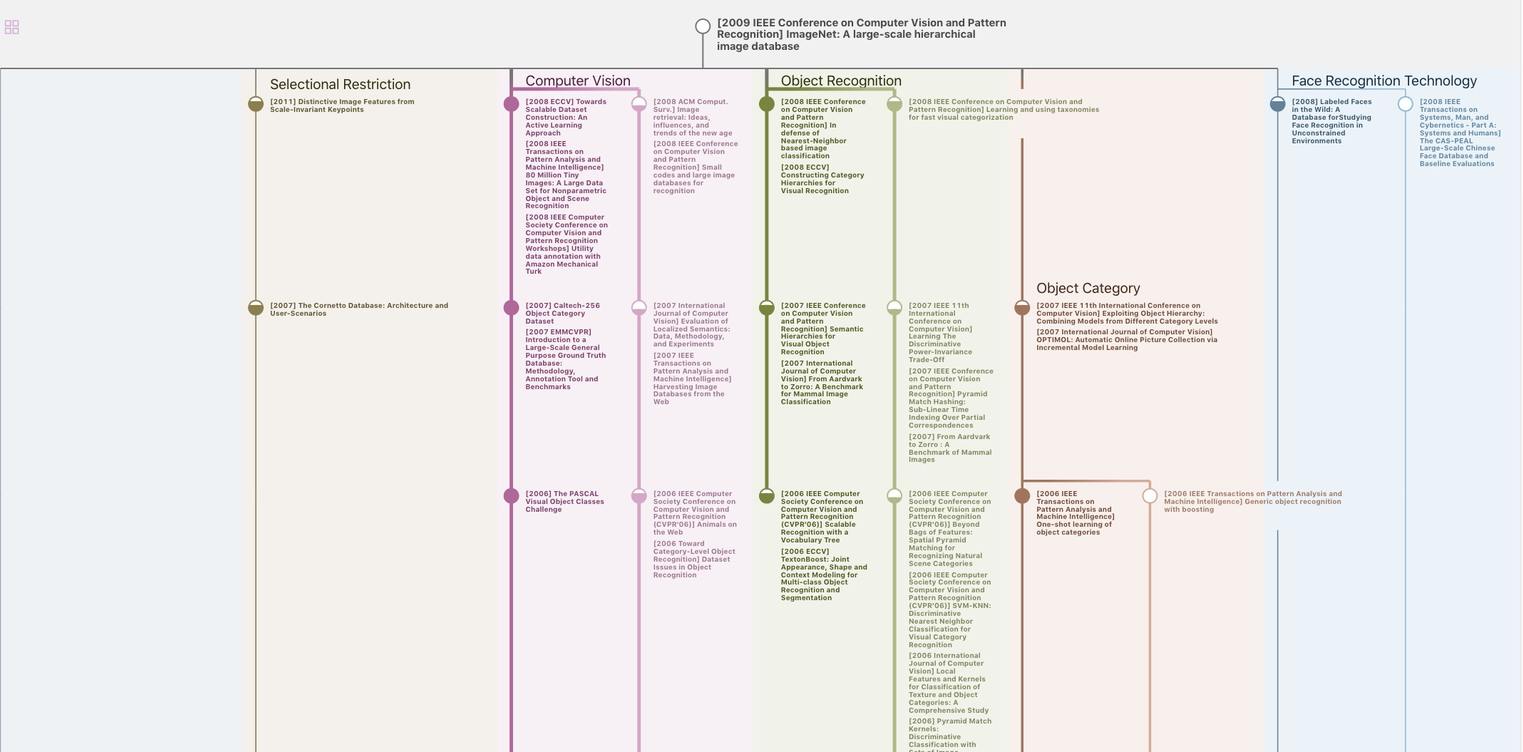
生成溯源树,研究论文发展脉络
Chat Paper
正在生成论文摘要