A probabilistic approach for acoustic emission based monitoring techniques: With application to structural health monitoring
MECHANICAL SYSTEMS AND SIGNAL PROCESSING(2024)
摘要
It has been demonstrated that acoustic-emission (AE), inspection of structures can offer advantages over other types of monitoring techniques in the detection of damage; namely, an increased sensitivity to damage, as well as an ability to localise its source. There are, however, numerous challenges associated with the analysis of AE data. One issue is the high sampling frequencies required to capture AE activity. In just a few seconds, a recording can generate very high volumes of data, of which a significant portion may be of little interest for analysis. Identifying the individual AE events in a recorded time-series is therefore a necessary procedure for reducing the size of the dataset and projecting out the influence of background noise from the signal. In this paper, a state-of-the-art technique is presented that can automatically identify cluster the AE events from a probabilistic perspective. A nonparametric Bayesian approach, based on the Dirichlet process (DP), is employed to overcome some of the challenges associated with this task. Additionally, the developed model is applied for damage detection using AE data collected from an experimental setup. Two main sets of AE data are considered in this work: (1) from a journal bearing in operation, and (2) from an Airbus A320 main landing gear subjected to fatigue testing.
更多查看译文
关键词
Structural health monitoring,Acoustic emission,Damage detection,Bayesian methods,Fracture identification,Dirichlet process
AI 理解论文
溯源树
样例
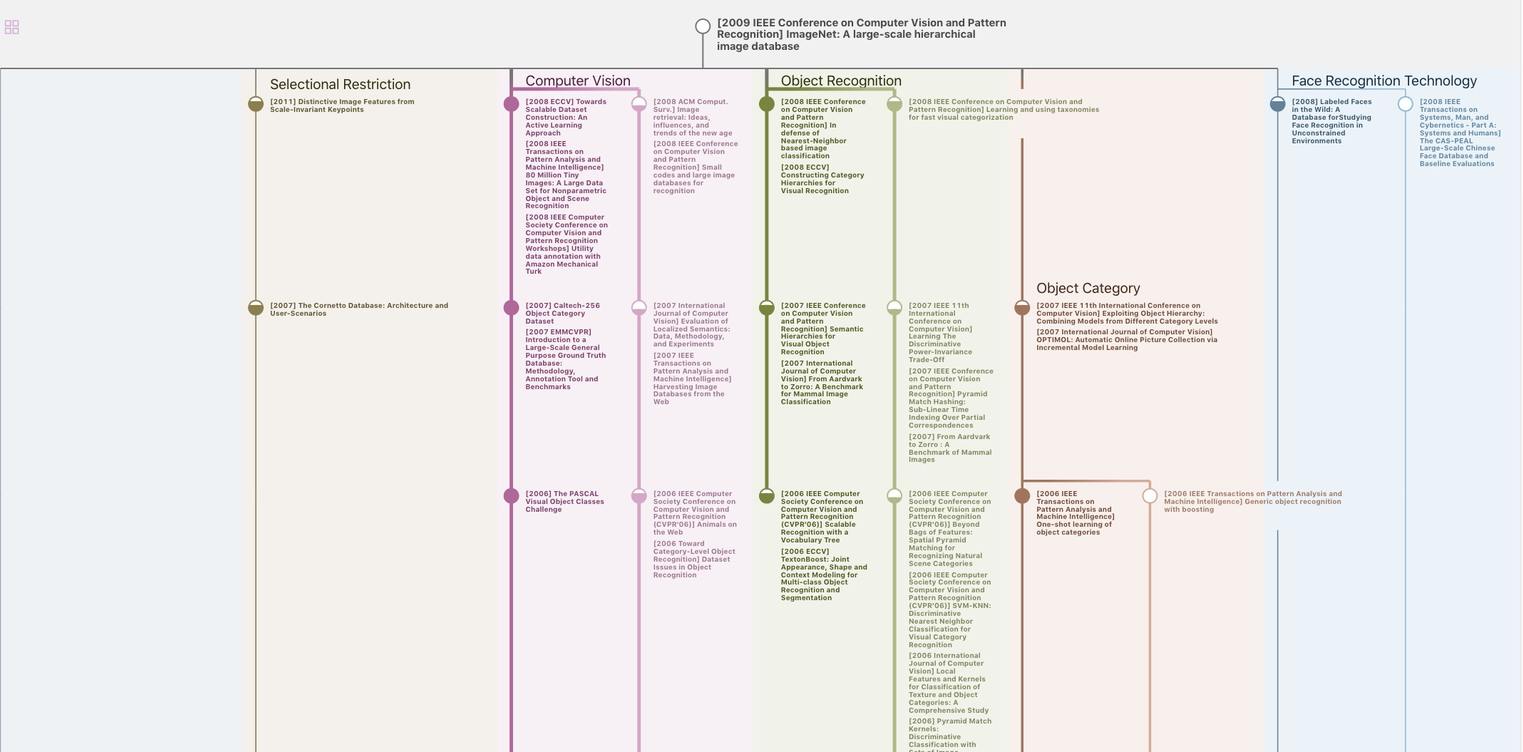
生成溯源树,研究论文发展脉络
Chat Paper
正在生成论文摘要