Machine learning method for 12C event classification and reconstruction in the active target time-projection chamber
NUCLEAR INSTRUMENTS & METHODS IN PHYSICS RESEARCH SECTION A-ACCELERATORS SPECTROMETERS DETECTORS AND ASSOCIATED EQUIPMENT(2023)
摘要
Active target time projection chambers are important tools in low energy radioactive ion beams or gamma rays related researches. In this work, we present the application of machine learning methods to the analysis of data obtained from an active target time projection chamber. Specifically, we investigate the effectiveness of Visual Geometry Group (VGG) and the Residual neural Network (ResNet) models for event classification and reconstruction in decays from the excited 2(2)(+) state in C-12 Hoyle rotation band. The results show that machine learning methods are effective in identifying C-12 events from the background noise, with ResNet-34 achieving an impressive precision of 0.99 on simulation data, and the best performing event reconstruction model ResNet-18 providing an energy resolution of sigma(E) < 77 keV and an angular reconstruction deviation of sigma(theta) < 0.1 rad. The promising results suggest that the ResNet model trained on Monte Carlo samples could be used for future classifying and predicting experimental data in active target time projection chambers related experiments.
更多查看译文
关键词
Machine learning,Convolutional neural network,Active targets,Time projection chamber,Hoyle rotation band
AI 理解论文
溯源树
样例
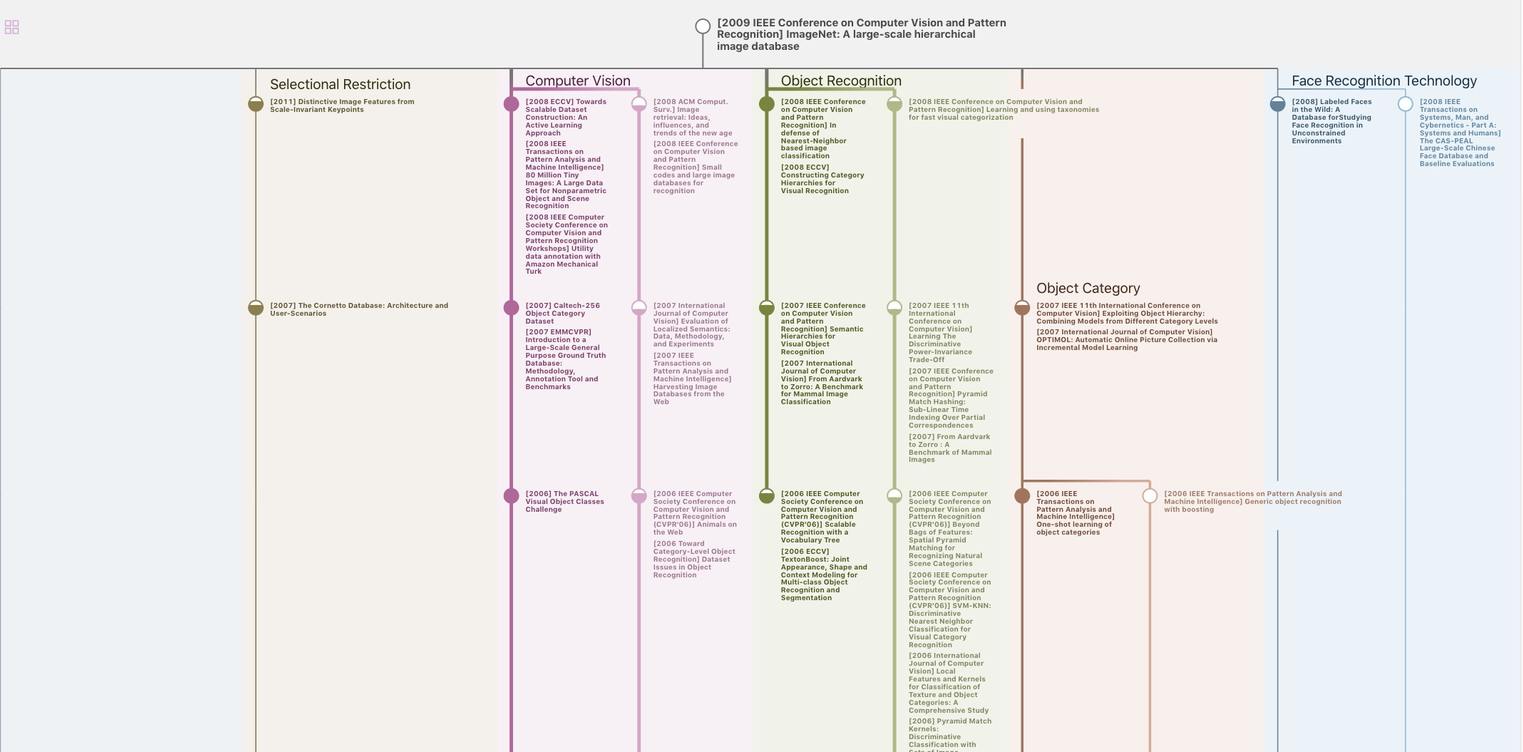
生成溯源树,研究论文发展脉络
Chat Paper
正在生成论文摘要