Estimation of Characteristics-based Quantile Factor Models
arXiv (Cornell University)(2023)
摘要
This paper studies the estimation of characteristic-based quantile factor models where the factor loadings are unknown functions of observed individual characteristics while the idiosyncratic error terms are subject to conditional quantile restrictions. We propose a three-stage estimation procedure that is easily implementable in practice and has nice properties. The convergence rates, the limiting distributions of the estimated factors and loading functions, and a consistent selection criterion for the number of factors at each quantile are derived under general conditions. The proposed estimation methodology is shown to work satisfactorily when: (i) the idiosyncratic errors have heavy tails, (ii) the time dimension of the panel dataset is not large, and (iii) the number of factors exceeds the number of characteristics. Finite sample simulations and an empirical application aimed at estimating the loading functions of the daily returns of a large panel of S\&P500 index securities help illustrate these properties.
更多查看译文
关键词
quantile factor models,estimation,characteristics-based
AI 理解论文
溯源树
样例
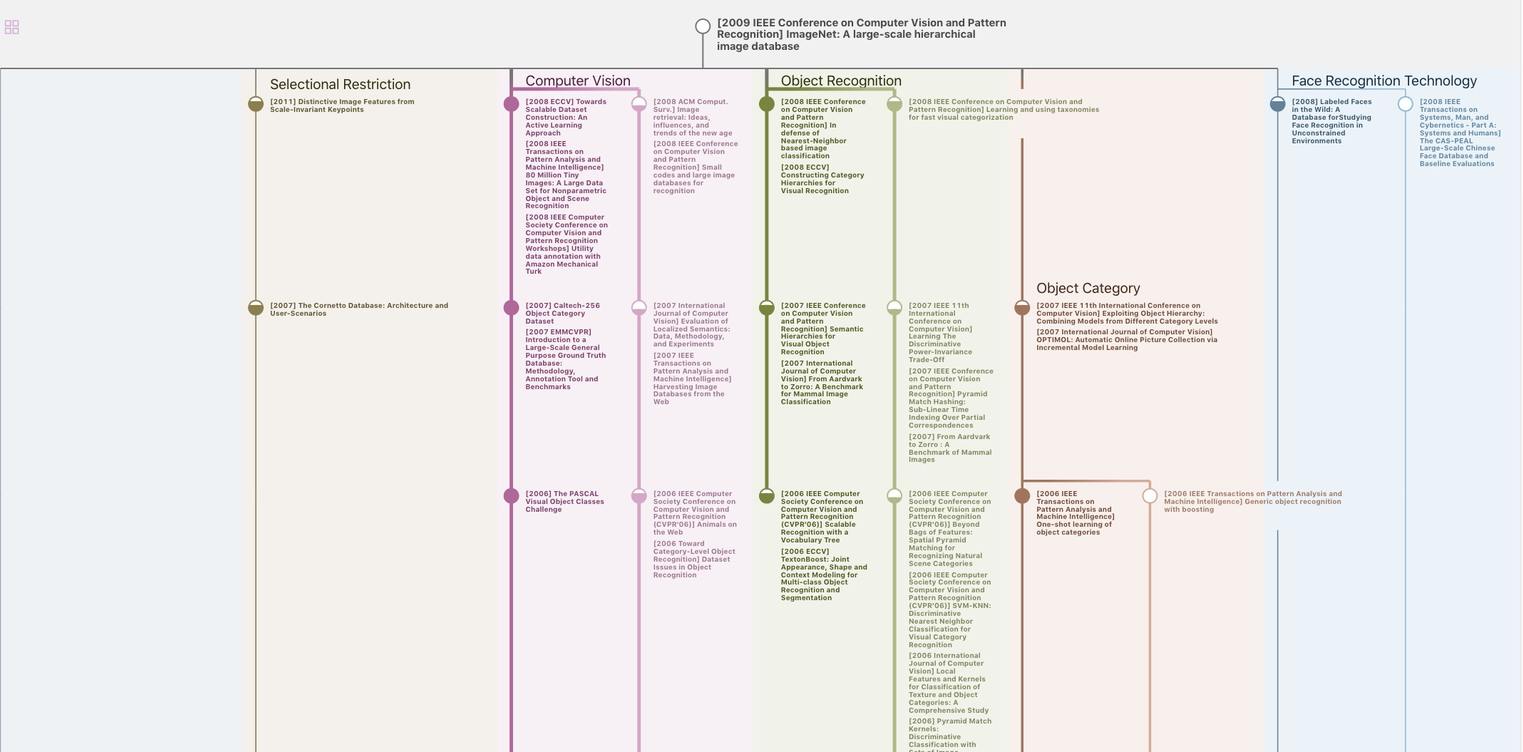
生成溯源树,研究论文发展脉络
Chat Paper
正在生成论文摘要