On the redundancy in large material datasets: efficient and robust learning with less data
Zenodo (CERN European Organization for Nuclear Research)(2023)
摘要
Extensive efforts to gather materials data have largely overlooked potential data redundancy. In this study, we present evidence of a significant degree of redundancy across multiple large datasets for various material properties, by revealing that up to 95 % of data can be safely removed from machine learning training with little impact on in-distribution prediction performance. The redundant data is related to over-represented material types and does not mitigate the severe performance degradation on out-of-distribution samples. In addition, we show that uncertainty-based active learning algorithms can construct much smaller but equally informative datasets. We discuss the effectiveness of informative data in improving prediction performance and robustness and provide insights into efficient data acquisition and machine learning training. This work challenges the "bigger is better" mentality and calls for attention to the information richness of materials data rather than a narrow emphasis on data volume.
更多查看译文
关键词
large material datasets,robust learning,less data,redundancy
AI 理解论文
溯源树
样例
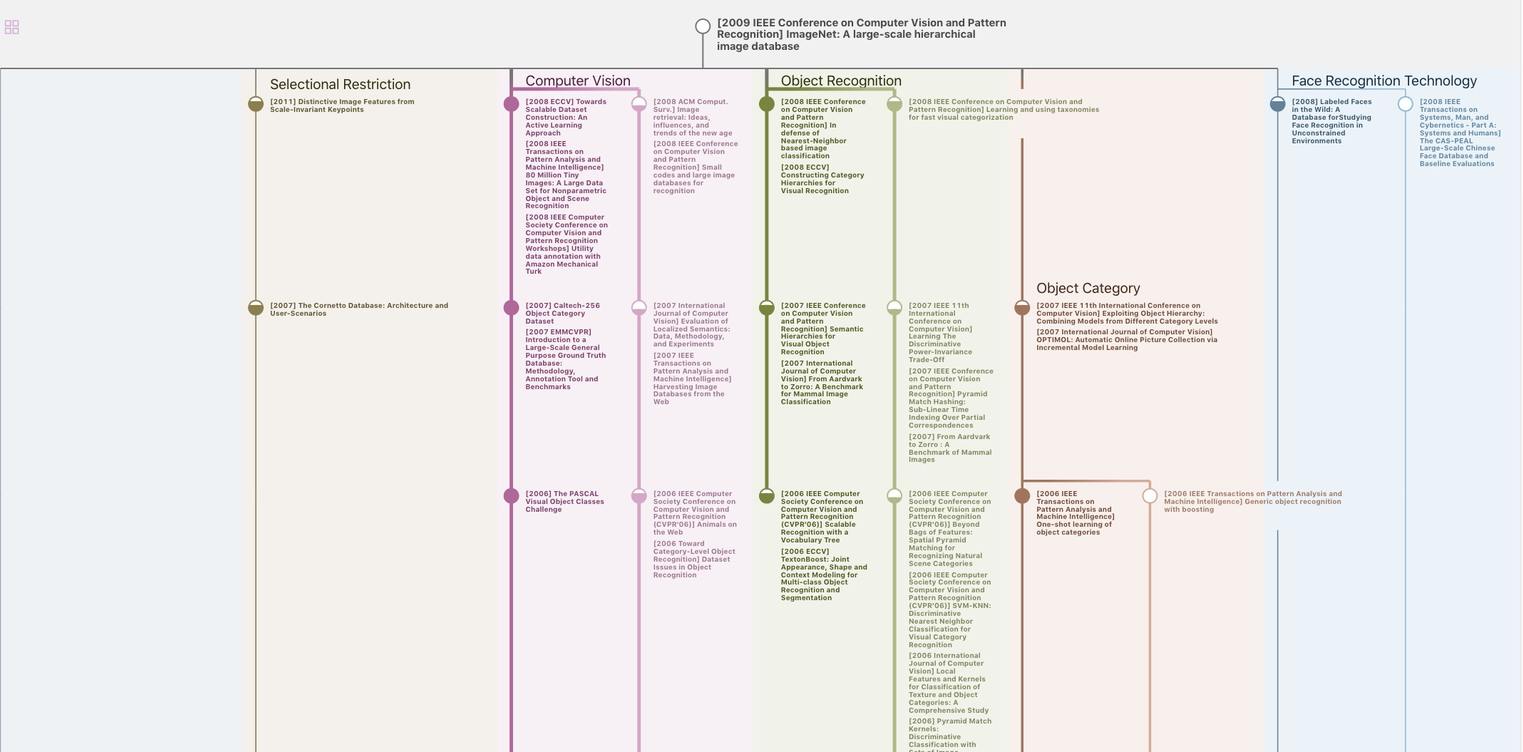
生成溯源树,研究论文发展脉络
Chat Paper
正在生成论文摘要