High performance clean versus artifact dry electrode EEG data classification using Convolutional Neural Network transfer learning
Clinical Neurophysiology Practice(2023)
摘要
Objective: Convolutional Neural Networks (CNNs) are promising for artifact detection in electroencephalography (EEG) data, but require large amounts of data. Despite increasing use of dry electrodes for EEG data acquisition, dry electrode EEG datasets are sparse. We aim to develop an algorithm for clean versus artifact dry electrode EEG data classification using transfer learning. Methods: Dry electrode EEG data were acquired in 13 subjects while physiological and technical artifacts were induced. Data were per 2-second segment labeled as clean or artifact and split in an 80% train and 20% test set. With the train set, we fine-tuned a pre-trained CNN for clean versus artifact wet electrode EEG data classification using 3-fold cross validation. The three fine-tuned CNNs were combined in one final clean versus artifact classification algorithm, in which the majority vote was used for classification. We calculated accuracy, F1-score, precision, and recall of the pre-trained CNN and fine-tuned algorithm when applied to unseen test data. Results: The algorithm was trained on 0.40 million and tested on 0.17 million overlapping EEG segments. The pre-trained CNN had a test accuracy of 65.6%. The fine-tuned clean versus artifact classification algorithm had an improved test accuracy of 90.7%, F1-score of 90.2%, precision of 89.1% and recall of 91.2%. Conclusions: Despite a relatively small dry electrode EEG dataset, transfer learning enabled development of a high performing CNN-based algorithm for clean versus artifact classification. Significance: Development of CNNs for classification of dry electrode EEG data is challenging as dry electrode EEG datasets are sparse. Here, we show that transfer learning can be used to overcome this problem.
更多查看译文
关键词
Electroencephalography,Dry electrodes,Artifact,Convolutional neural network,Transfer learning
AI 理解论文
溯源树
样例
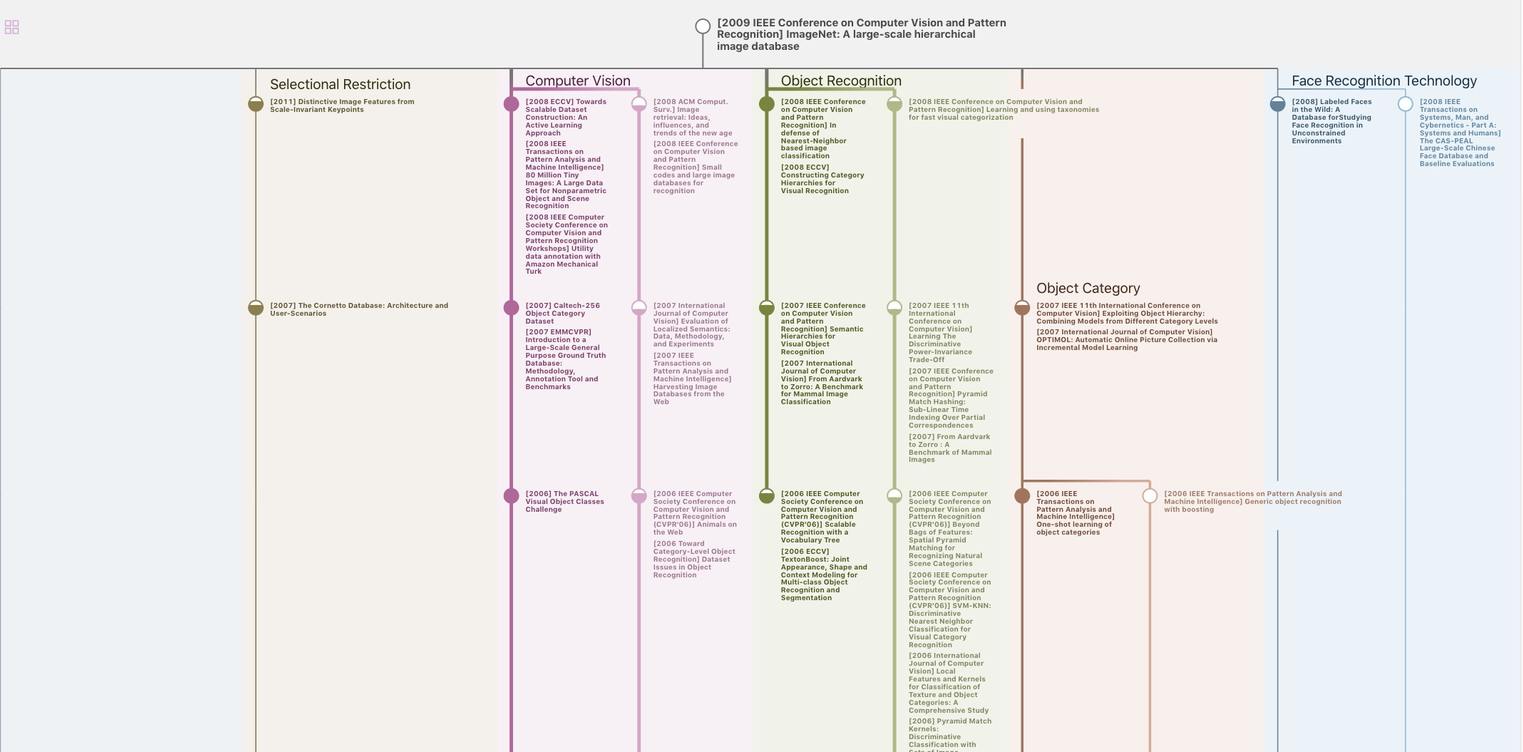
生成溯源树,研究论文发展脉络
Chat Paper
正在生成论文摘要