Generating Robust Counterfactual Explanations
MACHINE LEARNING AND KNOWLEDGE DISCOVERY IN DATABASES: RESEARCH TRACK, ECML PKDD 2023, PT III(2023)
摘要
Counterfactual explanations have become a mainstay of the XAI field. This particularly intuitive statement allows the user to understand what small but necessary changes would have to be made to a given situation in order to change a model prediction. The quality of a counterfactual depends on several criteria: realism, actionability, validity, robustness, etc. In this paper, we are interested in the notion of robustness of a counterfactual. More precisely, we focus on robustness to counterfactual input changes. This form of robustness is particularly challenging as it involves a trade-off between the robustness of the counterfactual and the proximity with the example to explain. We propose a new framework, CROCO, that generates robust counterfactuals while managing effectively this trade-off, and guarantees the user a minimal robustness. An empirical evaluation on tabular datasets confirms the relevance and effectiveness of our approach.
更多查看译文
关键词
Counterfactual explanation,Robustness,Algorithmic recourse
AI 理解论文
溯源树
样例
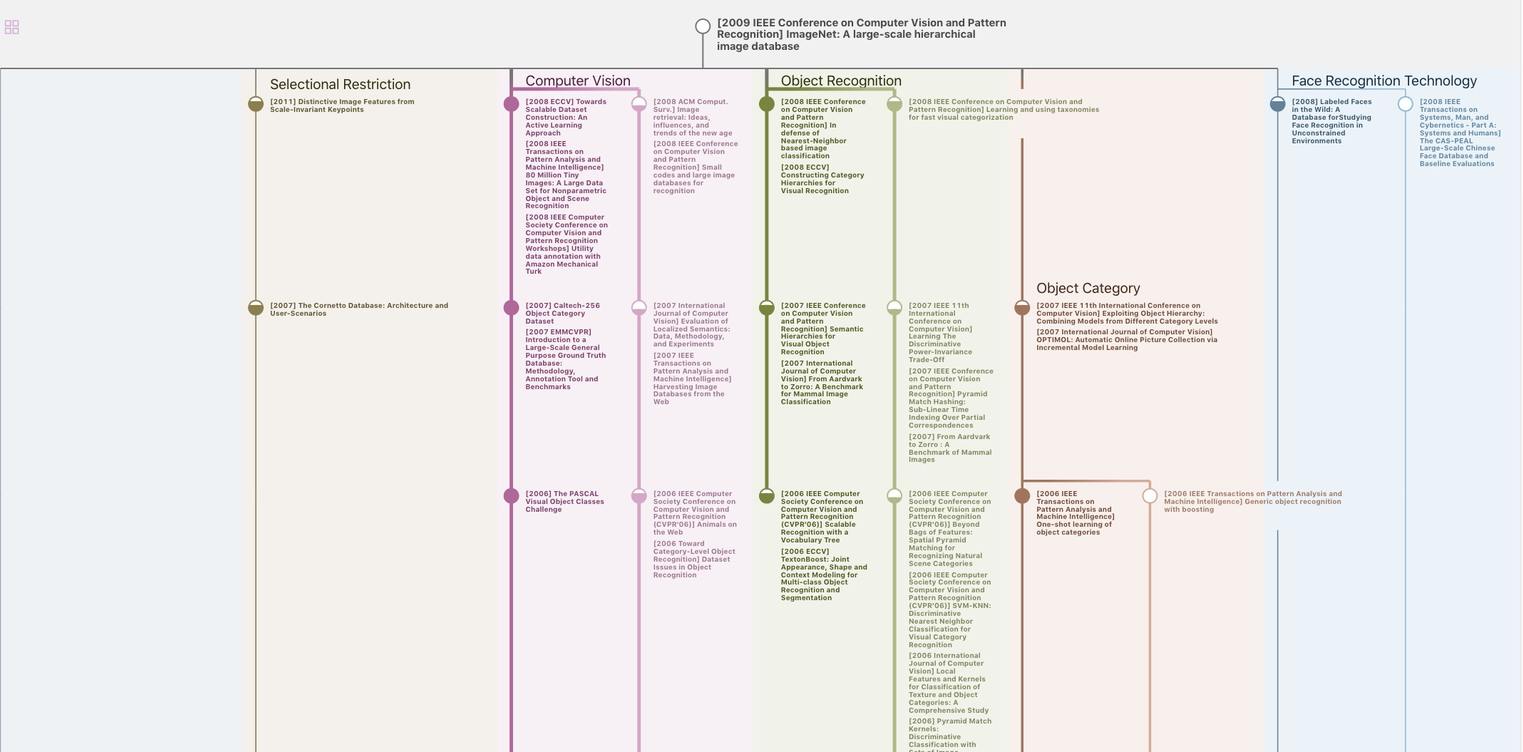
生成溯源树,研究论文发展脉络
Chat Paper
正在生成论文摘要