Limiting eigenvalue distribution of heavy-tailed Toeplitz matrices
arxiv(2023)
摘要
We consider an $N \times N$ random symmetric Toeplitz matrix with an i.i.d. input sequence drawn from a distribution that lies in the domain of attraction of an $\alpha$-stable law for $0 < \alpha < 2$. We show that under an appropriate scaling, its empirical eigenvalue distribution, as $N \to \infty$, converges weakly to a random symmetric probability distribution on $\mathbb{R}$, which can be described as the expected spectral measure of a certain random unbounded self-adjoint operator on $\ell^2(\mathbb{Z})$. The limiting distribution turns out to be almost surely subgaussian. Furthermore, the support of the limiting distribution is bounded almost surely if $0<\alpha <1$ and is unbounded almost surely if $1\leq \alpha <2$.
更多查看译文
关键词
eigenvalue distribution,heavy-tailed
AI 理解论文
溯源树
样例
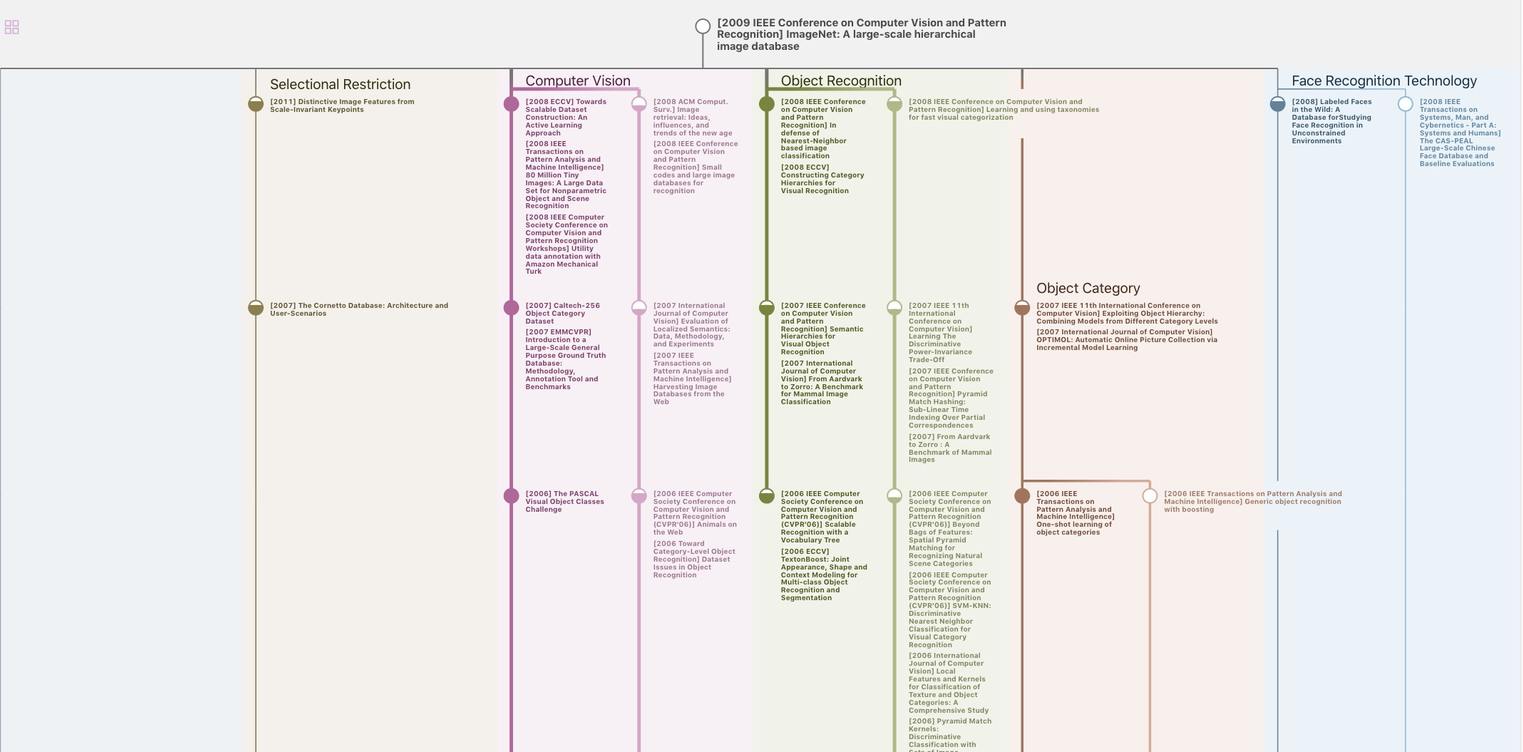
生成溯源树,研究论文发展脉络
Chat Paper
正在生成论文摘要