CIMLA: Interpretable AI for inference of differential causal networks
CoRR(2023)
摘要
The discovery of causal relationships from high-dimensional data is a major open problem in bioinformatics. Machine learning and feature attribution models have shown great promise in this context but lack causal interpretation. Here, we show that a popular feature attribution model estimates a causal quantity reflecting the influence of one variable on another, under certain assumptions. We leverage this insight to implement a new tool, CIMLA, for discovering condition-dependent changes in causal relationships. We then use CIMLA to identify differences in gene regulatory networks between biological conditions, a problem that has received great attention in recent years. Using extensive benchmarking on simulated data sets, we show that CIMLA is more robust to confounding variables and is more accurate than leading methods. Finally, we employ CIMLA to analyze a previously published single-cell RNA-seq data set collected from subjects with and without Alzheimer's disease (AD), discovering several potential regulators of AD.
更多查看译文
关键词
differential causal networks,interpretable ai,inference
AI 理解论文
溯源树
样例
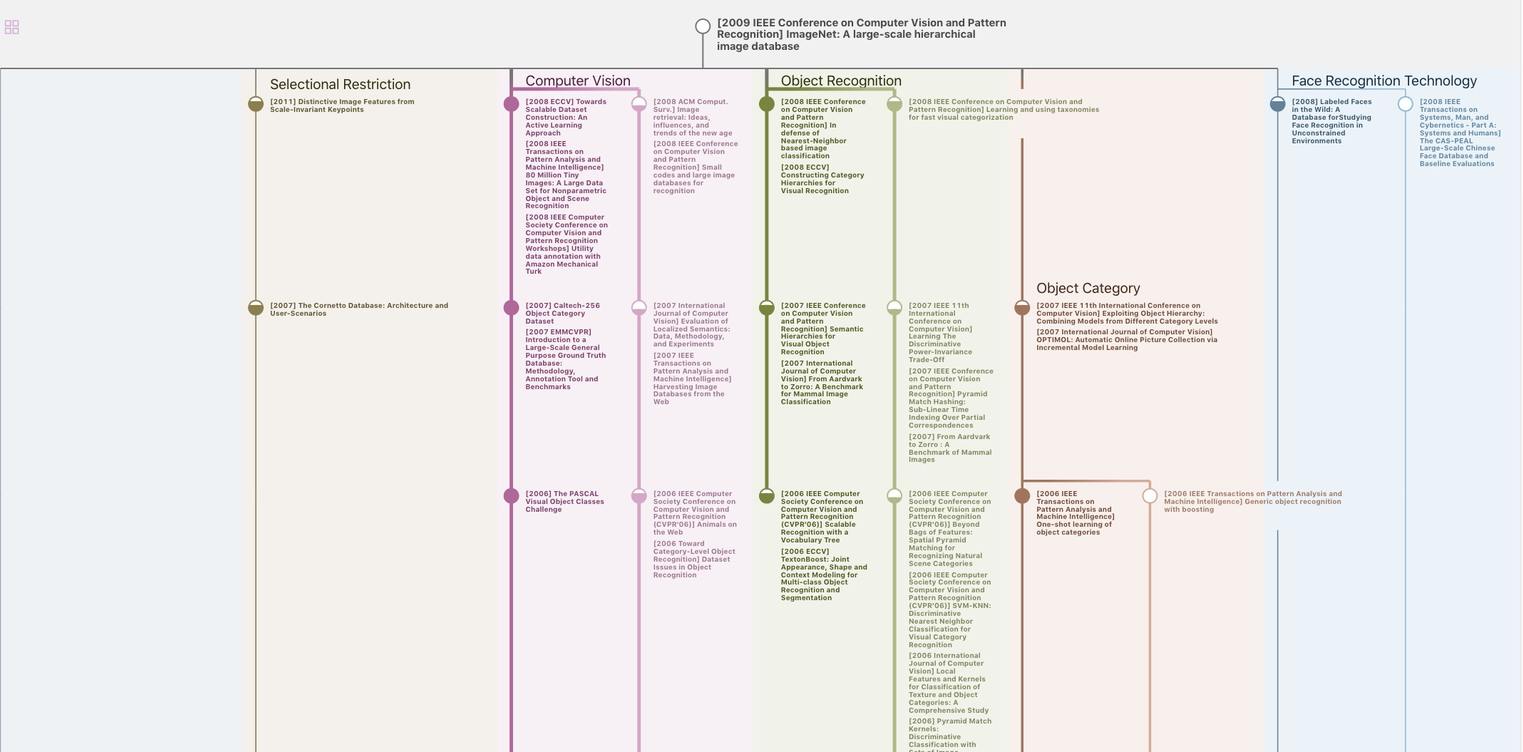
生成溯源树,研究论文发展脉络
Chat Paper
正在生成论文摘要